A Personalized Learning Scheme for Internet of Vehicles Caching
2021 IEEE GLOBAL COMMUNICATIONS CONFERENCE (GLOBECOM)(2021)
摘要
The emergence of Internet of Vehicles (IoV), as a large-scale distributed system, introduces new research and development challenges for supporting resource-constrained devices. In fact, the latency of retrieving contents and performing the desired tasks may increase dramatically and failures may occur when resource limits are exceeded. In this paper, we assess the use of advanced machine learning paradigms to achieve more accurate personalized edge caching and replacement decisions, while supporting data privacy, vehicle mobility, time-varying and location-aware content popularity. Firstly, we show that popular federated learning-based schemes fail in maintaining acceptable performance under the above settings. Secondly, we propose a scalable-by-design edge caching scheme for IoV, leveraging decentralized region-to-region Road Side Units (RSUs) exchanges while enhancing region local models. Finally, simulation results show that our scheme achieves higher performance in terms of average delay and edge hit ratio while keeping the cost and privacy risks at a minimum.
更多查看译文
关键词
accurate personalized edge caching,replacement decisions,data privacy,vehicle mobility,time-varying,location-aware content popularity,popular federated learning-based schemes,scalable-by-design edge caching scheme,IoV,decentralized region-to-region Road Side Units,region local models,average delay,personalized learning scheme,Vehicles caching,large-scale distributed system,research,development challenges,resource-constrained devices,desired tasks,resource limits,advanced machine
AI 理解论文
溯源树
样例
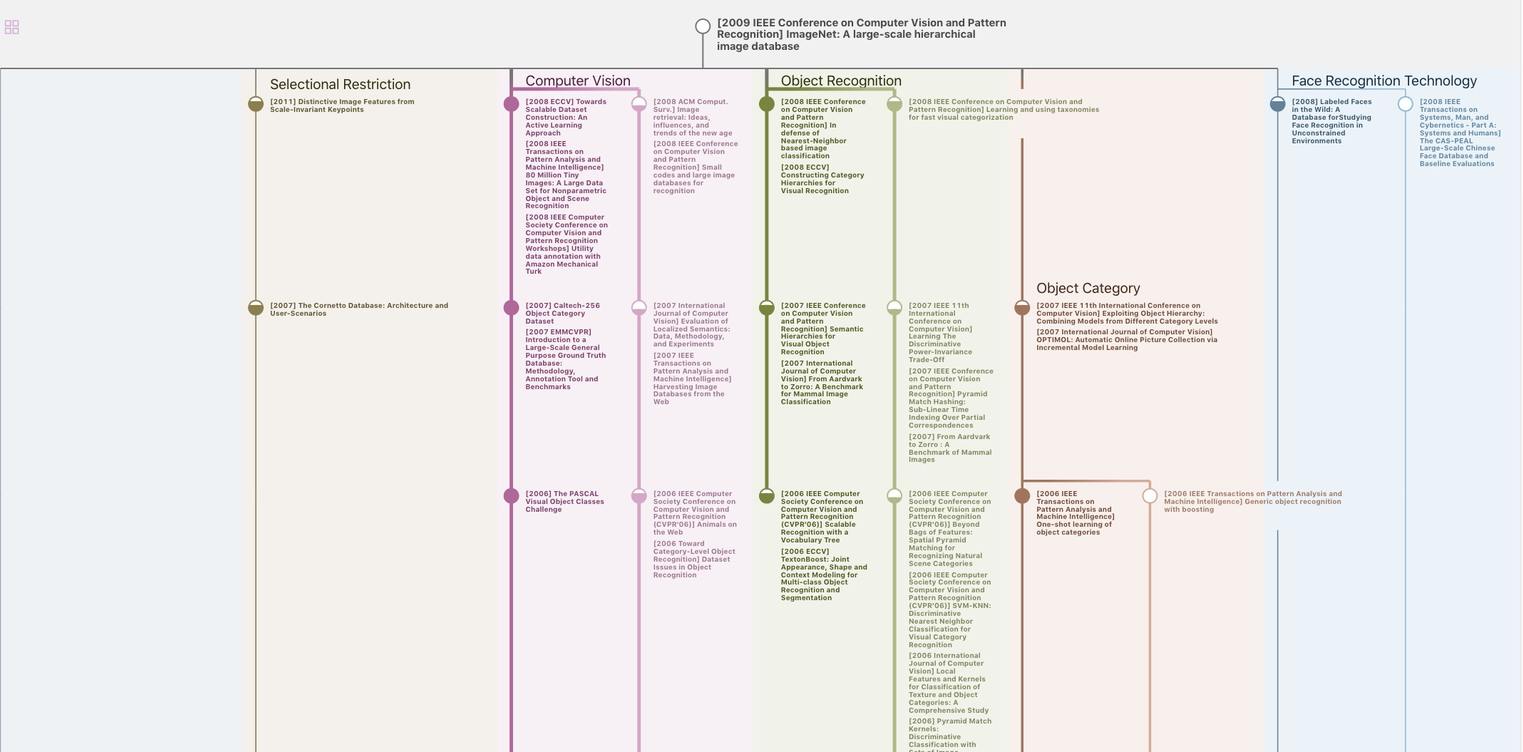
生成溯源树,研究论文发展脉络
Chat Paper
正在生成论文摘要