Deep Reinforcement Learning for cell on/off energy saving on Wireless Networks
2021 IEEE GLOBAL COMMUNICATIONS CONFERENCE (GLOBECOM)(2021)
摘要
Increased network traffic demands have led to extremely dense network deployments. This translates to significant growth in energy consumption at the radio access networks, resulting in high network operation costs (OPEX). In this work, we apply deep reinforcement learning to reduce the energy consumption at the base station in dense wireless networks, by allowing cells that overlap in geographical areas to be put in standby mode according to the changing network conditions. We start by formulating the problem of the cell on/off energy saving in dense wireless networks as a Markov decision process. Then, a deep reinforcement learning (DRL) solution is proposed. This DRL solution takes into account different key performance indicators (KPIs) of both the network and user equipment and aims to reduce the energy consumed by the network without significantly impacting the overall KPIs. The performance of the proposed solution is evaluated using a practical network simulator.
更多查看译文
关键词
Reinforcement learning, Energy Saving, Cell on/off, Deep Neural Networks
AI 理解论文
溯源树
样例
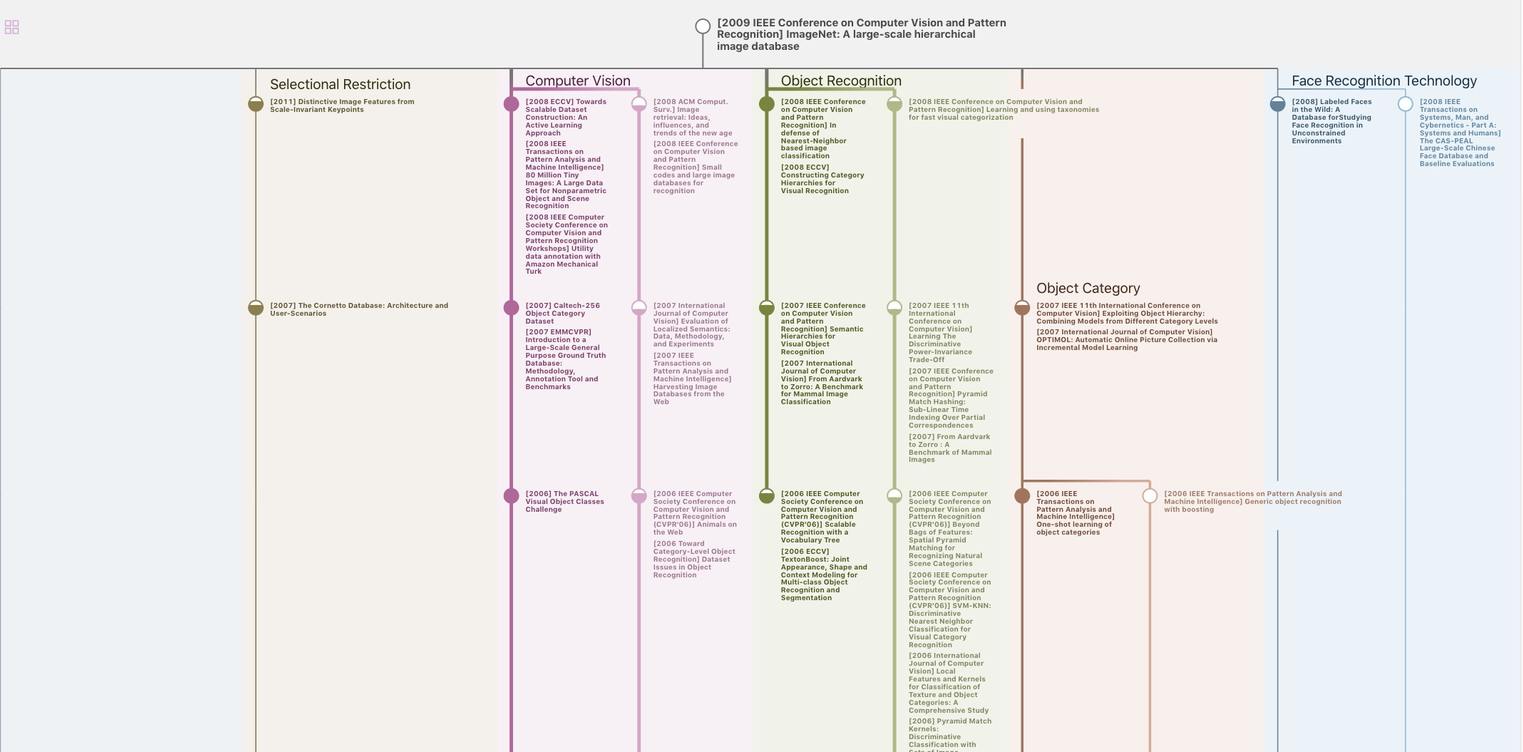
生成溯源树,研究论文发展脉络
Chat Paper
正在生成论文摘要