Load Balancing for Communication Networks via Data-Efficient Deep Reinforcement Learning
2021 IEEE GLOBAL COMMUNICATIONS CONFERENCE (GLOBECOM)(2021)
摘要
Within a cellular network, load balancing between different cells is of critical importance to network performance and quality of service. Most existing load balancing algorithms are manually designed and tuned rule-based methods where near-optimality is almost impossible to achieve. These rule-based meth-ods are difficult to adapt quickly to traffic changes in real-world environments. Given the success of Reinforcement Learning (RL) algorithms in many application domains, there have been a number of efforts to tackle load balancing for communication systems using RL-based methods. To our knowledge, none of these efforts have addressed the need for data efficiency within the RL framework, which is one of the main obstacles in applying RL to wireless network load balancing. In this paper, we formulate the communication load balancing problem as a Markov Decision Process and propose a data-efficient transfer deep reinforcement learning algorithm to address it. Experimental results show that the proposed method can significantly improve the system performance over other baselines and is more robust to environmental changes.
更多查看译文
关键词
load balancing, reinforcement learning, transfer learning
AI 理解论文
溯源树
样例
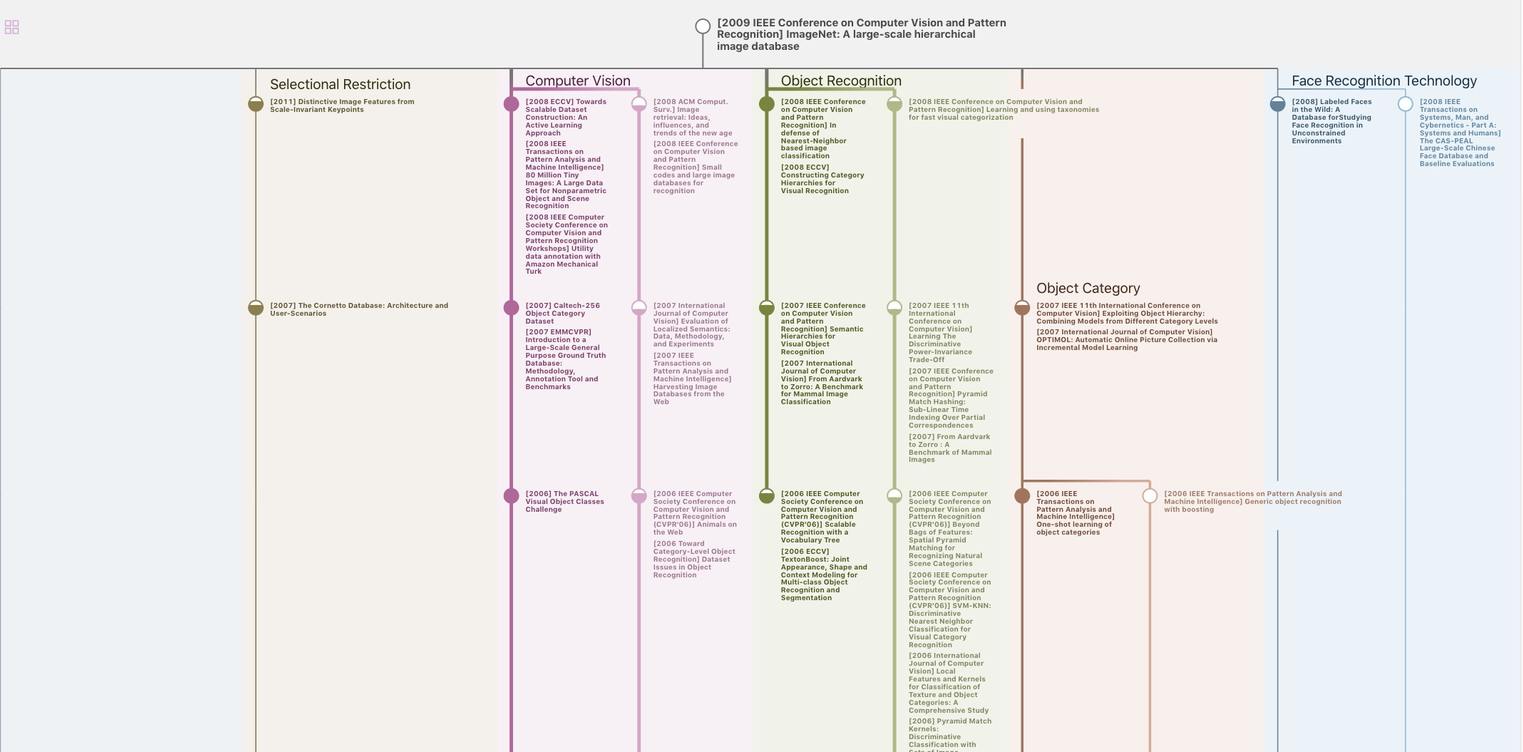
生成溯源树,研究论文发展脉络
Chat Paper
正在生成论文摘要