Joint Power and Trajectory Optimization for IRS-aided Master-Auxiliary-UAV-powered IoT Networks
2021 IEEE GLOBAL COMMUNICATIONS CONFERENCE (GLOBECOM)(2021)
摘要
In this paper, we propose a novel Intelligent Reflected Surface (IRS)-aided Master-Auxiliary-Unmanned Aerial Vehicle (UAV)-powered Internet of Things (IoT) Network (IRS-MAIN). Compared to the conventional terrestrial or aerial IRS-assisted communication networks, the IRS-MAIN not only benefits from wide communication range due to the mobility of UAVs, but also enjoys enhanced channel condition brought by the IRS. To be specific, let the Auxiliary UAV (AUAV) carry an IRS to enhance signals from the Master UAV (MUAV) served as a radio frequency (RF) transmitter. We focus on the problem to maximize the total throughput by jointly optimizing the trajectories and transmit power of the MUAV. A modified multi-agent deep reinforcement learning (MADRL) based algorithm, named as Pre-activation Penalty Multi-agent Deep Deterministic Policy Gradient (PP-MADDPG), is proposed to solve the formulated problem in an accurate and efficient way. Simulation results are provided to demonstrate that PP-MADDPG outperforms the baseline method in terms of the throughput as well as the convergence rate.
更多查看译文
关键词
preactivation penalty multiagent deep deterministic policy gradient,joint power-trajectory optimization,IRS-aided master-auxiliary-UAV-powered IoT networks,IRS-MAIN,conventional terrestrial IRS-assisted communication networks,aerial IRS-assisted communication networks,master UAV,transmit power,modified multiagent deep reinforcement learning based algorithm,intelligent reflected surface-aided master-auxiliary-unmanned aerial vehicle-powered Internet of Things network,AUAV,MUAV,radio frequency transmitter,MADRL,PP-MADDPG
AI 理解论文
溯源树
样例
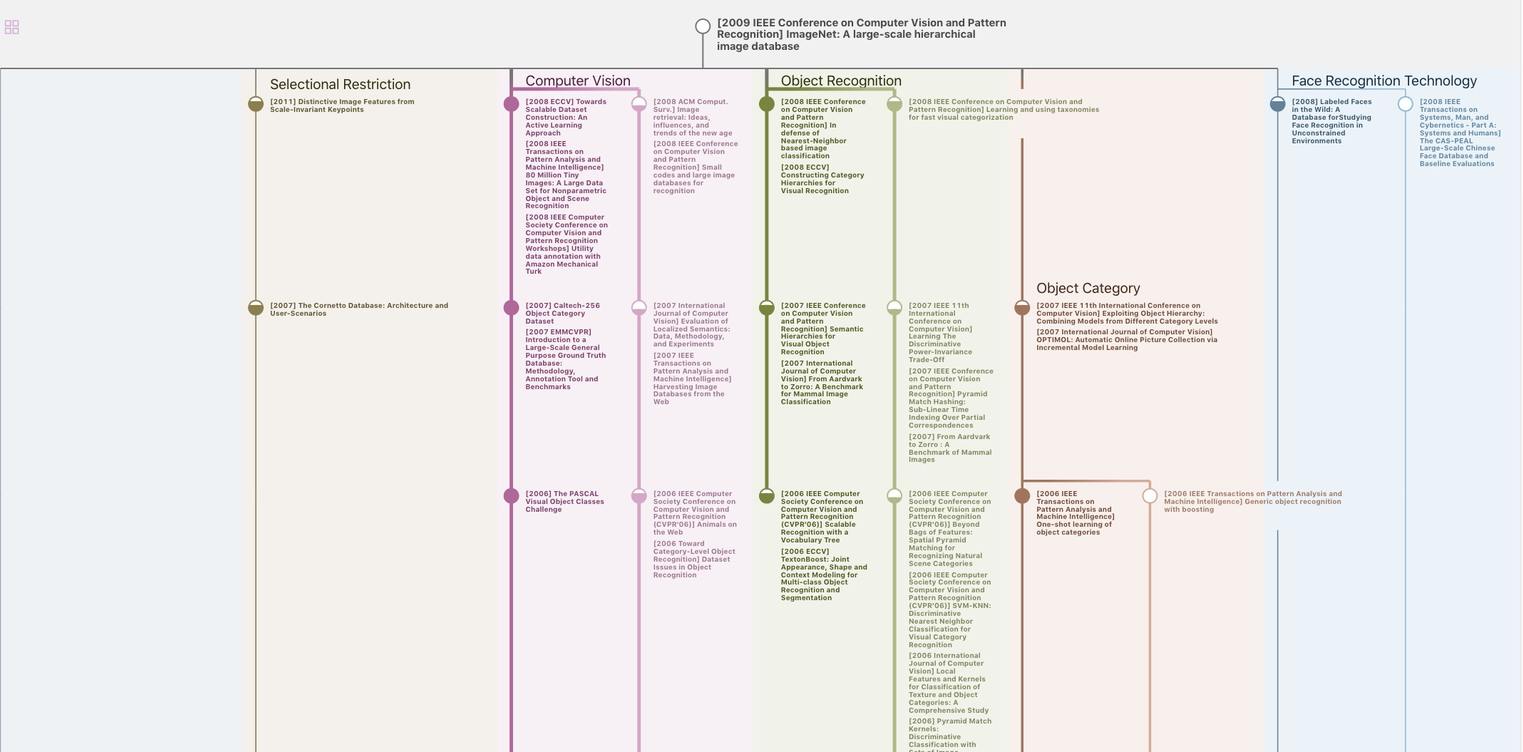
生成溯源树,研究论文发展脉络
Chat Paper
正在生成论文摘要