Temporal Graph Representation Learning for Detecting Anomalies in E-payment Systems
ICDM(2021)
摘要
In this paper, we consider the problem of detecting anomalies in real-world E-payment systems. The E-payment systems can be viewed as a temporal interaction graph, with nodes representing users and edges representing multidimensional transaction sequences between them. To identify illicit users in the E-payment network, we formulate it as a node classification task and apply recent advanced temporal graph representation learning approaches to solve the problem. To fully capture and model the dynamics of the E-payment network, we propose the model, Graph Temporal Edge Aggregation (GTEA), a framework of representation learning for temporal interaction graphs. Instead of aggregating all related interactions of a node over time, GTEA learns to model temporal dynamics for each edge in the graph. Such characteristics enable it to capture pairwise interactional patterns, which can be discriminative for modeling relationships. In addition, a sparsity-inducing self-attention mechanism is incorporated into the framework, which highlights important neighbors and simultaneously filters out a large number of long-tail noises. By combining interactive temporal dynamics with multi-dimensional relational dependencies in a network, GTEA can learn fine-grained representations for entities in an E-payment network, which can be used to distinguish different roles of users for anomaly detections. We demonstrate the effectiveness of GTEA over existing state-of-the-art models with three real-world E-payment datasets on node classification tasks.
更多查看译文
关键词
Edge Embedding,Graph Neural Networks,Temporal Dynamics Modeling
AI 理解论文
溯源树
样例
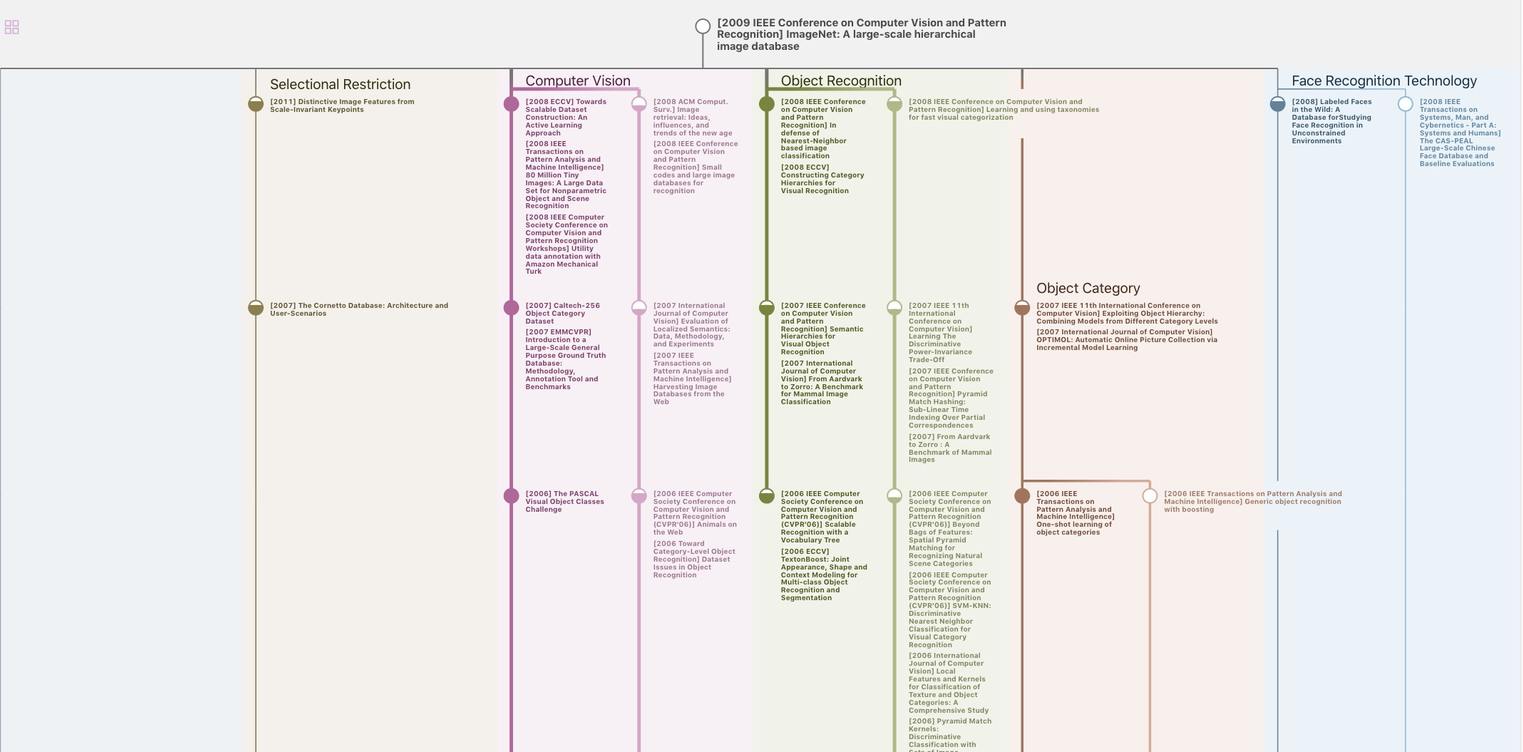
生成溯源树,研究论文发展脉络
Chat Paper
正在生成论文摘要