ROSE-NER - Robust Semi-supervised Named Entity Recognition on Insufficient Labeled Data.
IJCKG(2021)
摘要
With the wide application of deep learning technology in recent years, the effect of named entity recognition tasks has been significantly improved when there is sufficient labeled data. However, obtaining a large amount of labeled data is quite difficult in many domains, for example in the domain of medicine. Noisy data can also negatively affect the robustness of NER models. In this paper, we propose a robust semi-supervised NER approach ROSE-NER to tackle these challenges. A two-step semi-supervised model is introduced to expand a handful of labeled data with a large amount of predicted pseudo-labeled data. The combination of these data will be exploited in the model training. In addition, we introduce an adversarial training method to improve the robustness of the model by eliminating the impact of noise samples. Experiments on medical datasets show that our approach reduces dependency on massive labeled data, and it outperforms other State-of-the-Art approaches.
更多查看译文
关键词
Named Entity Recognition, Semi-Supervision, Adversarial Learning
AI 理解论文
溯源树
样例
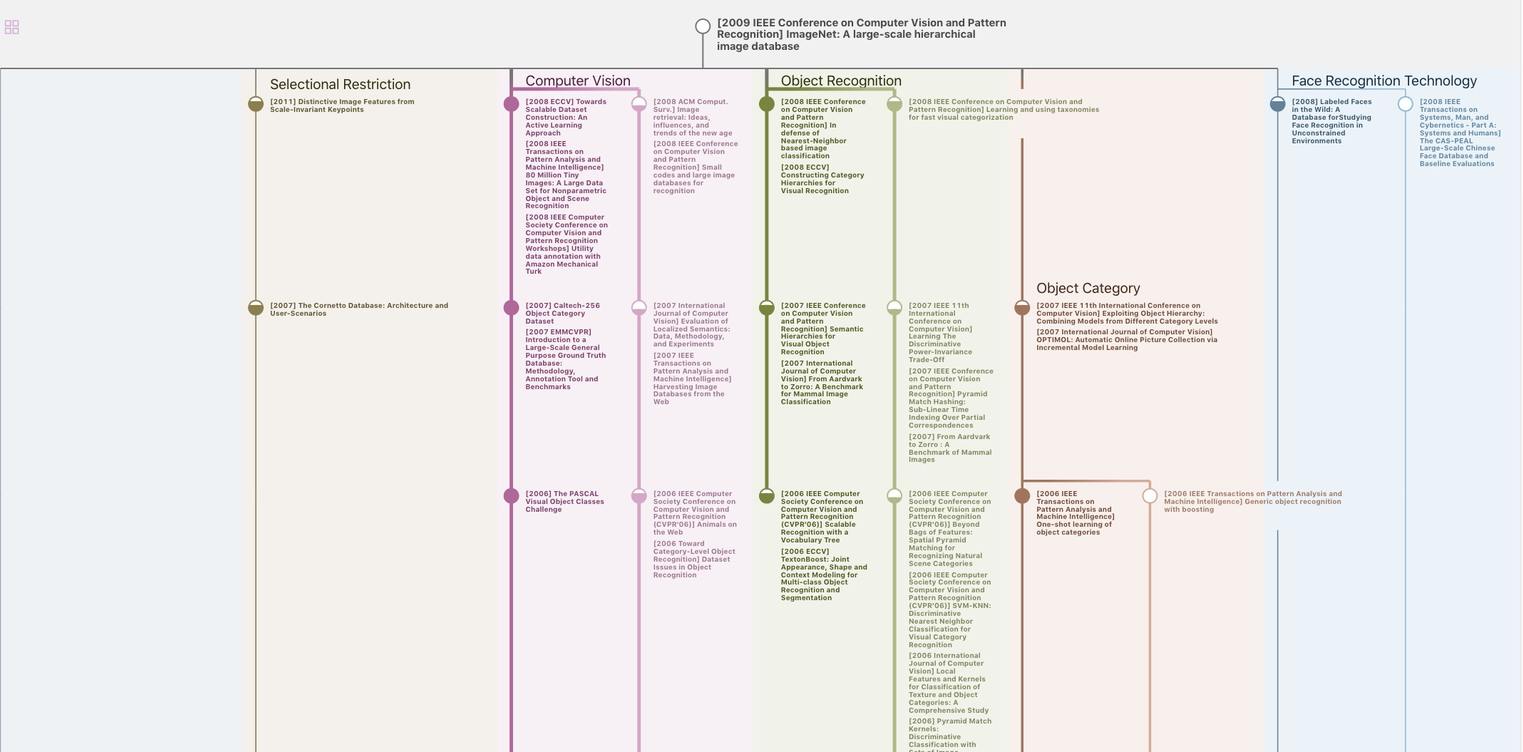
生成溯源树,研究论文发展脉络
Chat Paper
正在生成论文摘要