StarGAT: Star-Shaped Hierarchical Graph Attentional Network for Heterogeneous Network Representation Learning
2021 21ST IEEE INTERNATIONAL CONFERENCE ON DATA MINING (ICDM 2021)(2021)
摘要
Many real-world graphs can be viewed as Heterogeneous Networks or Heterogeneous Information Networks (HINs) for that they comprise a diversity of node types and relation types. Due to the efficient representation ability of Graph Neural Network and the idea of random walk, many recent studies apply graph representation learning to HINs and achieve satisfactory results. However, these works either treat different node types in a metapath equally, which is inconsistent with the original graph semantic information for that different node types should have different statuses, or only consider the first-order (node-level) and second-order (metapath-level, a.k.a. link-level) information aggregation while ignoring the higher-order relations. To tackle these two problems, we propose a novel StarShaped Hierarchical Graph Attentional Network (StarGAT) model to boost representation learning in HINs. Specifically, we assume nodes in HINs can be categorized into a star-shaped structure including one center node type and a bunch of auxiliary node types in a specific task; and we encode node-level, link-level and motif-level attentions in a hierarchical manner to capture richer semantic information. Extensive experiments on three datasets illustrate the model effectiveness.
更多查看译文
关键词
Graph Attentional Network, Network Embedding, Heterogeneous Information Network, Motif
AI 理解论文
溯源树
样例
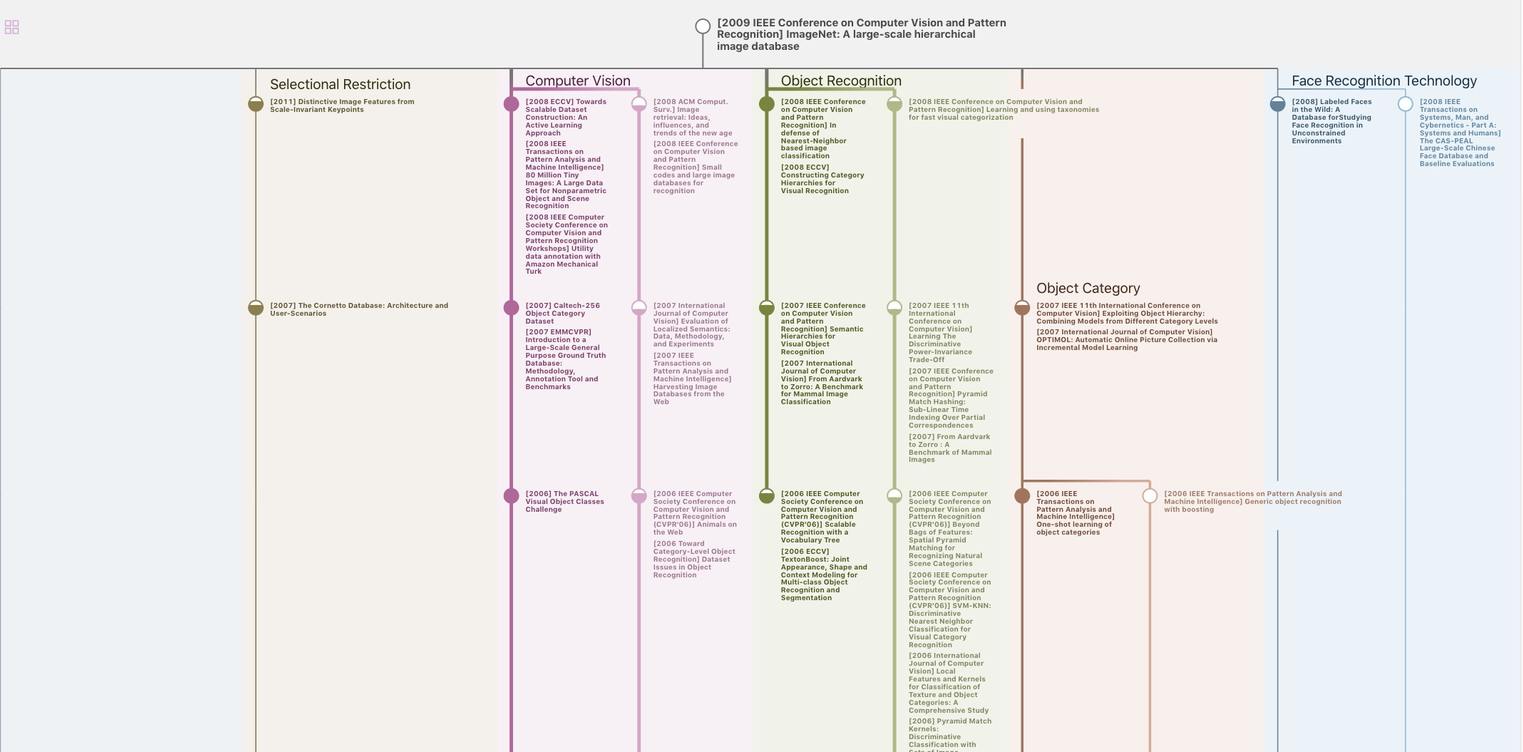
生成溯源树,研究论文发展脉络
Chat Paper
正在生成论文摘要