Limited-memory Common-directions Method With Subsampled Newton Directions for Large-scale Linear Classification
2021 21ST IEEE INTERNATIONAL CONFERENCE ON DATA MINING (ICDM 2021)(2021)
摘要
The common-directions method is an optimization method recently proposed to utilize second-order information. It is especially efficient on large-scale linear classification problems, and it is competitive with state-of-the-art optimization methods like BFGS, LBFGS, and Nesterov's accelerated gradient method. The main idea of the method is to minimize the local quadratic approximation within the selected subspace. Regarding the selection of the subspace, the original authors only focused on the span of current and past gradient directions. In this work, we analyze the impact of subspace selection, and point out that the lack of direction diversity can be a potential weakness for using gradients as directions. To address this problem, we propose the use of subsampled Newton directions, which always possess diversity unless they are already close to the true Newton direction. Our experiments on large-scale linear classification problems show that our proposed methods are generally better than subsampled Newton methods and the original common-directions method.
更多查看译文
关键词
subsampled Newton directions,optimization method,Nesterov accelerated gradient method,past gradient directions,subspace selection,direction diversity,limited-memory common-directions method,second-order information,linear classification problems,local quadratic approximation
AI 理解论文
溯源树
样例
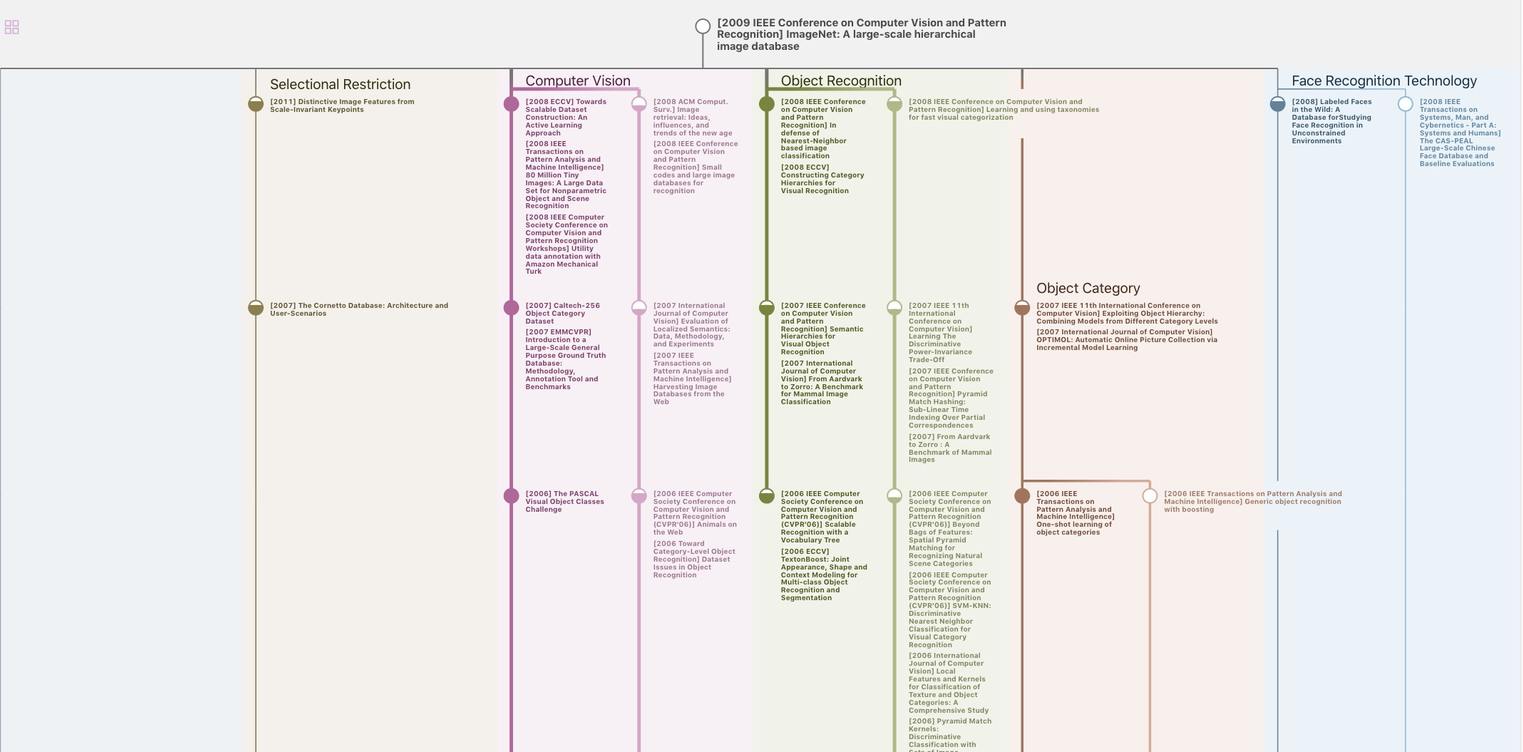
生成溯源树,研究论文发展脉络
Chat Paper
正在生成论文摘要