Jointly Multi-Similarity Loss for Deep Metric Learning
2021 21ST IEEE INTERNATIONAL CONFERENCE ON DATA MINING (ICDM 2021)(2021)
摘要
Deep metric learning has been widely adopted to construct good representations for images and sentences with pair-based loss functions such as contrastive loss and triplet loss. However, these loss functions restrict the effectiveness of learned embedding and face two critical challenges: 1) high bias on account of a large set of uninformative and redundant pairs; 2) low information exploration due to the lack of processing the relation information among pairs. In this paper, we propose the jointly multi-similarity (JMS) loss to address the above challenges with a novel pair weighting strategy that assigns higher weights to the more informative pairs and discards the less informative ones. Specifically, we integrate the relationship information among pairs into a single framework and optimize the JMS loss by considering various information jointly. Furthermore, we extensively compare the JMS loss with other start-of-the-art approaches by conducting multiple experiments on image retrieval and semantic text similarity tasks. The experimental results show that the JMS loss consistently outperforms competitors.
更多查看译文
关键词
deep metric learning, embedding, pair sampling, pair weighting
AI 理解论文
溯源树
样例
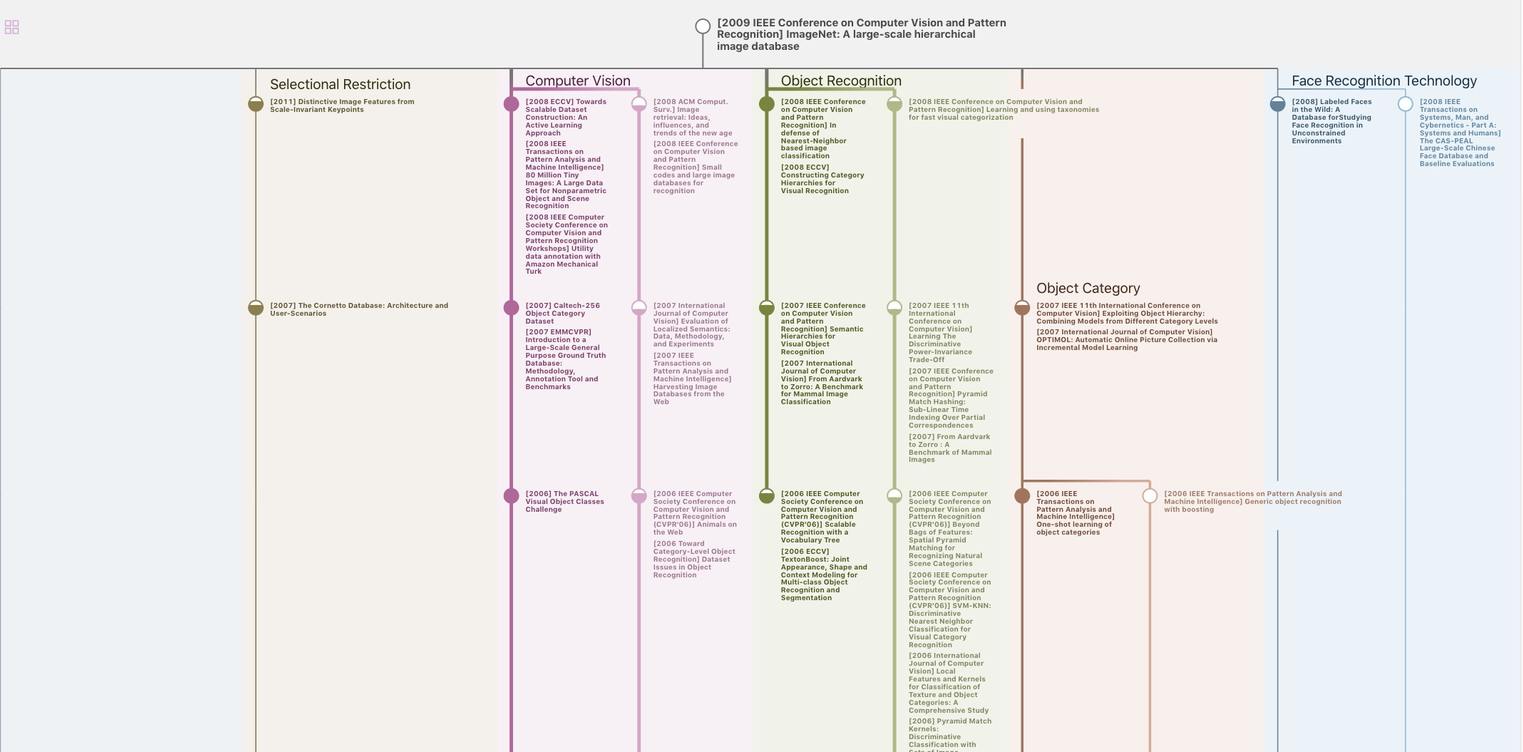
生成溯源树,研究论文发展脉络
Chat Paper
正在生成论文摘要