Learning from Limited Data for Speech-based Traumatic Brain Injury (TBI) Detection
2021 20th IEEE International Conference on Machine Learning and Applications (ICMLA)(2021)
摘要
Due to the high cost of collecting data especially for conditions that afflict a small percentage of the population, data is often scarce in healthcare. Inadequate data presents a challenge for training Deep Neural Networks (DNNs). Many Traumatic Brain Injury (TBI) patients require long periods of recovery with unexpected setbacks and possible rehospitalization, making continuous monitoring important. While speech-based TBI assessment has been found to be effective, available datasets are too small for DNNs. To solve the limited TBI speech data problem, we explore three Learning from Limited Data (LLD) methods (transfer, multi-task and meta-learning) that augment a small primary TBI dataset by learning from external non-TBI datasets to improve DNN performance. We found that all three LLD methods mitigate overfitting, improving binary TBI classification accuracy by 33.6%, 36.7%, and 26.4% respectively. External datasets with scripted speech improved the TBI detection accuracy of all three learning methods the most. Using a few-shot learning approach, we extrapolated results on real data to estimate the full trajectory of expected performance for various amounts of data.
更多查看译文
关键词
Traumatic Brain Injury (TBI),speech assessment,transfer learning,multi-task learning,meta-learning
AI 理解论文
溯源树
样例
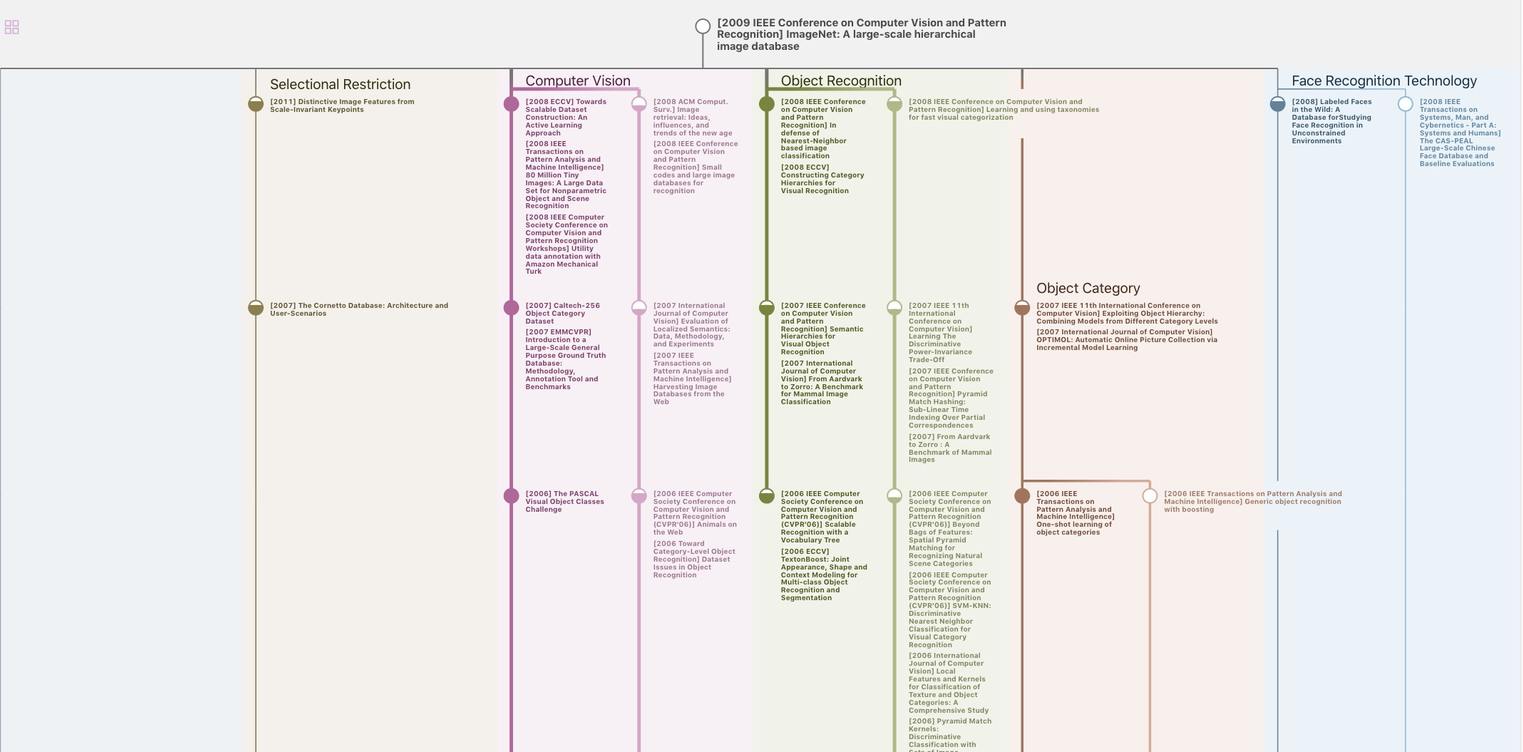
生成溯源树,研究论文发展脉络
Chat Paper
正在生成论文摘要