Automated Hand Osteoarthritis Classification Using Convolutional Neural Networks.
ICMLA(2021)
摘要
Osteoarthritis (OA) is the most common form of arthritis and often occurs in joints such as the knees, hips, and hands. Given there is no cure for OA, early detection and prevention are required to avoid further damage to the joint. Typically, joints are given a Kellgren and Lawrence (KL) grade of 0 to 4 with KL <= 1 meaning non-OA and KL >= 2 being positive for OA. Overall hand OA is determined by a positive OA rating of a joint on more than one finger. Therefore, to detect hand OA, one needs to detect worrisome hand joints first. This study uses a convolutional neural network (CNN) and proposes a custom architecture to automatically classify joints from hand X-rays into 5 KL categories as well as 2 categories of non-OA/OA. Post-processing is used to determine overall hand OA. Using a dataset of 3,556 hand X-rays, our custom CNN architecture was able to achieve a 5-category finger joint classification accuracy of 82.7% with a Matthews correlation coefficient (MCC) of 0.61. For 2-category classification, our model achieved an accuracy of 92.9% with an MCC of 0.74 and an area under the curve (AUC) score of 0.965. Based on the joint-level classification results of each hand, our model achieved an accuracy of 88.6% to classify the hand-level OA, i.e., to distinguish hand X-rays with and without OA. To our knowledge, this is the first work that uses CNN to classify hand joints into KL grades and detect overall hand OA based on individual hand joints.
更多查看译文
关键词
Hand Osteoarthritis,X-ray,Machine Learning,Convolutional Neural Networks
AI 理解论文
溯源树
样例
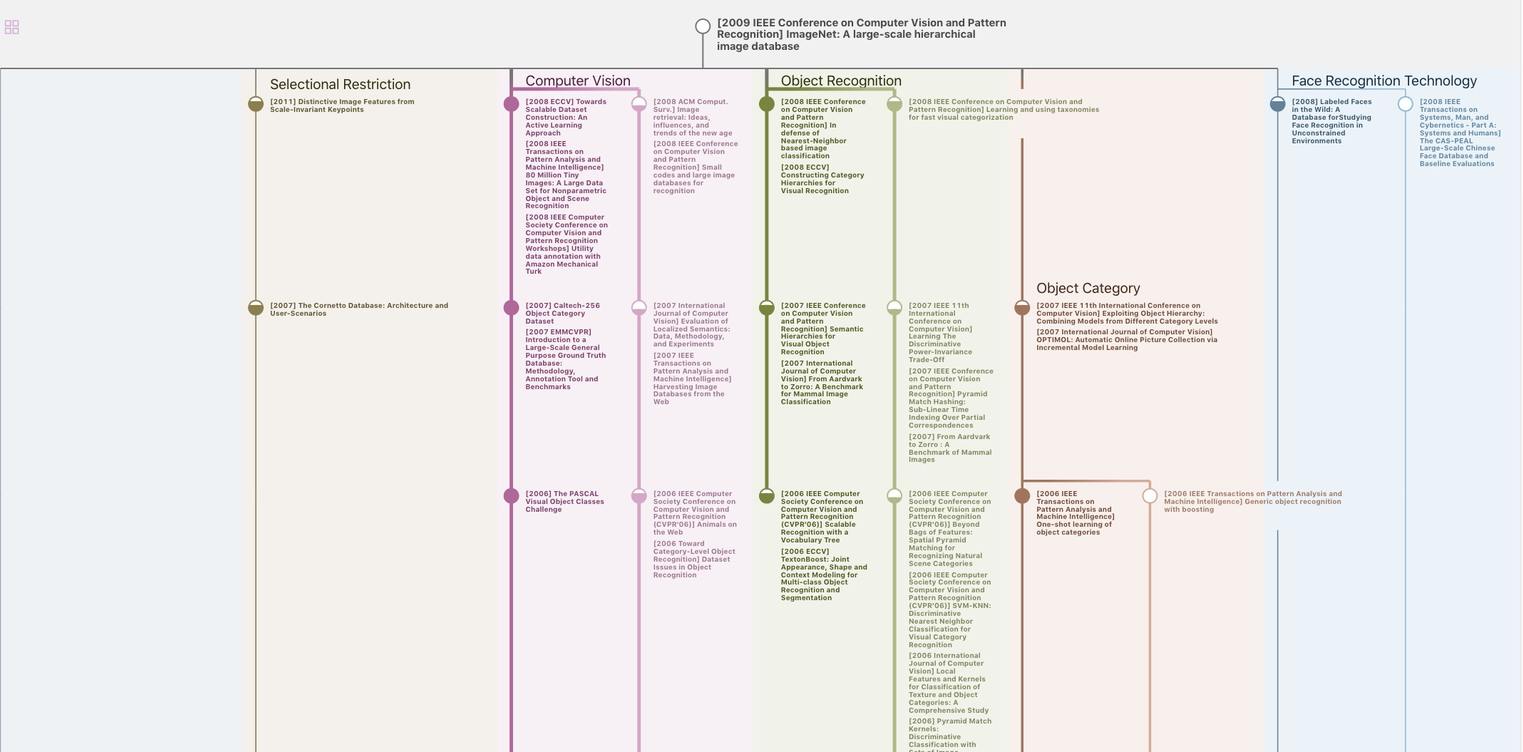
生成溯源树,研究论文发展脉络
Chat Paper
正在生成论文摘要