Quantum Neuron with Separable-State Encoding
arXiv (Cornell University)(2022)
摘要
The use of advanced quantum neuron models for pattern recognition applications requires fault tolerance. Therefore, it is not yet possible to test such models on a large scale in currently available quantum processors. As an alternative, we propose a quantum perceptron (QP) model that uses a reduced number of multi-qubit gates and is therefore less susceptible to quantum errors in current actual quantum computers with limited tolerance. The proposed quantum algorithm is superior to its classical counterpart, although since it does not take full advantage of quantum entanglement, it provides a lower encoding power than other quantum algorithms using multiple qubit entanglement. However, the use of separable-sate encoding allows for testing the algorithm and different training schemes at a large scale in currently available non-fault tolerant quantum computers. We demonstrate the performance of the proposed model by implementing a few qubits version of the QP in a simulated quantum computer. The proposed QP uses an N-ary encoding of the binary input data characterizing the patterns. We develop a hybrid (quantum-classical) training procedure for simulating the learning process of the QP and test their efficiency.
更多查看译文
关键词
quantum neuron,separable-state
AI 理解论文
溯源树
样例
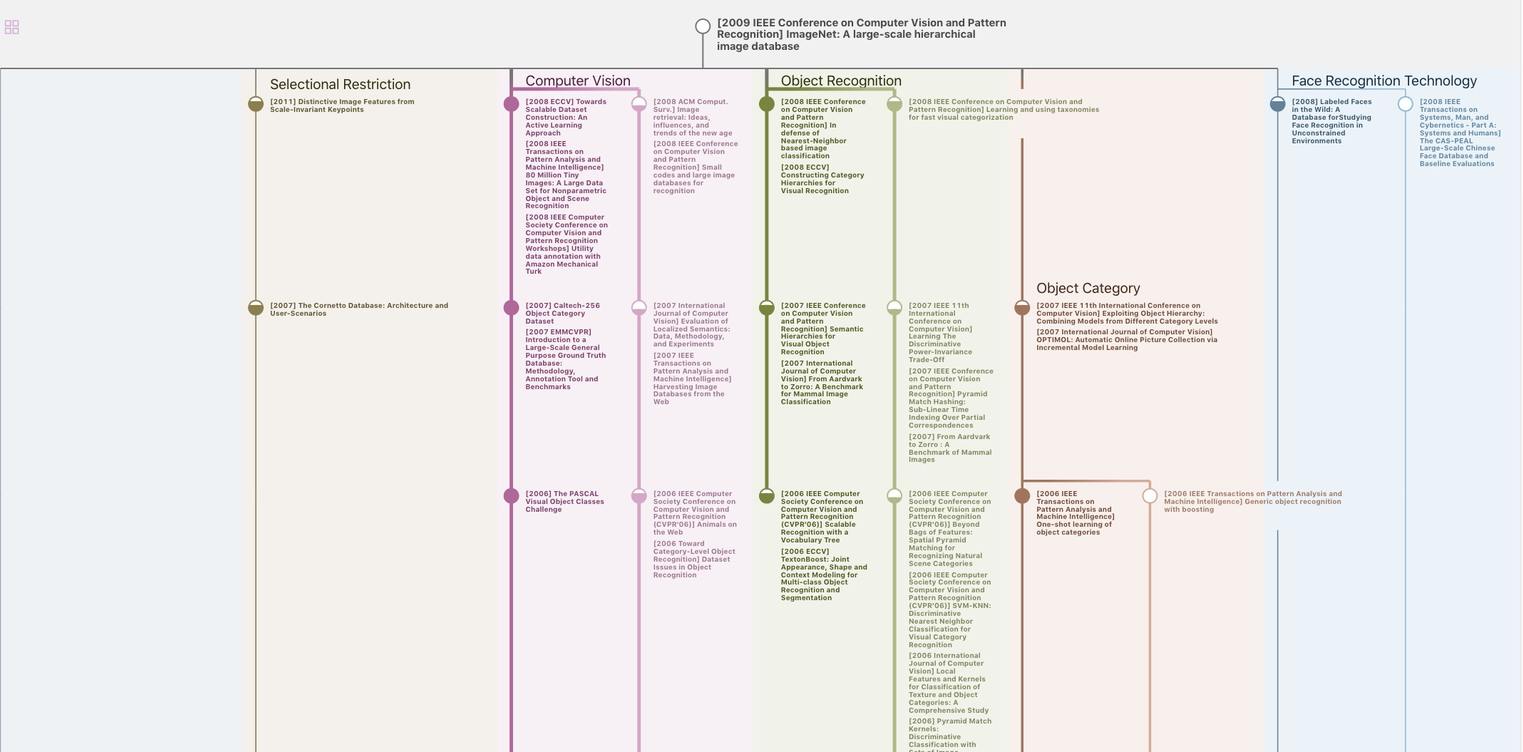
生成溯源树,研究论文发展脉络
Chat Paper
正在生成论文摘要