Placental MRI: Identification of radiological features to predict placental attachment disease regardless of reader expertise
EUROPEAN JOURNAL OF RADIOLOGY(2022)
摘要
Purpose: To compare the accuracy of placental MRI in reporting placental adhesive disease in readers with different expertise and to identify the most reliable MRI features that predict placental pathology regardless of reader expertise.& nbsp;Methods: Retrospective analysis of 27 placental MRI studies by six radiologists with different expertise levels; specificity, sensitivity, and accuracy were used to quantify the predictive performance of eight radiological features previously described in the literature. Histopathological evaluation was used as a diagnostic gold standard when available and the presence of the radiological features was decided by consensus. Features with higher sensitivity and specificity were identified and the optimal cut-off was calculated to obtain the resulting accuracy.& nbsp;Results: The accuracy for seniors with expertise was non-statistically higher (0.83) compared to senior with no expertise (SWE) (0.65) and juniors (0.74) with SWE having tendency to over-estimate the severity of abnormality (26% vs 17%), whilst junior underestimated the degree of placental infiltration when compared to seniors with expertise (18.5% vs 0%, p = 0.006).Dark bands was the criteria with the highest sensitivity (95%) and high specificity (74%), followed by myometrial thinning (89%-76%) and uterine bulging (86%-81%). These three features demonstrated substantial (K) agreement. Using these features with optimal diagnostic cut-off, the accuracy increased to 0.91 for both the seniors and SWE and to 0.93 for the juniors.& nbsp;Conclusion: Placental MRI is most accurately interpreted by experienced radiologists; however, less experienced readers can obtain an accurate diagnosis relying on set criteria that are easier to be identified.
更多查看译文
关键词
Abnormal placentation, Placental MRI, Placental attachment disease
AI 理解论文
溯源树
样例
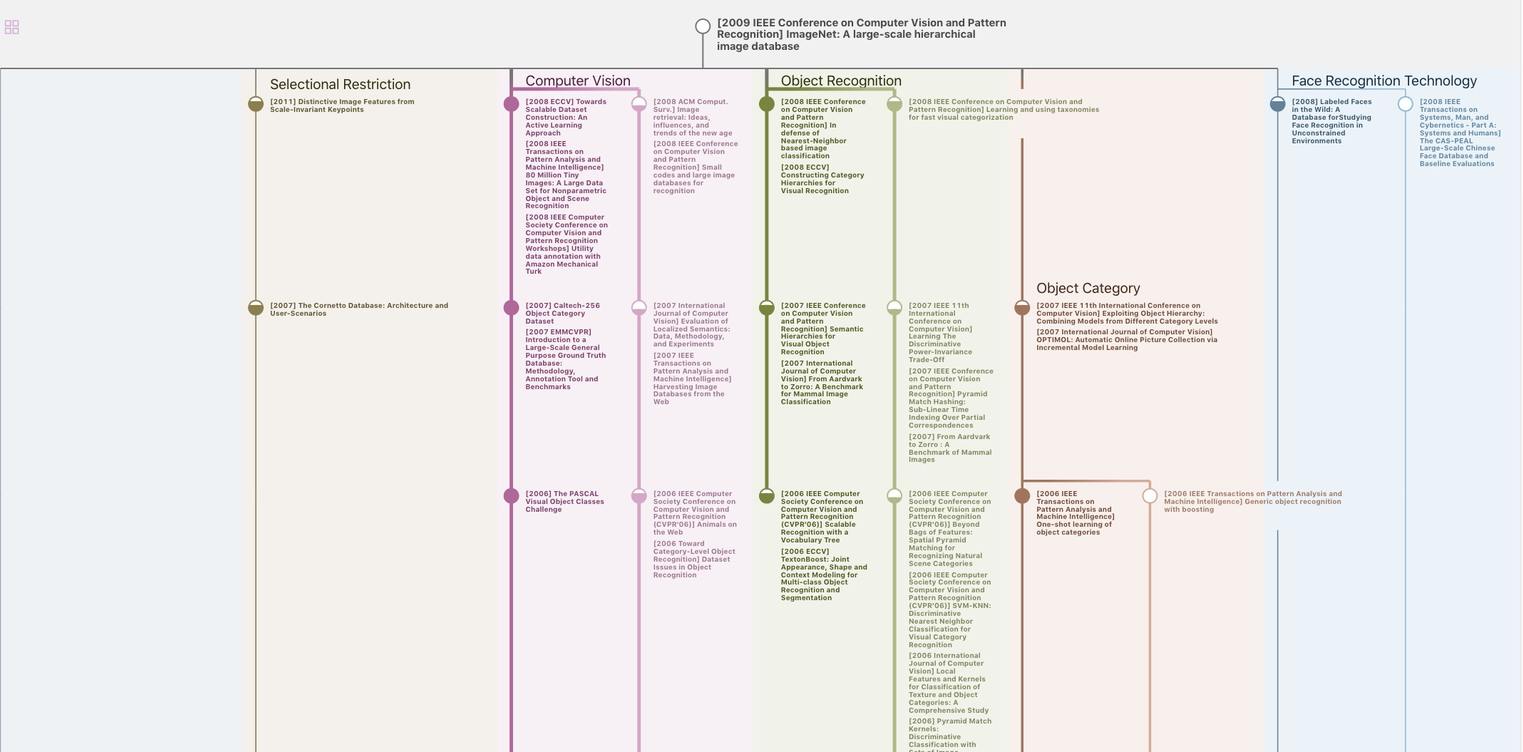
生成溯源树,研究论文发展脉络
Chat Paper
正在生成论文摘要