Automated 3D Fetal Brain Segmentation Using an Optimized Deep Learning Approach
AMERICAN JOURNAL OF NEURORADIOLOGY(2022)
摘要
BACKGROUND AND PURPOSE: MR imaging provides critical information about fetal brain growth and development. Currently, morphologic analysis primarily relies on manual segmentation, which is time-intensive and has limited repeatability. This work aimed to develop a deep learning?based automatic fetal brain segmentation method that provides improved accuracy and robustness compared with atlas-based methods. MATERIALS AND METHODS: A total of 106 fetal MR imaging studies were acquired prospectively from fetuses between 23 and 39?weeks of gestation. We trained a deep learning model on the MR imaging scans of 65 healthy fetuses and compared its performance with a 4D atlas-based segmentation method using the Wilcoxon signed-rank test. The trained model was also evaluated on data from 41 fetuses diagnosed with congenital heart disease. RESULTS: The proposed method showed high consistency with the manual segmentation, with an average Dice score of 0.897. It also demonstrated significantly improved performance (P?.001) based on the Dice score and 95% Hausdorff distance in all brain regions compared with the atlas-based method. The performance of the proposed method was consistent across gestational ages. The segmentations of the brains of fetuses with high-risk congenital heart disease were also highly consistent with the manual segmentation, though the Dice score was 7% lower than that of healthy fetuses. CONCLUSIONS: The proposed deep learning method provides an efficient and reliable approach for fetal brain segmentation, which outperformed segmentation based on a 4D atlas and has been used in clinical and research settings.
更多查看译文
AI 理解论文
溯源树
样例
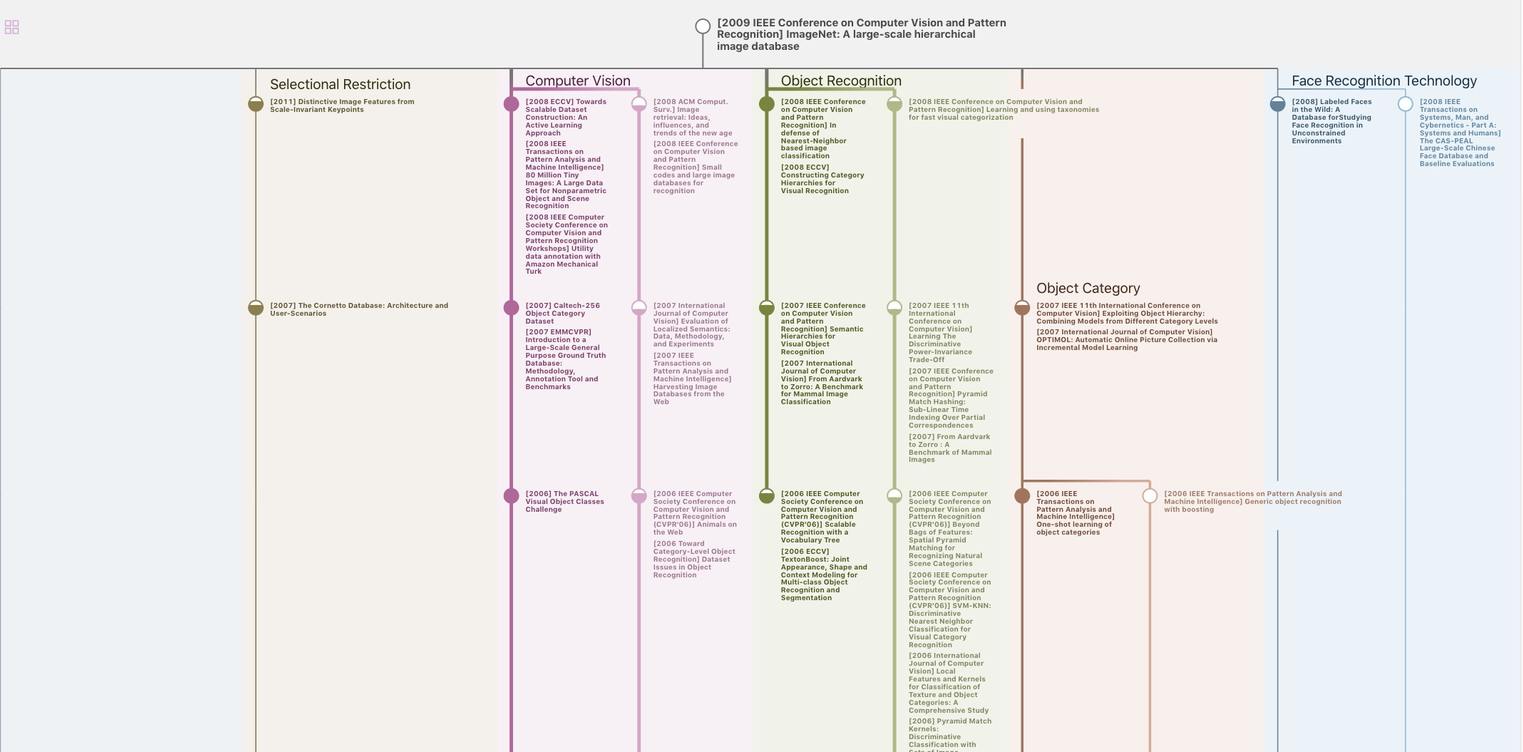
生成溯源树,研究论文发展脉络
Chat Paper
正在生成论文摘要