tinyMAN: Lightweight Energy Manager using Reinforcement Learning for Energy Harvesting Wearable IoT Devices
arxiv(2022)
摘要
Advances in low-power electronics and machine learning techniques lead to many novel wearable IoT devices. These devices have limited battery capacity and computational power. Thus, energy harvesting from ambient sources is a promising solution to power these low-energy wearable devices. They need to manage the harvested energy optimally to achieve energy-neutral operation, which eliminates recharging requirements. Optimal energy management is a challenging task due to the dynamic nature of the harvested energy and the battery energy constraints of the target device. To address this challenge, we present a reinforcement learning-based energy management framework, tinyMAN, for resource-constrained wearable IoT devices. The framework maximizes the utilization of the target device under dynamic energy harvesting patterns and battery constraints. Moreover, tinyMAN does not rely on forecasts of the harvested energy which makes it a prediction-free approach. We deployed tinyMAN on a wearable device prototype using TensorFlow Lite for Micro thanks to its small memory footprint of less than 100 KB. Our evaluations show that tinyMAN achieves less than 2.36 ms and 27.75 $\mu$J while maintaining up to 45% higher utility compared to prior approaches.
更多查看译文
关键词
energy harvesting wearable,lightweight energy manager,reinforcement learning,iot devices
AI 理解论文
溯源树
样例
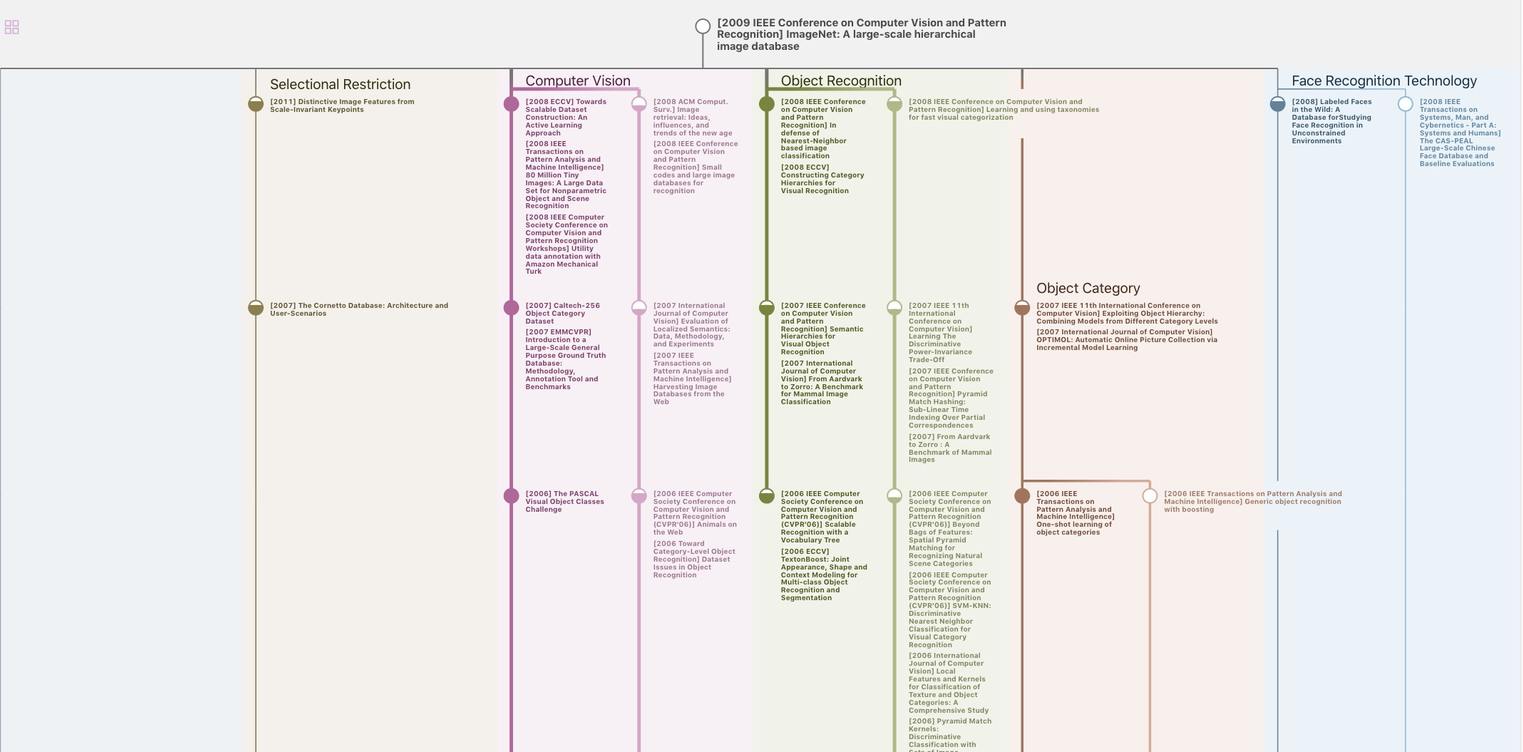
生成溯源树,研究论文发展脉络
Chat Paper
正在生成论文摘要