Physics-informed neural networks for learning the homogenized coefficients of multiscale elliptic equations
Journal of Computational Physics(2022)
摘要
Multiscale elliptic equations with scale separation are often approximated by the corresponding homogenized equations with slowly varying homogenized coefficients (the G-limit). The traditional homogenization techniques typically rely on the periodicity of the multiscale coefficients, thus finding the G-limits often requires sophisticated techniques in more general settings even when multiscale coefficient is known, if possible. Alternatively, we propose a simple approach to estimate the G-limits from (noise-free or noisy) multiscale solution data, either from the existing forward multiscale solvers or sensor measurements. By casting this problem into an inverse problem, our approach adopts physics-informed neural networks (PINNs) algorithm to estimate the G-limits from the multiscale solution data by leveraging a priori knowledge of the underlying homogenized equations. Unlike the existing approaches, our approach does not rely on the periodicity assumption or the known multiscale coefficient during the learning stage, allowing us to estimate homogenized coefficients in more general settings beyond the periodic setting. We demonstrate that the proposed approach can deliver reasonable and accurate approximations to the G-limits as well as homogenized solutions through several benchmark problems.
更多查看译文
关键词
Physics-informed neural network,Multiscale,Homogenization,G-limit,G-convergence
AI 理解论文
溯源树
样例
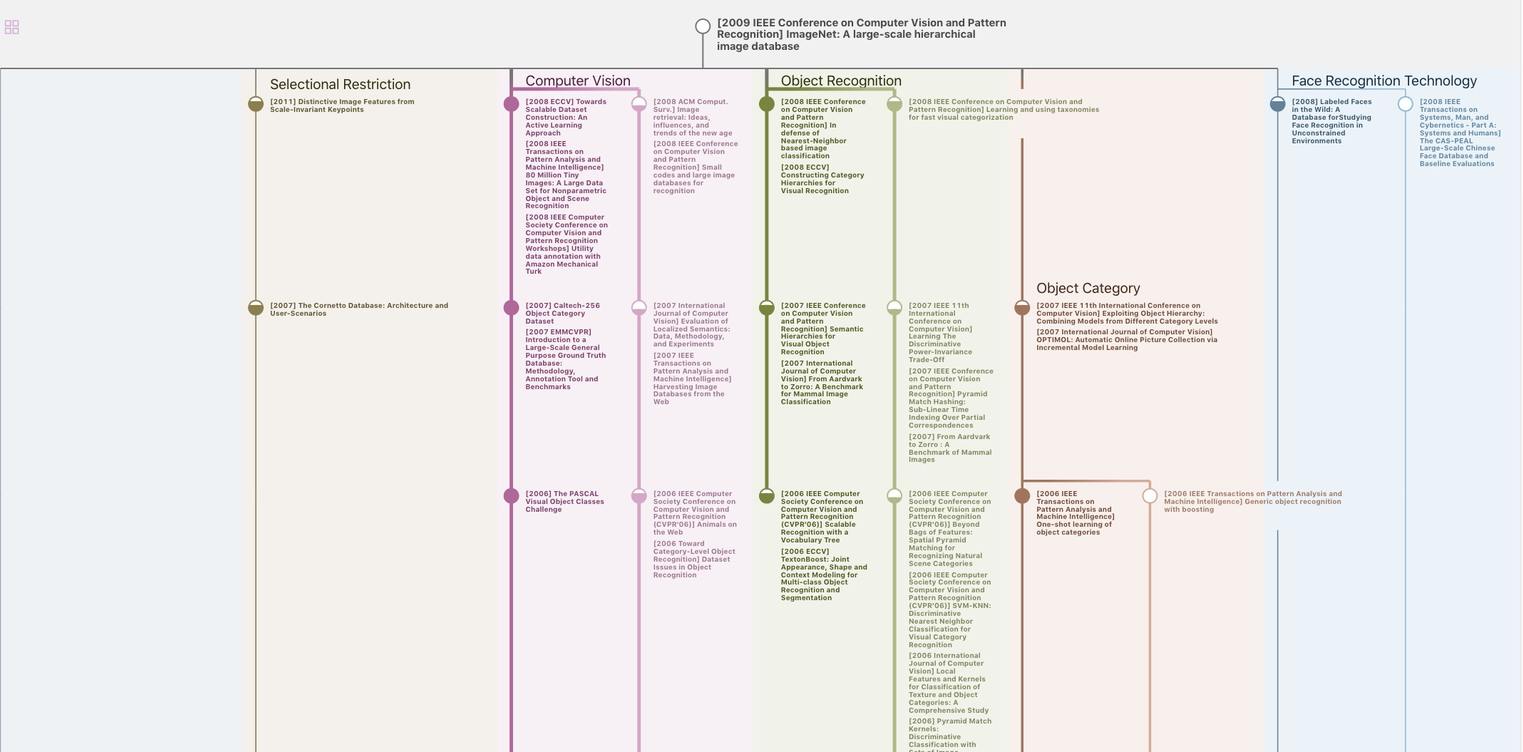
生成溯源树,研究论文发展脉络
Chat Paper
正在生成论文摘要