Domain-Augmented Domain Adaptation
CoRR(2022)
摘要
Unsupervised domain adaptation (UDA) enables knowledge transfer from the labelled source domain to the unlabeled target domain by reducing the cross-domain discrepancy. However, most of the studies were based on direct adaptation from the source domain to the target domain and have suffered from large domain discrepancies. To overcome this challenge, in this paper, we propose the domain-augmented domain adaptation (DADA) to generate pseudo domains that have smaller discrepancies with the target domain, to enhance the knowledge transfer process by minimizing the discrepancy between the target domain and pseudo domains. Furthermore, we design a pseudo-labeling method for DADA by projecting representations from the target domain to multiple pseudo domains and taking the averaged predictions on the classification from the pseudo domains as the pseudo labels. We conduct extensive experiments with the state-of-the-art domain adaptation methods on four benchmark datasets: Office Home, Office-31, VisDA2017, and Digital datasets. The results demonstrate the superiority of our model.
更多查看译文
关键词
adaptation,domain-augmented
AI 理解论文
溯源树
样例
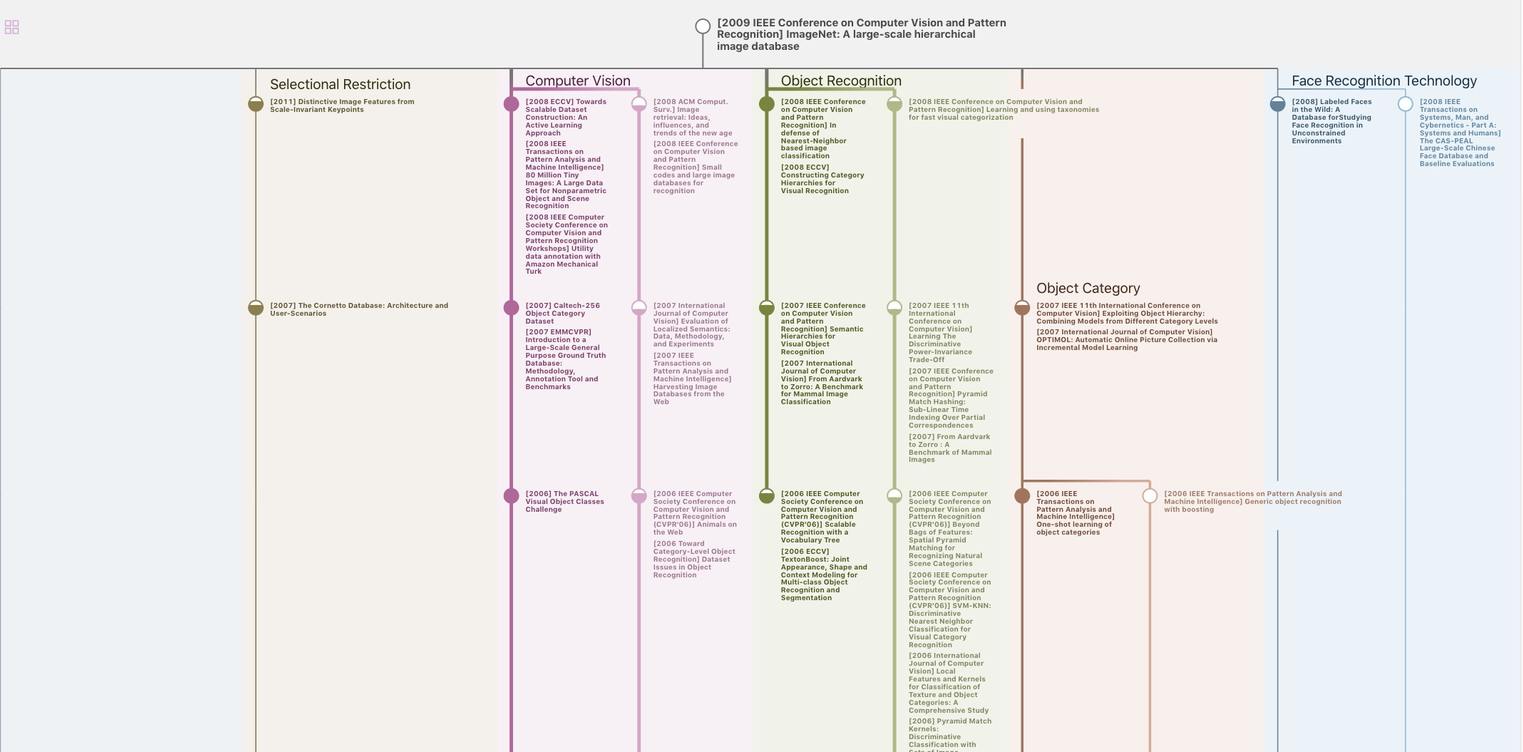
生成溯源树,研究论文发展脉络
Chat Paper
正在生成论文摘要