Learning Causal Overhypotheses through Exploration in Children and Computational Models.
Conference on Causal Learning and Reasoning (CLeaR)(2022)
摘要
Despite recent progress in reinforcement learning (RL), RL algorithms for exploration still remain an active area of research. Existing methods often focus on state-based metrics, which do not consider the underlying causal structures of the environment, and while recent research has begun to explore RL environments for causal learning, these environments primarily leverage causal information through causal inference or induction rather than exploration. In contrast, human children - some of the most proficient explorers - have been shown to use causal information to great benefit. In this work, we introduce a novel RL environment designed with a controllable causal structure, which allows us to evaluate exploration strategies used by both agents and children in a unified environment. In addition, through experimentation on both computation models and children, we demonstrate that there are significant differences between information-gain optimal RL exploration in causal environments and the exploration of children in the same environments. We conclude with a discussion of how these findings may inspire new directions of research into efficient exploration and disambiguation of causal structures for RL algorithms.
更多查看译文
关键词
causal overhypotheses,learning,children,exploration
AI 理解论文
溯源树
样例
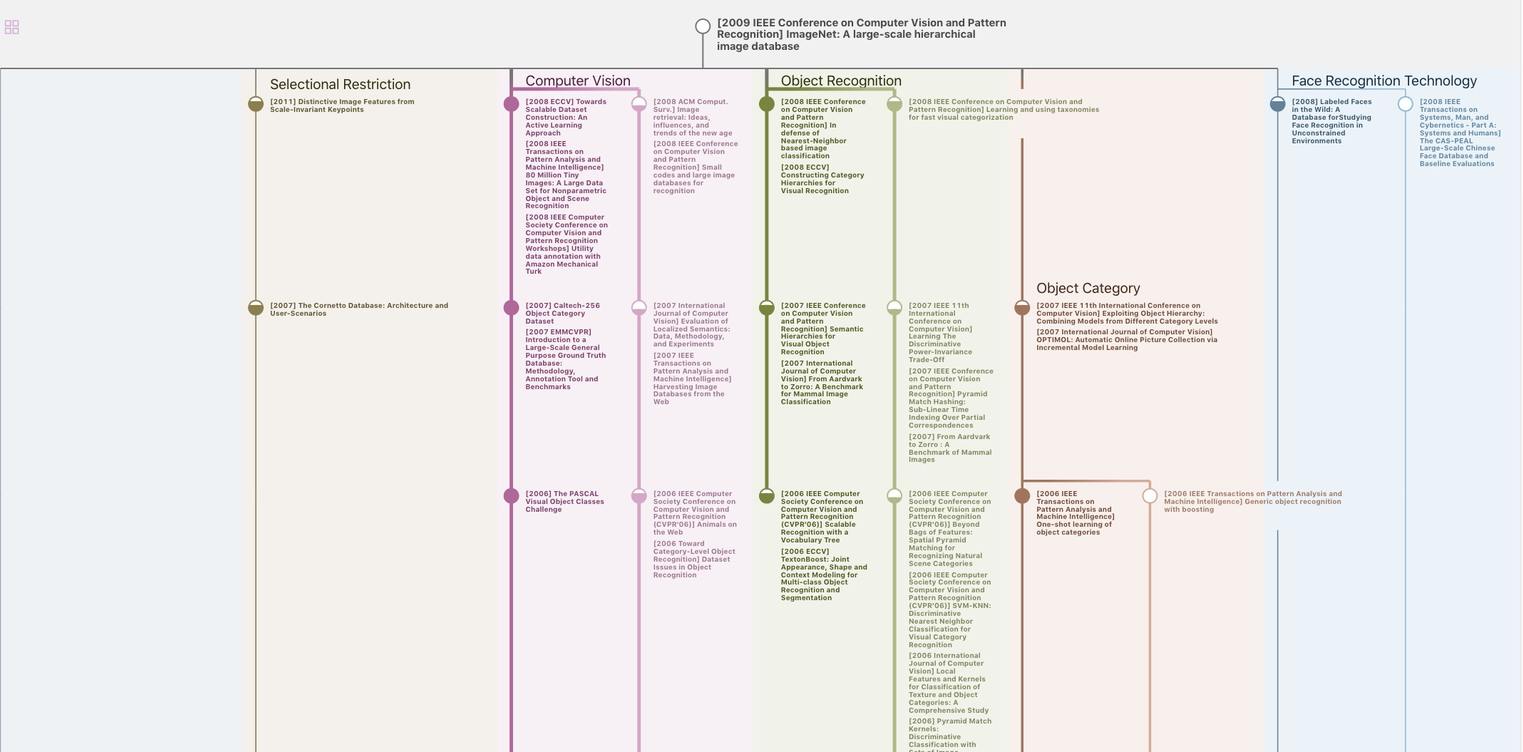
生成溯源树,研究论文发展脉络
Chat Paper
正在生成论文摘要