ISIATasker: Task Allocation for Instant-SensingߝInstant-Actuation Mobile Crowdsensing
IEEE internet of things journal(2022)
摘要
Task allocation is a key issue in mobile crowdsensing (MCS), which affects the sensing efficiency and quality. Previous studies focus on the allocation of tasks that have already been published to the platform, but there are some very urgent tasks that need to be executed once they were detected. Existing studies for either delay-tolerant or time-sensitive tasks have a certain time delay from task publishing to execution, so it is impossible to achieve task detection then execution seamlessly. Thus, we first define the instant sensing and then instant actuation (ISIA) problem in MCS and propose a new model to solve it. We aim to allocate POIs where ISIA tasks are most likely to be detected to workers with similar sensing types so that these tasks can be executed once they are detected. This article presents a two-phase task allocation framework called ISIATasker. In the sensing locations clustering and sensor selection phase, we cluster independent sensing locations into several POIs and then select the optimal cooperative sensor set for each POI to assist workers in completing sensing. In the POIs allocation phase, we propose a method called PA-DDQN based on deep reinforcement learning to plan an optimal path for each worker, thus maximizing the overall sensing type matching degree and POI coverage to enable ISIA. Finally, extensive experiments are conducted based on real-world data sets to demonstrate that the matching degree and POI coverage of ISIATasker outperform other baselines.
更多查看译文
关键词
Deep reinforcement learning (RL),mobile crowdsensing (MCS),task allocation,task urgency
AI 理解论文
溯源树
样例
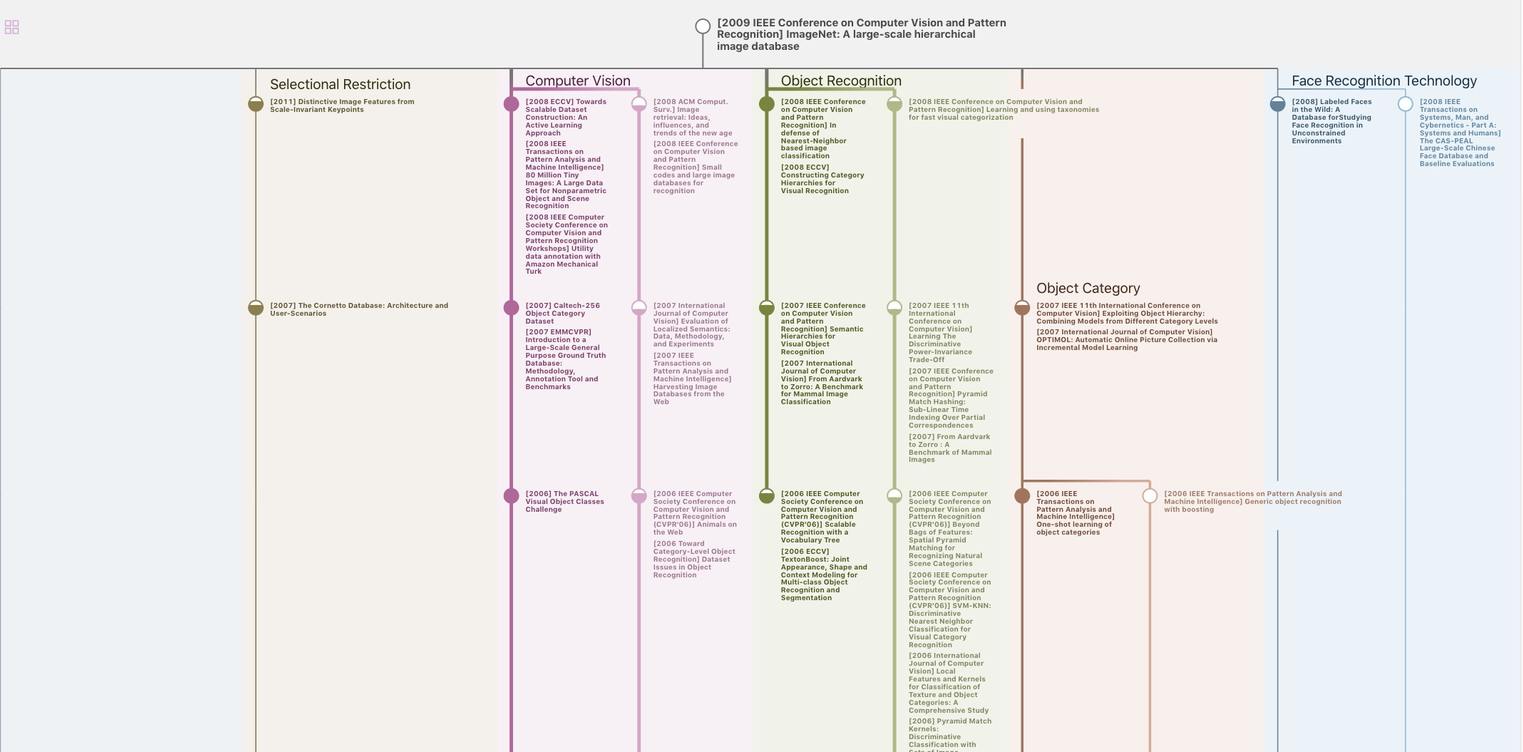
生成溯源树,研究论文发展脉络
Chat Paper
正在生成论文摘要