Inference and Learning with Model Uncertainty in Probabilistic Logic Programs.
AAAI Conference on Artificial Intelligence(2022)
摘要
An issue that has so far received only limited attention in probabilistic logic programming (PLP) is the modelling of so-called epistemic uncertainty, the uncertainty about the model itself. Accurately quantifying this model uncertainty is paramount to robust inference, learning and ultimately decision making. We introduce BetaProbLog, a PLP language that can model epistemic uncertainty. BetaProbLog has sound semantics, an effective inference algorithm that combines Monte Carlo techniques with knowledge compilation, and a parameter learning algorithm. We empirically outperform state-of-the-art methods on probabilistic inference tasks in second-order Bayesian networks, digit classification and discriminative learning in the presence of epistemic uncertainty.
更多查看译文
关键词
Reasoning Under Uncertainty (RU)
AI 理解论文
溯源树
样例
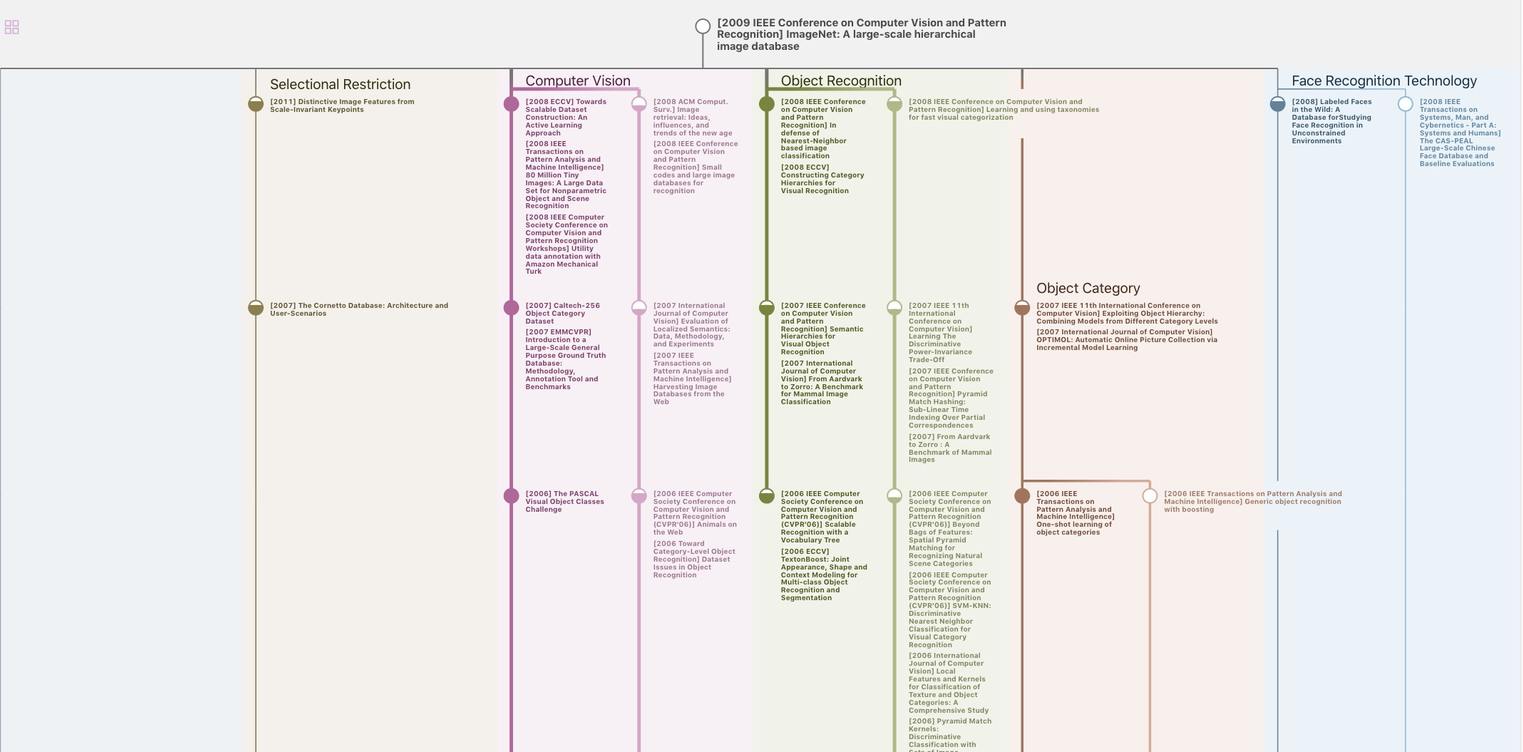
生成溯源树,研究论文发展脉络
Chat Paper
正在生成论文摘要