Towards Explainable Action Recognition by Salient Qualitative Spatial Object Relation Chains.
AAAI Conference on Artificial Intelligence(2022)
摘要
In order to be trusted by humans, Artificial Intelligence agents should be able to describe rationales behind their decisions. One such application is human action recognition in critical or sensitive scenarios, where trustworthy and explainable action recognizers are expected. For example, reliable pedestrian action recognition is essential for self-driving cars and explanations for real-time decision making are critical for investigations if an accident happens. In this regard, learning-based approaches, despite their popularity and accuracy, are disadvantageous due to their limited interpretability. This paper presents a novel neuro-symbolic approach that recognizes actions from videos with human-understandable explanations. Specifically, we first propose to represent videos symbolically by qualitative spatial relations between objects called qualitative spatial object relation chains. We further develop a neural saliency estimator to capture the correlation between such object relation chains and the occurrence of actions. Given an unseen video, this neural saliency estimator is able to tell which object relation chains are more important for the action recognized. We evaluate our approach on two real-life video datasets, with respect to recognition accuracy and the quality of generated action explanations. Experiments show that our approach achieves superior performance on both aspects to previous symbolic approaches, thus facilitating trustworthy intelligent decision making. Our approach can be used to augment state-of-the-art learning approaches with explainabilities.
更多查看译文
关键词
Knowledge Representation And Reasoning (KRR),Cognitive Modeling & Cognitive Systems (CMS),Computer Vision (CV),Intelligent Robotics (ROB)
AI 理解论文
溯源树
样例
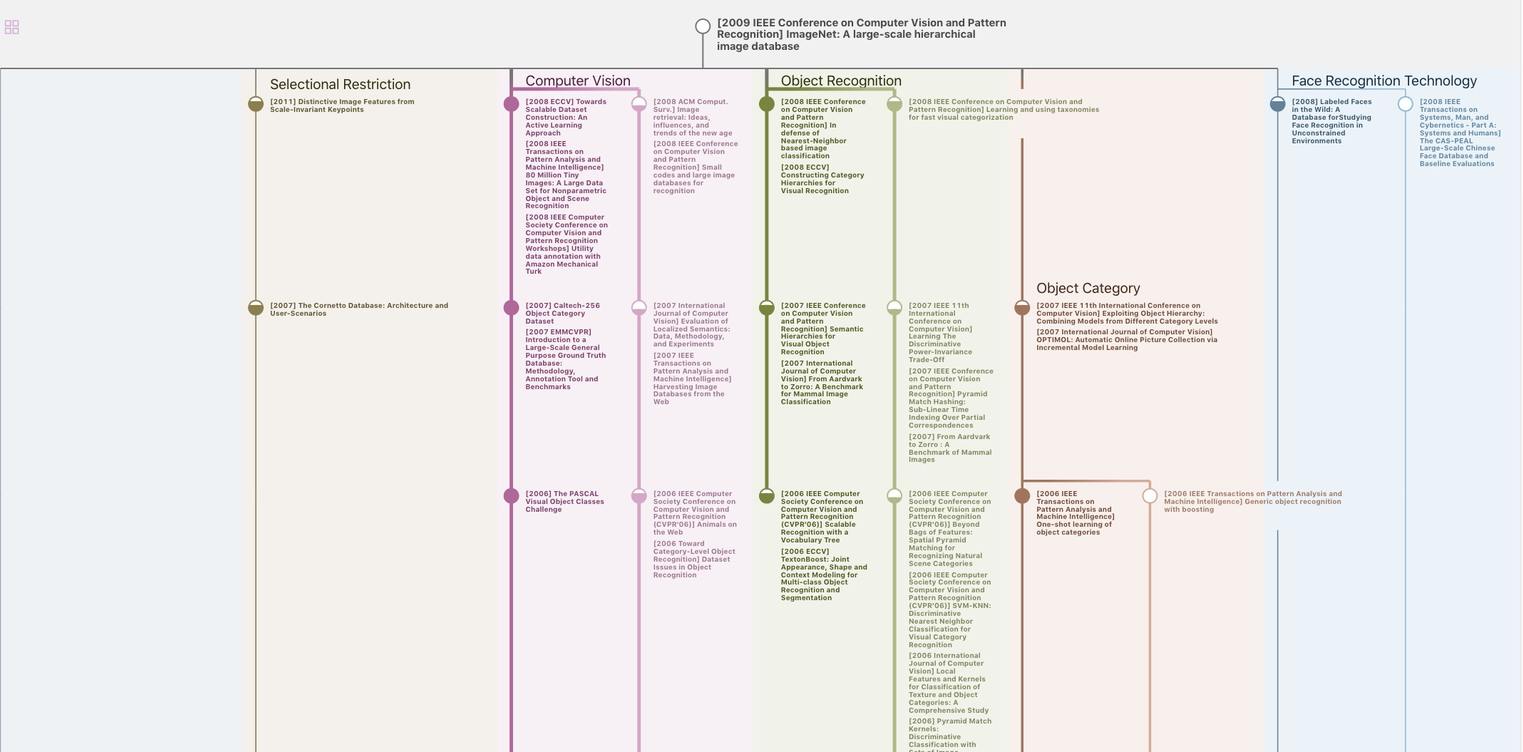
生成溯源树,研究论文发展脉络
Chat Paper
正在生成论文摘要