A Discriminative and Robust Feature Learning Approach for EEG-Based Motor Imagery Decoding (Student Abstract).
AAAI Conference on Artificial Intelligence(2022)
摘要
Convolutional neural networks (CNNs) have been commonly applied in the area of the Electroencephalography (EEG)-based Motor Imagery (MI) classification, significantly pushing the boundary of the state-of-the-art. In order to simultaneously decode the discriminative features and eliminate the negative effects of non-Gaussian noise and outliers in the motor imagery data, in this abstract, we propose a novel robust supervision signal, called Correntropy based Center Loss (CCL), for CNN training, which utilizes the correntropy induced distance as the objective measure. It is encouraging to see that the CNN model trained by the combination of softmax loss and CCL loss outperforms the state-of-the-art models on two public datasets.
更多查看译文
关键词
Correntropy,Center Loss,Convolutional Neural Networks,Motor Imagery
AI 理解论文
溯源树
样例
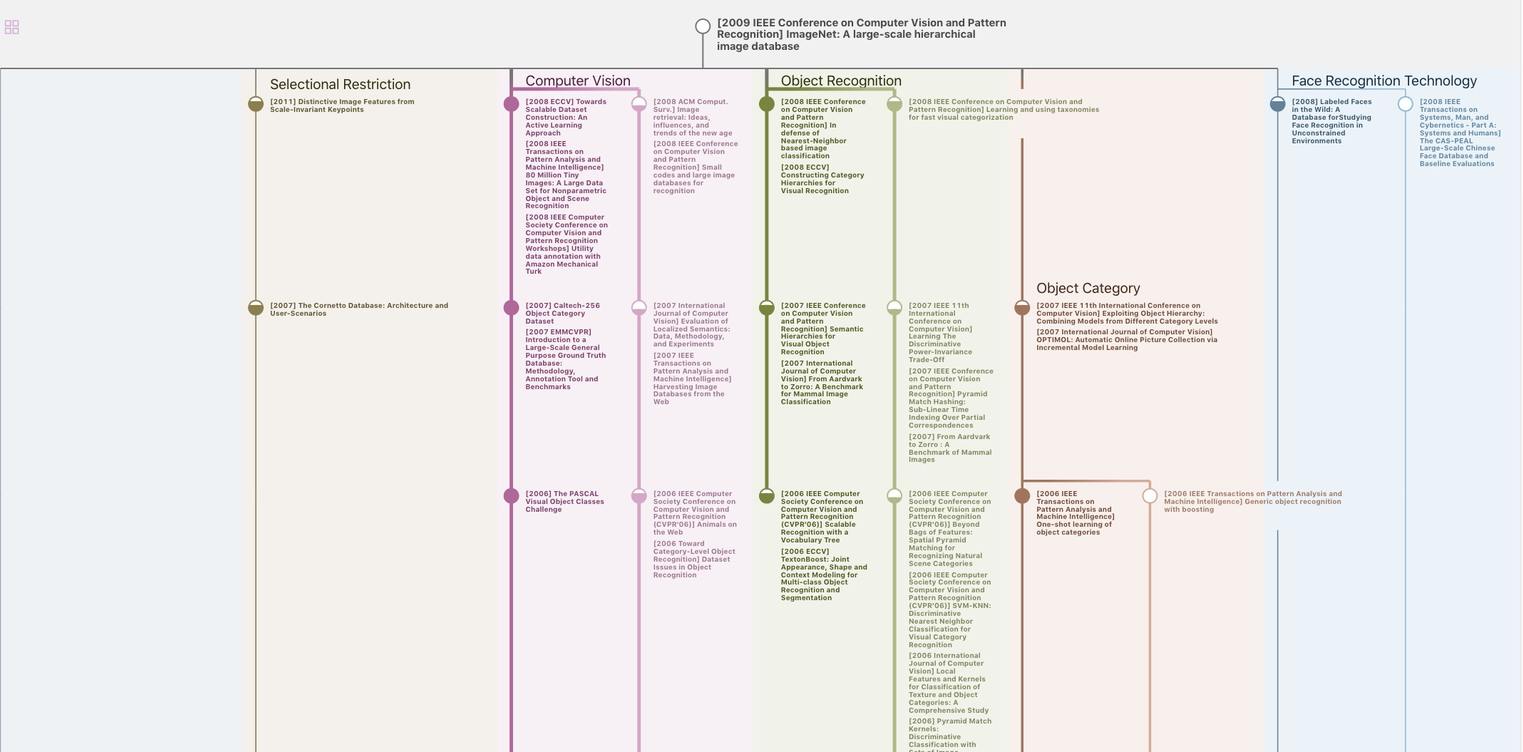
生成溯源树,研究论文发展脉络
Chat Paper
正在生成论文摘要