HCRNet: high-throughput circRNA-binding event identification from CLIP-seq data using deep temporal convolutional network
BRIEFINGS IN BIOINFORMATICS(2022)
摘要
Identifying genome-wide binding events between circular RNAs (circRNAs) and RNA-binding proteins (RBPs) can greatly facilitate our understanding of functional mechanisms within circRNAs. Thanks to the development of cross-linked immunoprecipitation sequencing technology, large amounts of genome-wide circRNA binding event data have accumulated, providing opportunities for designing high-performance computational models to discriminate RBP interaction sites and thus to interpret the biological significance of circRNAs. Unfortunately, there are still no computational models sufficiently flexible to accommodate circRNAs from different data scales and with various degrees of feature representation. Here, we present HCRNet, a novel end-to-end framework for identification of circRNA-RBP binding events. To capture the hierarchical relationships, the multi-source biological information is fused to represent circRNAs, including various natural language sequence features. Furthermore, a deep temporal convolutional network incorporating global expectation pooling was developed to exploit the latent nucleotide dependencies in an exhaustive manner. We benchmarked HCRNet on 37 circRNA datasets and 31 linear RNA datasets to demonstrate the effectiveness of our proposed method. To evaluate further the model's robustness, we performed HCRNet on a full-length dataset containing 740 circRNAs. Results indicate that HCRNet generally outperforms existing methods. In addition, motif analyses were conducted to exhibit the interpretability of HCRNet on circRNAs. All supporting source code and data can be downloaded from and . And the web server of HCRNet is publicly accessible at .
更多查看译文
关键词
deep learning, deep temporal convolutional network, circRNA-RBP binding event identification, natural language processing
AI 理解论文
溯源树
样例
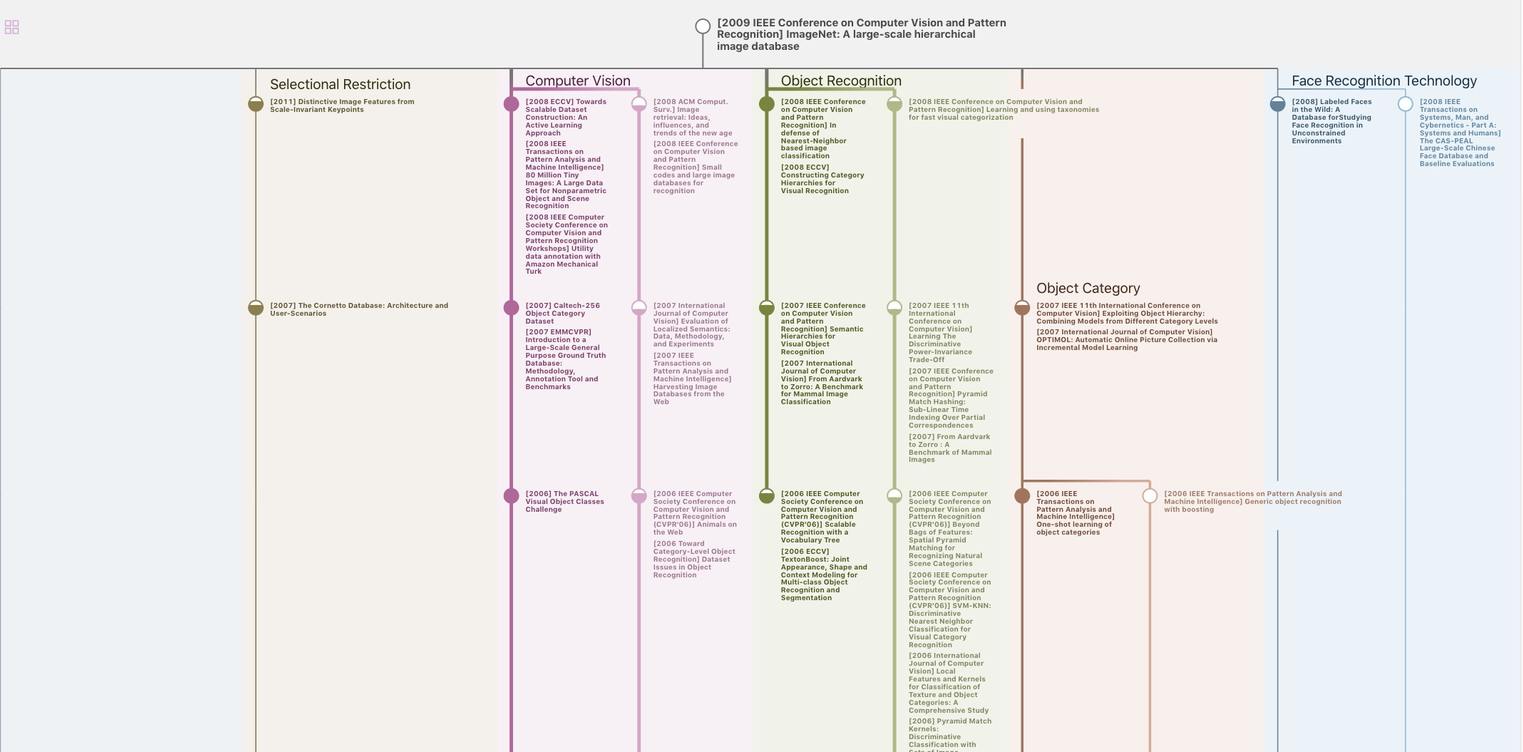
生成溯源树,研究论文发展脉络
Chat Paper
正在生成论文摘要