A two-stage multiresolution neural network for automatic diagnosis of hepatic echinococcosis from ultrasound images: A multicenter study
MEDICAL PHYSICS(2022)
摘要
Purpose Hepatic echinococcosis is a parasitic disease. Ultrasound imaging is a crucially important tool for the diagnosis of this disease. Based on ultrasonic manifestations, hepatic echinococcosis can be classified into many subtypes. However, subtyping is nontrivial due to the challenges of complex sonographic textures and large intraclass and small interclass differences. The purpose of this study is to develop a computer-aided diagnosis system for hepatic echinococcosis based on ultrasound images. Methods We collected a multicenter ultrasound dataset containing 9112 images from 5028 patients who were diagnosed with hepatic echinococcosis (the largest cohort to date) and developed a two-stage multiresolution neural network for the automatic diagnosis of hepatic echinococcosis into nine subtypes, as suggested by the WHO. Our method was based on YOLO3 with two additional strategies to improve its performance: coarse grouping and multiresolution sampling. Considering that some subtypes are inherently very similar and difficult to differentiate; in the first stage, we detected and classified lesions into four coarse groups instead of making a direct classification into nine classes. In the second stage, we performed fine-grained classification within each coarse group. Multiple views with different resolutions were sampled from the detected lesions and were input into Darknet53. The softmax outputs for the multiresolution views were averaged to generate the final output. Results Both the proposed coarse grouping and multiresolution sampling strategies proved to be effective and improved the classification performance by a large margin compared with the setting without using the two strategies. Using fivefold cross-validation, our method achieved 87.1%, 86.2%, and 86.5% in the average recall, precision and F1-score, respectively, and outperformed other state-of-the-art methods remarkably. Conclusions The experimental results demonstrate the great promise of our method for classifying hepatic echinococcosis. Our method can be used as an effective tool to facilitate large-scale screening for hepatic echinococcosis in high-risk, resource-poor areas, thus contributing to the early diagnosis of this disease and resulting in more successful treatment.
更多查看译文
关键词
computer-aided diagnosis, deep learning, hepatic echinococcosis diagnosis, lesion classification, lesion detection, ultrasound image analysis
AI 理解论文
溯源树
样例
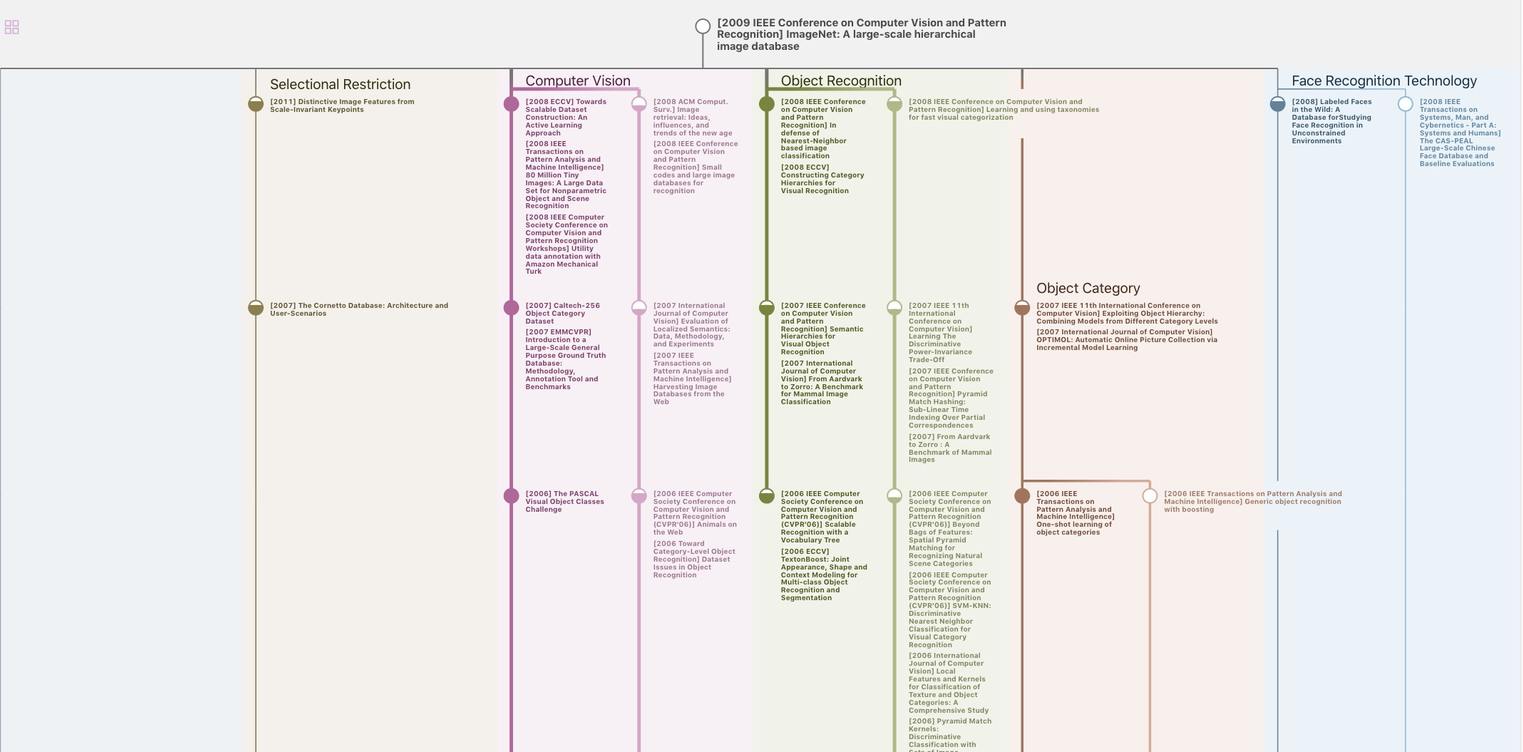
生成溯源树,研究论文发展脉络
Chat Paper
正在生成论文摘要