A comparative simulation of normalization methods for machine learning-based intrusion detection systems using KDD Cup ' 99 dataset
JOURNAL OF INTELLIGENT & FUZZY SYSTEMS(2022)
摘要
Network Intrusion detection systems (NIDS) detect malicious and intrusive information in computer networks. Presently, commercial NIDS is based on machine learning approaches that have complex algorithms and increase intrusion detection efficiency and efficacy. These machine learning-based NIDS use high dimensional network traffic data from which intrusive information is to be detected. This high-dimensional network traffic data in NIDS needs to be preprocessed and normalized to make it suitable for machine learning tools. A machine learning approach with appropriate normalization and prepossessing increases NIDS performance. This paper presents an empirical study on various normalization methods implemented on a benchmark network traffic dataset, KDD Cup'99, that has been used to evaluate the NIDS model. The present study shows decimal normalization has a better prediction performance than non-normalized traffic data categorized into 'normal' or 'intrusive' classes.
更多查看译文
关键词
Intrusion detection system, machine learning, normalization, classification, KDD cup ' 99 dataset
AI 理解论文
溯源树
样例
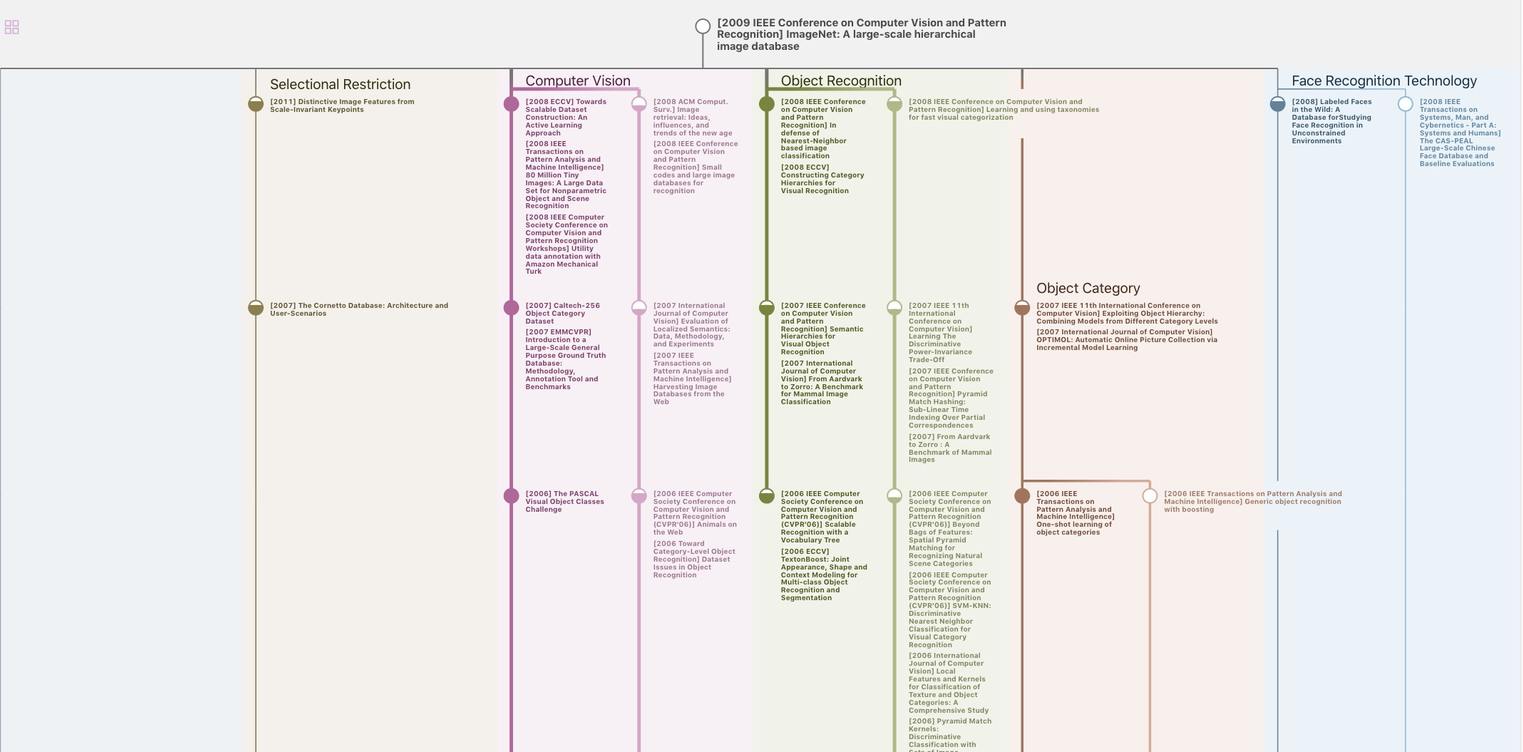
生成溯源树,研究论文发展脉络
Chat Paper
正在生成论文摘要