Deep image clustering by fusing contrastive learning and neighbor relation mining
Knowledge-Based Systems(2022)
摘要
Contrastive learning is widely used in deep image clustering due to its ability to learn discriminative representations. However, some studies simply combined contrastive learning with clustering. This line of works often ignores semantic meaningful representations and leads to suboptimal performance. In this paper, we propose a new deep image clustering framework called Nearest Neighbor Contrastive Clustering (NNCC), which fuses contrastive learning with neighbor relation mining. During training, contrastive learning and neighbor relation mining are updated alternately, where the former is conducted in the backward pass, while the latter is employed in the forward pass. Specially, we empirically find that data augmentation is an effective technique for generating nearest neighbors manually. A stronger data augmentation means more nearest neighbors involved for learning powerful discriminative representations in the contrastive learning. Due to effective neighbor relation mining, the proposed framework learns more semantic meaningful representations with contrastive learning and obtains more accurate image clusters. Through experimental results on six image datasets, the proposed framework defeats compared state-of-the-arts clustering methods.
更多查看译文
关键词
Unsupervised learning,Representation learning,Image clustering,Contrastive learning,Nearest neighbors
AI 理解论文
溯源树
样例
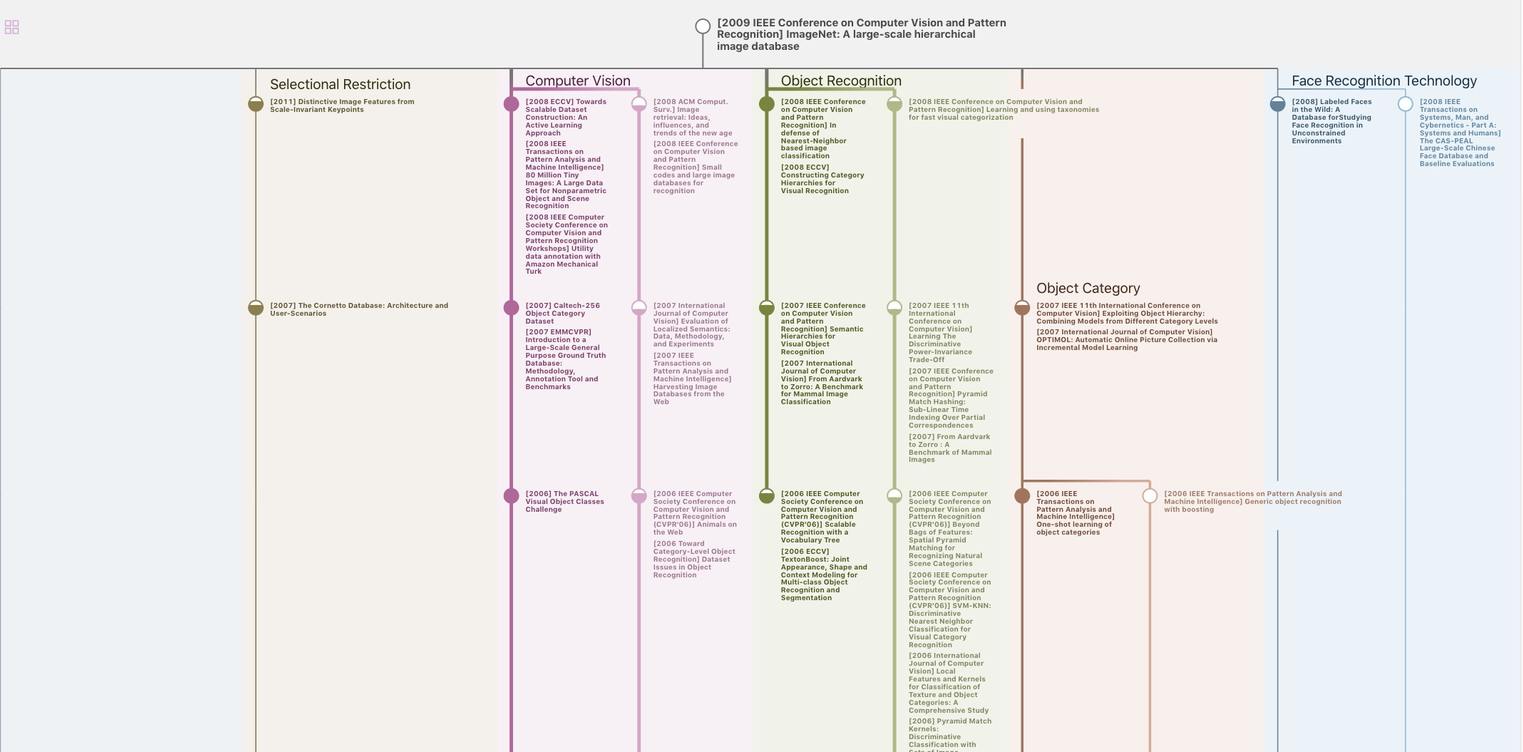
生成溯源树,研究论文发展脉络
Chat Paper
正在生成论文摘要