Spherical Zero-Shot Learning
IEEE Transactions on Circuits and Systems for Video Technology(2022)
摘要
Zero-shot Learning (ZSL) is a highly non-trivial task to generalize from seen to unseen classes. In this paper, we propose spherical zero-shot learning (SZSL) to address the major challenges in ZSL. By decoupling the similarity metric in the spherical embedding space into radius and angle, our SZSL can map classes to hyperspherical surfaces of different radiuses, which greatly increases its flexibility. Specifically, we introduce the spherical alignment on angles to spread classes as uniformly as possible to alleviate the hubness problem and simultaneously preserve the inter-class semantic structure to make the alignment more reasonable. We also introduce the spherical calibration with a minimum entropy based regularizer by adopting a larger radius for unseen classes than seen classes to reduce the prediction bias. Extensive experiments on five middle-scale benchmarks and large-scale ImageNet dataset demonstrate that the proposed approach consistently achieves superior performance for the traditional and generalized settings of ZSL.
更多查看译文
关键词
Zero-shot learning,decoupled metric,hubness problem,prediction bias
AI 理解论文
溯源树
样例
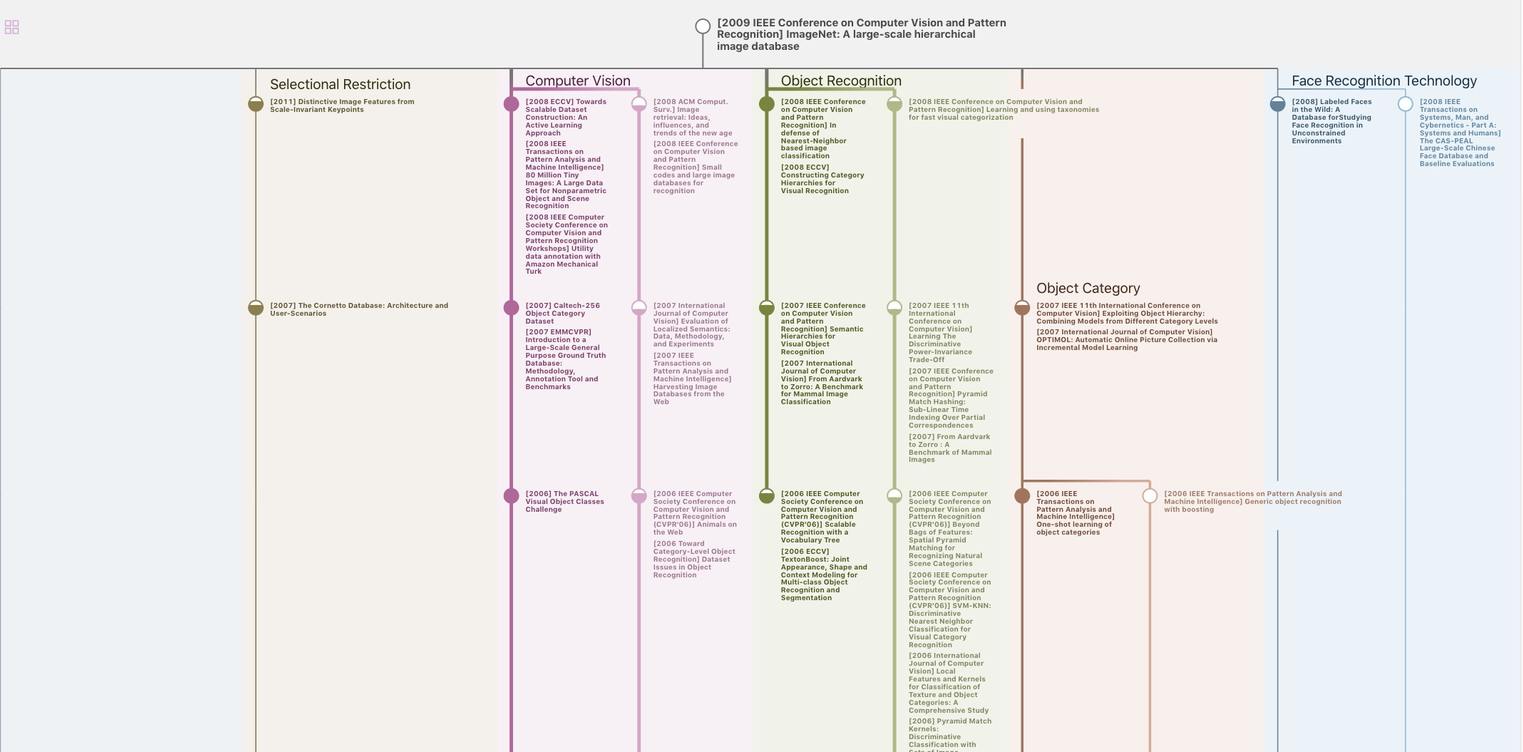
生成溯源树,研究论文发展脉络
Chat Paper
正在生成论文摘要