Rethinking explainability: toward a postphenomenology of black-box artificial intelligence in medicine
ETHICS AND INFORMATION TECHNOLOGY(2022)
摘要
In recent years, increasingly advanced artificial intelligence (AI), and in particular machine learning, has shown great promise as a tool in various healthcare contexts. Yet as machine learning in medicine has become more useful and more widely adopted, concerns have arisen about the “black-box” nature of some of these AI models, or the inability to understand—and explain—the inner workings of the technology. Some critics argue that AI algorithms must be explainable to be responsibly used in the clinical encounter, while supporters of AI dismiss the importance of explainability and instead highlight the many benefits the application of this technology could have for medicine. However, this dichotomy fails to consider the particular ways in which machine learning technologies mediate relations in the clinical encounter, and in doing so, makes explainability more of a problem than it actually is. We argue that postphenomenology is a highly useful theoretical lens through which to examine black-box AI, because it helps us better understand the particular mediating effects this type of technology brings to clinical encounters and moves beyond the explainability stalemate. Using a postphenomenological approach, we argue that explainability is more of a concern for physicians than it is for patients, and that a lack of explainability does not introduce a novel concern to the physician–patient encounter. Explainability is just one feature of technological mediation and need not be the central concern on which the use of black-box AI hinges.
更多查看译文
关键词
Artificial intelligence, Machine learning, Black-box, Postphenomenology, Technological mediation, Ethics
AI 理解论文
溯源树
样例
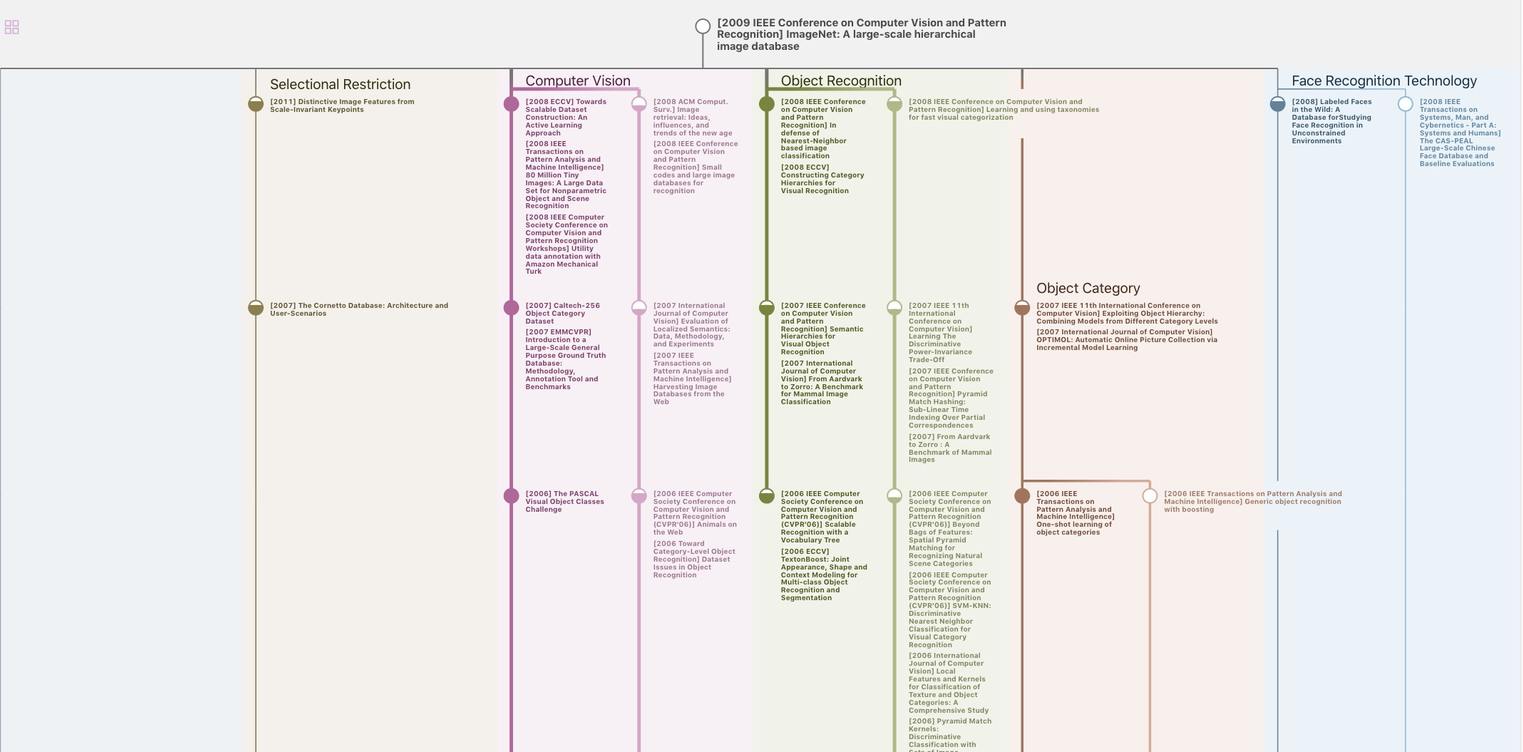
生成溯源树,研究论文发展脉络
Chat Paper
正在生成论文摘要