A Machine Learning Driven Pipeline for Automated Photoplethysmogram Signal Artifact Detection
2021 IEEE/ACM Conference on Connected Health: Applications, Systems and Engineering Technologies (CHASE)(2021)
摘要
Recent advances in Critical Congenital Heart Disease (CCHD) research using Photoplethysmography (PPG) signals have yielded an Internet of Things (IoT) based enhanced screening method that performs CCHD detection comparable to SpO2 screening. The use of PPG signals, however, poses a challenge due to its measurements being prone to artifacts. To comprehensively study the most effective way to remove the artifact segments from PPG waveforms, we performed feature engineering and investigated both Machine Learning (ML) and rule based algorithms to identify the optimal method of artifact detection. Our proposed artifact detection system utilizes a 3-stage ML model that incorporates both Gradient Boosting (GB) and Random Forest (RF). The proposed system achieved 84.01% of Intersection over Union (IoU), which is competitive to state-of-the-art artifact detection methods tested on higher resolution PPG.
更多查看译文
关键词
PPG,CCHD,artifacts,Machine Learning
AI 理解论文
溯源树
样例
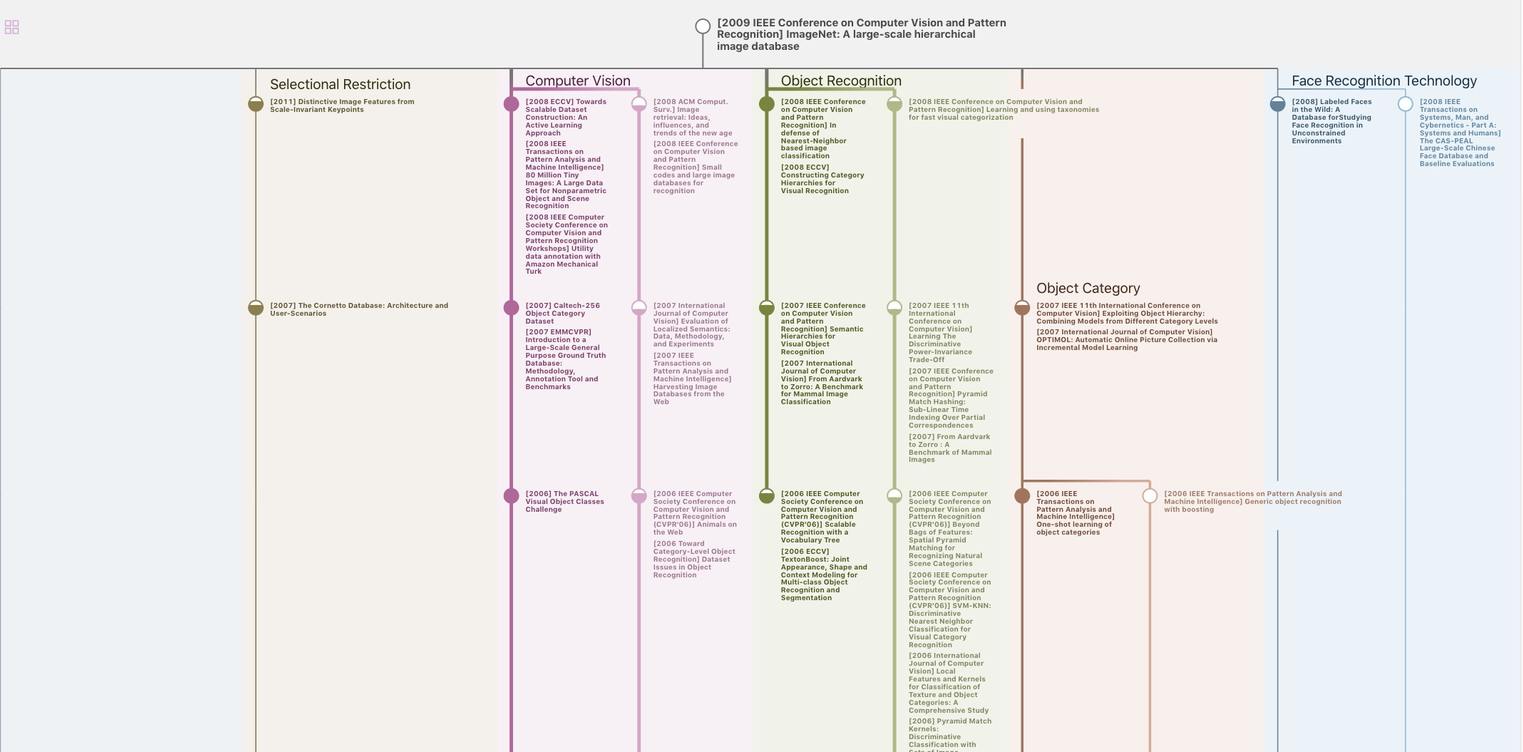
生成溯源树,研究论文发展脉络
Chat Paper
正在生成论文摘要