Modeling Scale-free Graphs with Hyperbolic Geometry for Knowledge-aware Recommendation
WSDM(2022)
摘要
ABSTRACTAiming to alleviate data sparsity and cold-start problems of tradi- tional recommender systems, incorporating knowledge graphs (KGs) to supplement auxiliary information has recently gained considerable attention. Via unifying the KG with user-item interactions into a tripartite graph, recent works explore the graph topologies to learn the low-dimensional representations of users and items with rich semantics. These real-world tripartite graphs are usually scale-free, however, the intrinsic hierarchical graph structures of which are underemphasized in existing works, consequently, leading to suboptimal recommendation performance. To address this issue and provide more accurate recommendation, we propose a knowledge-aware recommendation method with Lorentz model of the hyperbolic geometry, namely Lorentzian Knowledge-enhanced Graph convolutional networks for Recommendation (LKGR). LKGR facilitates better modeling of scale-free tripartite graphs after the data unification. Specifically, we employ different information propagation strategies in the hyperbolic space to explicitly encode heterogeneous information from historical interactions and KGs. Additionally, our proposed knowledge-aware attention mechanism enables the model to automatically measure the information contribution, producing the coherent information aggregation in the hyperbolic space. Extensive experiments on three real-world benchmarks demonstrate that LKGR outperforms state-of-the-art methods by 3.6-15.3% of [email protected] on Top-K recommendation.
更多查看译文
关键词
Recommender system, Knowledge graph, Hyperbolic geometric
AI 理解论文
溯源树
样例
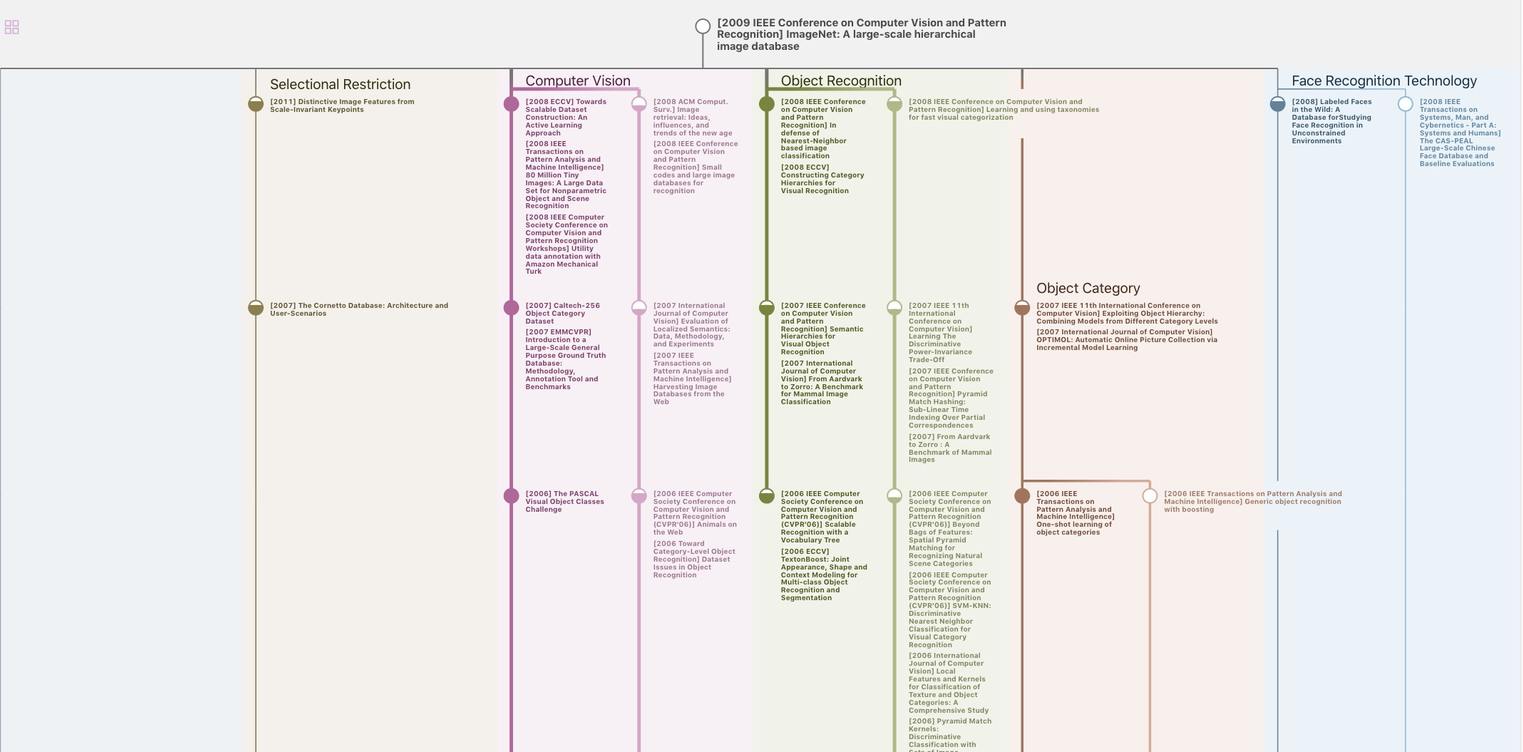
生成溯源树,研究论文发展脉络
Chat Paper
正在生成论文摘要