ESC-GAN: Extending Spatial Coverage of Physical Sensors
WSDM(2022)
摘要
ABSTRACTScientific discoveries and studies about our physical world have long benefited from large-scale and planetary sensing, from weather forecasting to wildfire monitoring. However, the limited deployment of sensors in the environment due to cost or physical access constraints has lagged behind our ever-growing need for increased data coverage and higher resolution, impeding timely and precise monitoring and understanding of the environment. Therefore, we seek to extend the spatial coverage of analysis based on existing sensory data, that is, to "generate" data for locations where no historical data exists. This problem is fundamentally different and more challenging than the traditional spatio-temporal imputation that assumes data for any particular location are only partially missing across time. Inspired by the success of Generative Adversarial Network (GAN) in imputation, we propose a novel ESC-GAN. We observe that there are local patterns in nearby locations, as well as trends in a global manner (e.g., temperature drops as altitude increases regardless of the location). As local patterns may exhibit at different scales (from meters to kilometers), we employ a multi-branch generator to aggregate information of different granularity. More specifically, each branch in the generator contains 1) randomly masked 3D partial convolutions at different resolutions to capture the local patterns and 2) global attention modules for global similarity. Next, we adversarially train a 3D convolution-based discriminator to distinguish the generator's output from the ground truth. Extensive experiments on three geo-sensor datasets demonstrate that ESC-GAN outperforms state-of-the-art methods on extending spatial coverage and also achieves the best results on a traditional spatio-temporal imputation task.
更多查看译文
关键词
Spatio-temporal data, imputation, super resolution, self-attention, generative adversarial network
AI 理解论文
溯源树
样例
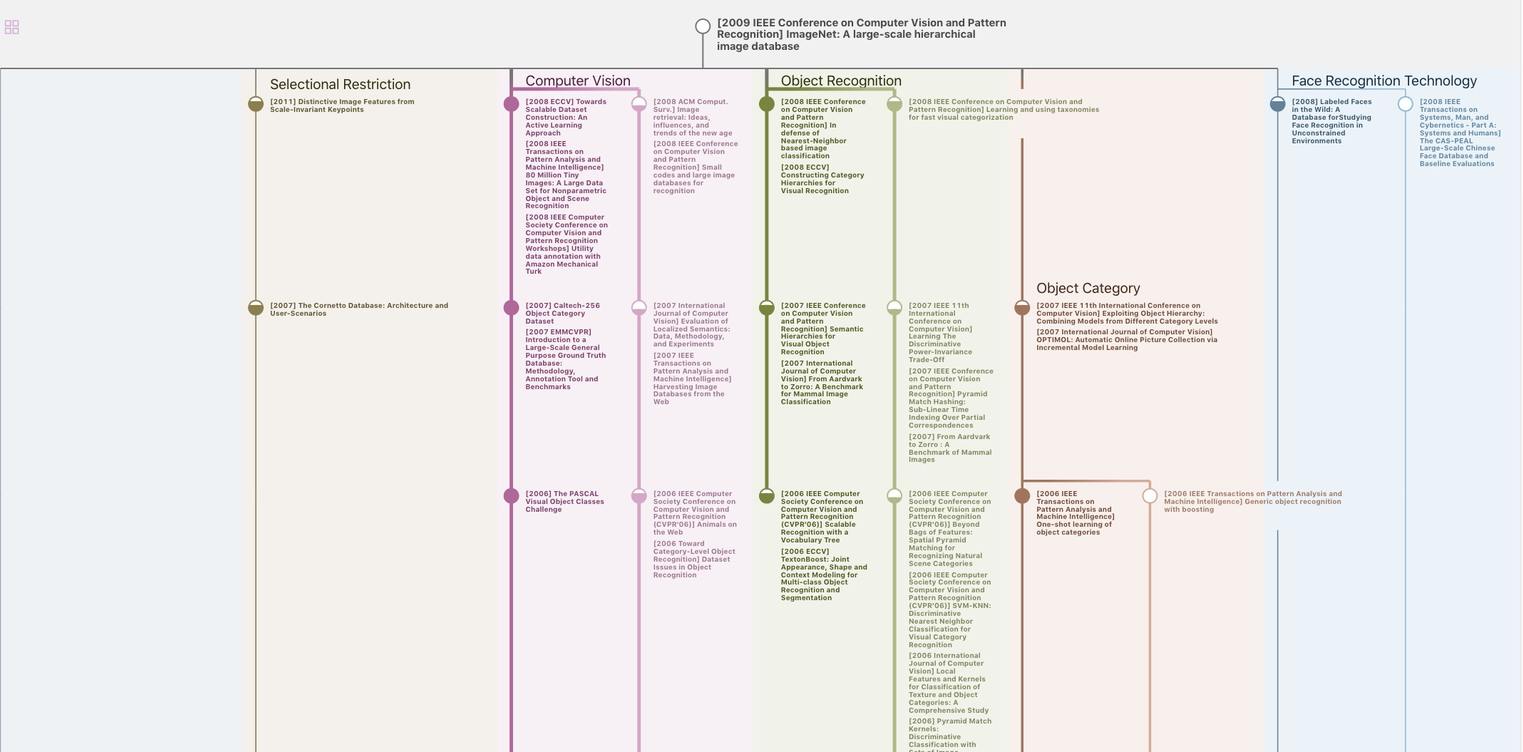
生成溯源树,研究论文发展脉络
Chat Paper
正在生成论文摘要