Performance Analysis of XGBoost Models with Ultrafast Shape Recognition Descriptors in Ligand-Based Virtual Screening.
ICBRA(2021)
摘要
Ligand-Based Virtual Screening (LBVS) is a powerful computational approach to develop drug discovery studies when at least an active ligand from a target molecule is known. In these studies, different metrics are used to measure the similarity of physicochemical properties of the actives and query molecules. However, as the metric selected directly affects the performance of the study, machine learning techniques have shown to be a good alternative to replace and compute these similarity measurements. In this context, we developed an XGBoost Model to perform similarity measurements as a new alternative in LBVS studies. For this purpose, we used a diverse dataset from the Directory of Useful Decoys-Enhanced (DUD-E) and applied the Ultrafast Shape Recognition (USR) and USR with CREDO atom types (USRCAT) methods as a baseline to compare the performance of the XGBoost model in terms of the Enrichment Factor (EF) and Area Under the Curve (AUC) metrics. Moreover, to compare the performance of XGBoost over other machine learning techniques, an Artificial Neural Network (ANN) model was implemented. Results from both machine learning models shows a good improvement achieving more than twice the EF of traditional USR methods. However, the XGBoost Model has the highest performance over ANN's with both USR and USRCAT descriptors.
更多查看译文
关键词
Drug discovery, machine learning, extreme gradient boosting, virtual screening
AI 理解论文
溯源树
样例
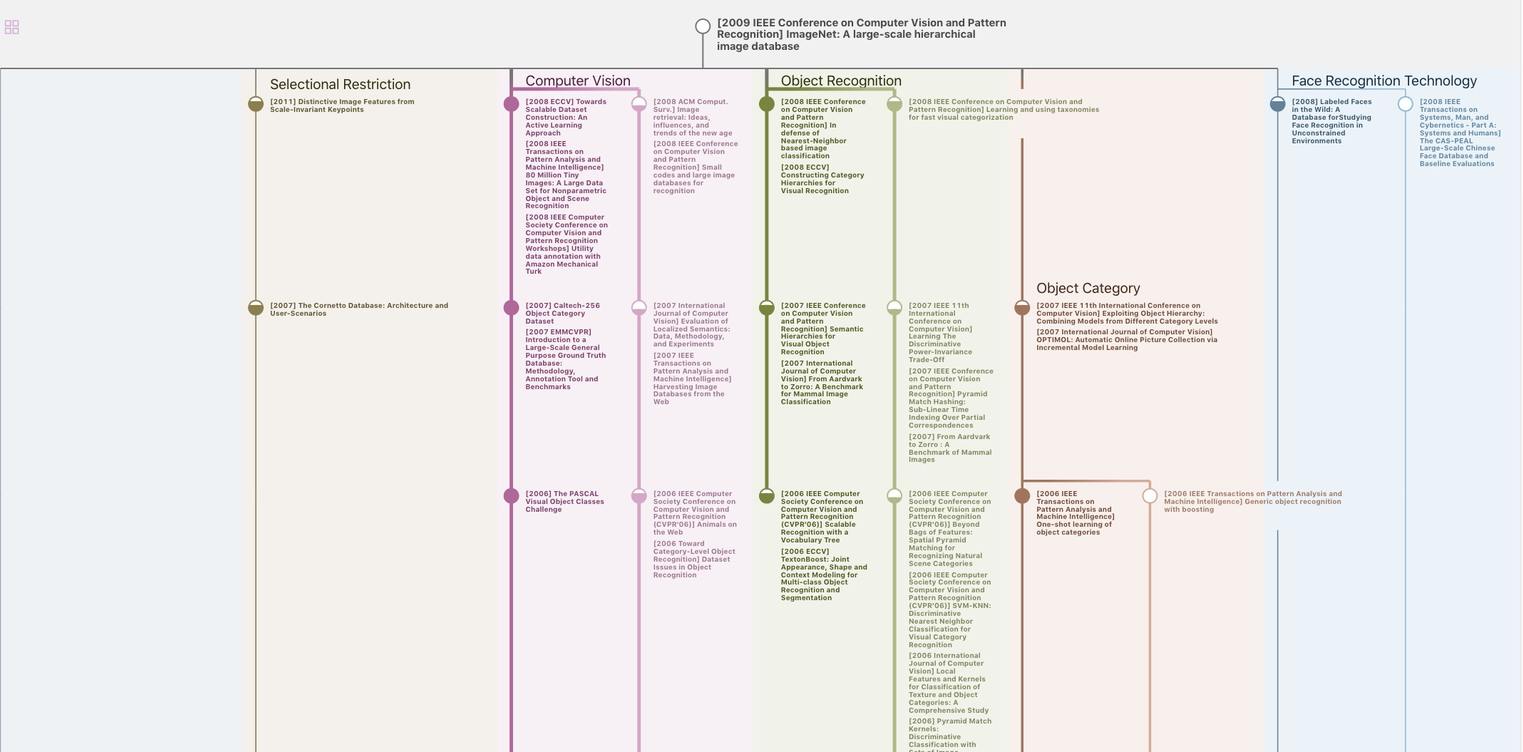
生成溯源树,研究论文发展脉络
Chat Paper
正在生成论文摘要