On Attacks To Federated Learning and a Blockchain-empowered Protection.
CCNC(2022)
摘要
Federated learning has been increasingly studied to cope with the scalability and privacy issues characterizing current and upcoming large-scale infrastructures, such as the Internet of Things, 5G networks, vehicular applications, and so on. This approach partitions the data storage and AI model training in a series of local learners, whose results are aggregated by a central server and then redistributed back to the learners to have a trained global model. However, despite avoiding outsourcing sensitive data to cloud-hosted services and fragmenting the workload for data processing, such a decentralized learning approach lays the overall solution open to various kinds of attacks, able to fully compromise the accuracy of the obtained global model. This study aims to quantitatively assess the impact of two widely-recognized attacks against federated learning and propose a tentative protection means by using blockchain.
更多查看译文
关键词
federated learning,blockchain-empowered protection,data storage,outsourcing sensitive data,data processing,decentralized learning,AI model training,cloud-hosted services
AI 理解论文
溯源树
样例
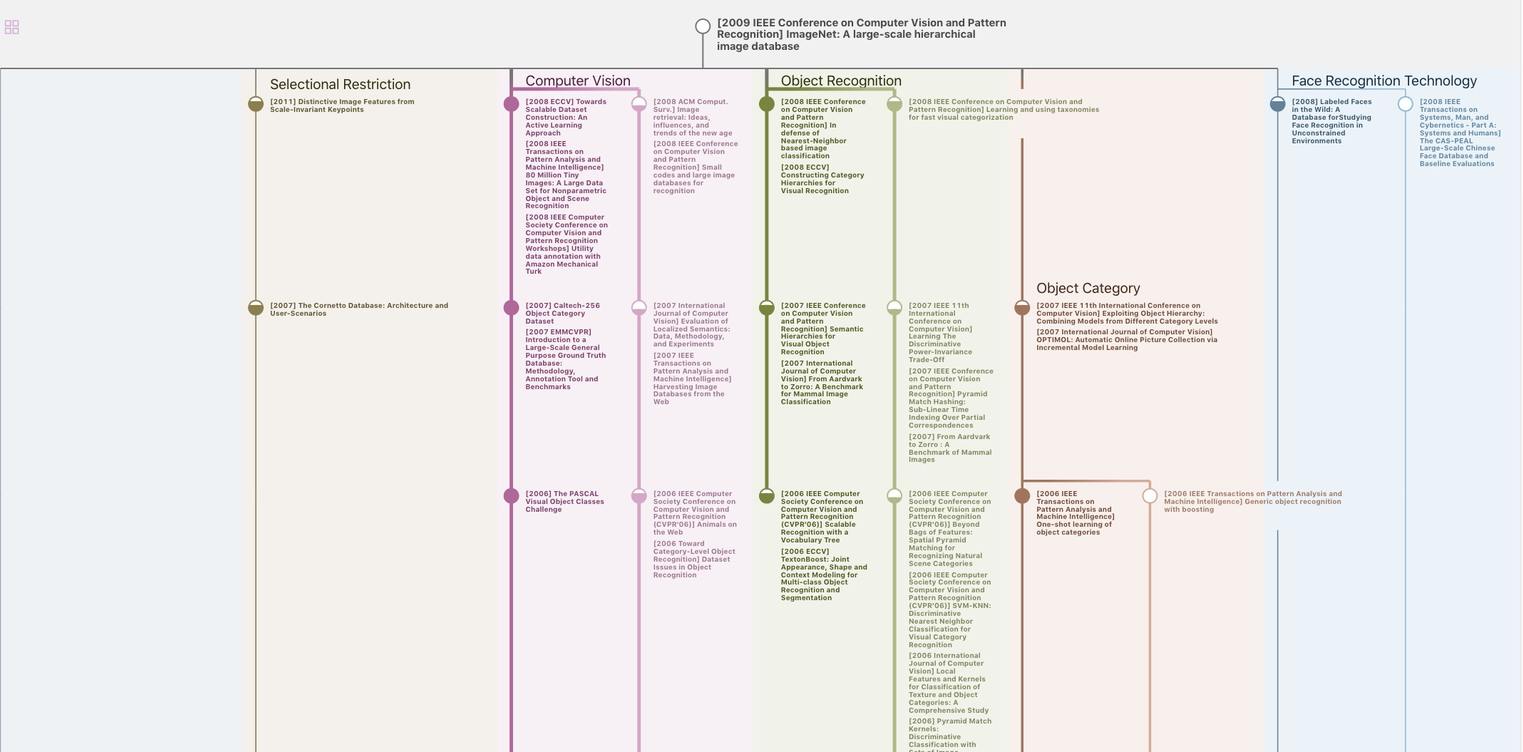
生成溯源树,研究论文发展脉络
Chat Paper
正在生成论文摘要