Multi-factor Behavioral Authentication Using Correlations Enhanced by Neural Network-based Score Fusion
Consumer Communications and Networking Conference(2022)
摘要
In recent years, personal behavioral authentication has been proposed as a new authentication method to support traditional knowledge-based, possession-based, and biometrics-based authentication. Most of the previous behavioral authentication research relied on historical behavioral patterns or trained classification models, thus requiring a large amount of preliminary data. In addition, while many studies utilized multiple authentication factors, authentication scores of each element were calculated independently without using correlations between them. This paper proposes a new authentication approach that uses behavioral correlations between each factor. In order to demonstrate the effectiveness of our proposal, we constructed a correlation-based authentication method using the data collected from smartphones and wearable activity trackers, including GPS locations, Wi-Fi access points, and activity types inferred from the metabolic equivalent of task (MET). Since the proposed method matches the data from multiple sensors to verify the request rather than utilizing the pattern extracted from the historical data, it does not require a large amount of preliminary data. We also employed a neural network-based score fusion method that aggregates the three authentication scores to improve the final authentication accuracy. The experimental result showed that the proposed method could achieve a half total error rate (HTER, an arithmetic average of a false rejection rate and false acceptance rate) of merely 8.0% that is much lower than other classification methods using the same dataset.
更多查看译文
关键词
behavioral authentication,multi-factor authentication,activity tracker,score fusion,neural network
AI 理解论文
溯源树
样例
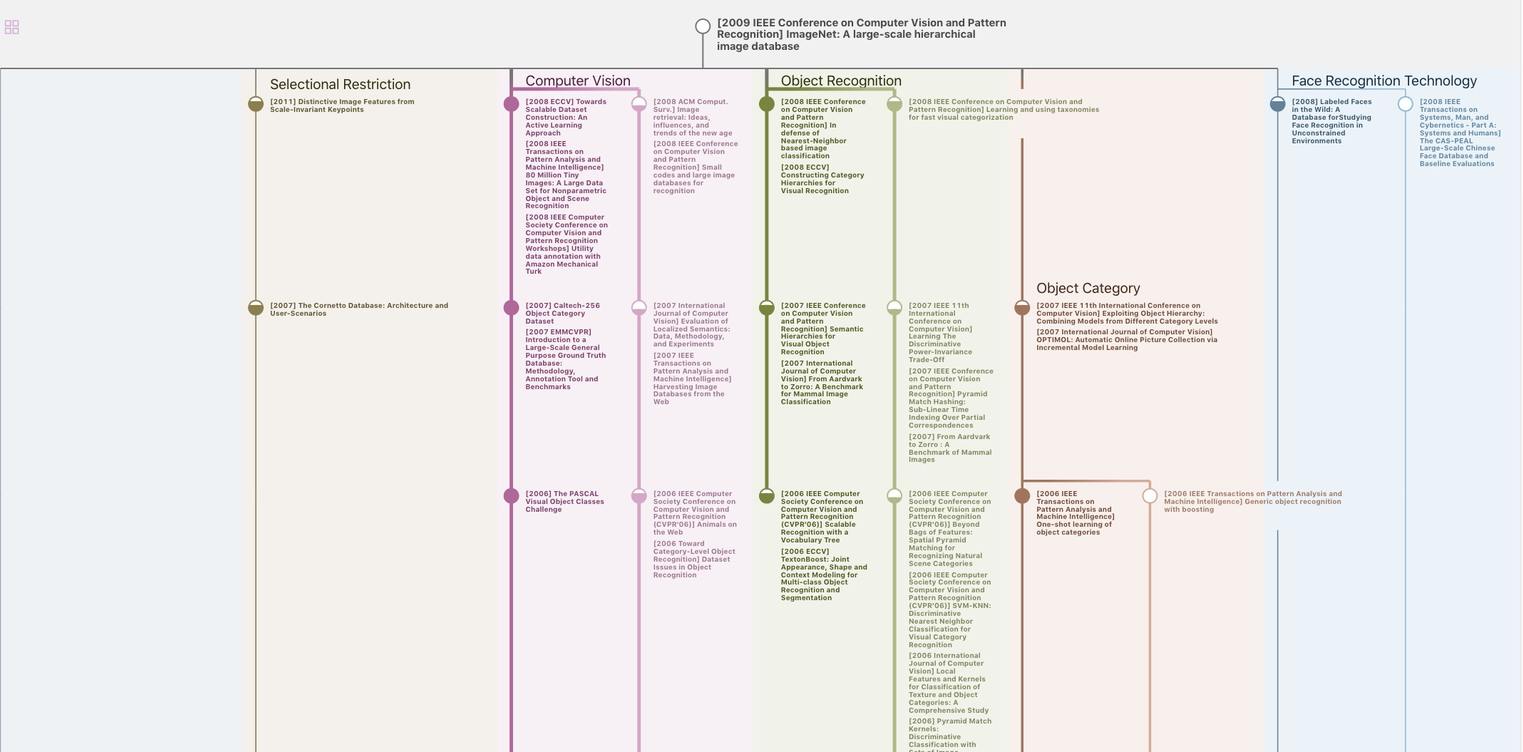
生成溯源树,研究论文发展脉络
Chat Paper
正在生成论文摘要