Few-shot Steel Surface Defect Detection Based on Meta Learning.
ICCPR(2021)
摘要
The performance of the deep learning-based object detection algorithms mainly relies on the large-scale image sets. However, it is extremely difficult to collect the defect samples in the practical steel production. Few-shot object detection provides an ideal solution for the insufficient training sets. However, the efficient feature extractor is crucial for the few-shot object detection methods. In this paper, a novel few-shot defect detection method on Steel Surface is proposed based on the meta-learning technology. The detection model includes three main components: a meta feature learner, a feature matching module and a bounding box prediction module. The meta features are extracted from the base classes with large-scale sets using the meta feature learner, and it can be generalized to detect the novel steel surface defects. The feature matching module adopts a few support images from the base classes and the novel class to generalize a global feature vector which can be used to adjust the weights of meta features maps for detecting the corresponding defects. The bounding box prediction module is designed to conduct the defect detection on the adjusted feature maps. Experiments on the steel surface defect dataset demonstrate that our proposed method can efficiently localize the defects with only a few annotated samples, and outperform the well-established baseline models.
更多查看译文
AI 理解论文
溯源树
样例
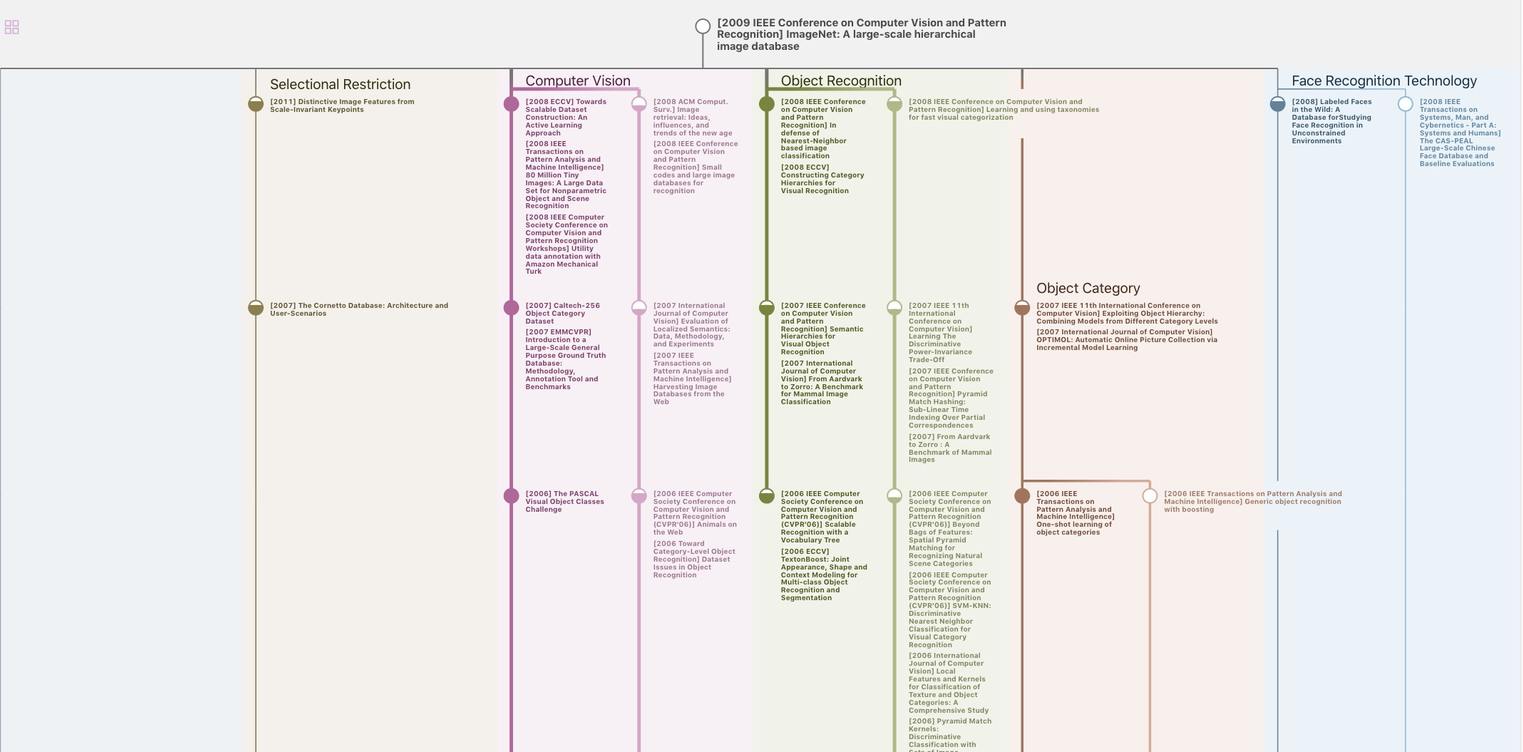
生成溯源树,研究论文发展脉络
Chat Paper
正在生成论文摘要