Rethinking Video Anomaly Detection - A Continual Learning Approach
2022 IEEE WINTER CONFERENCE ON APPLICATIONS OF COMPUTER VISION (WACV 2022)(2022)
摘要
While video anomaly detection has been an active area of research for several years, recent progress is limited to improving the state-of-the-art results on small datasets using an inadequate evaluation criterion. In this work, we take a new comprehensive look at the video anomaly detection problem from a more realistic perspective. Specifically, we consider practical challenges such as continual learning and few-shot learning, which humans can easily do but remains to be a significant challenge for machines. A novel algorithm designed for such practical challenges is also proposed. For performance evaluation in this new framework, we introduce a new dataset which is significantly more comprehensive than the existing benchmark datasets, and a new performance metric which takes into account the fundamental temporal aspect of video anomaly detection. The experimental results show that the existing state-of-the-art methods are not suitable for the considered practical challenges, and the proposed algorithm outperforms them with a large margin in continual learning and few-shot learning tasks.
更多查看译文
关键词
Security/Surveillance Datasets,Evaluation and Comparison of Vision Algorithms,Scene Understanding
AI 理解论文
溯源树
样例
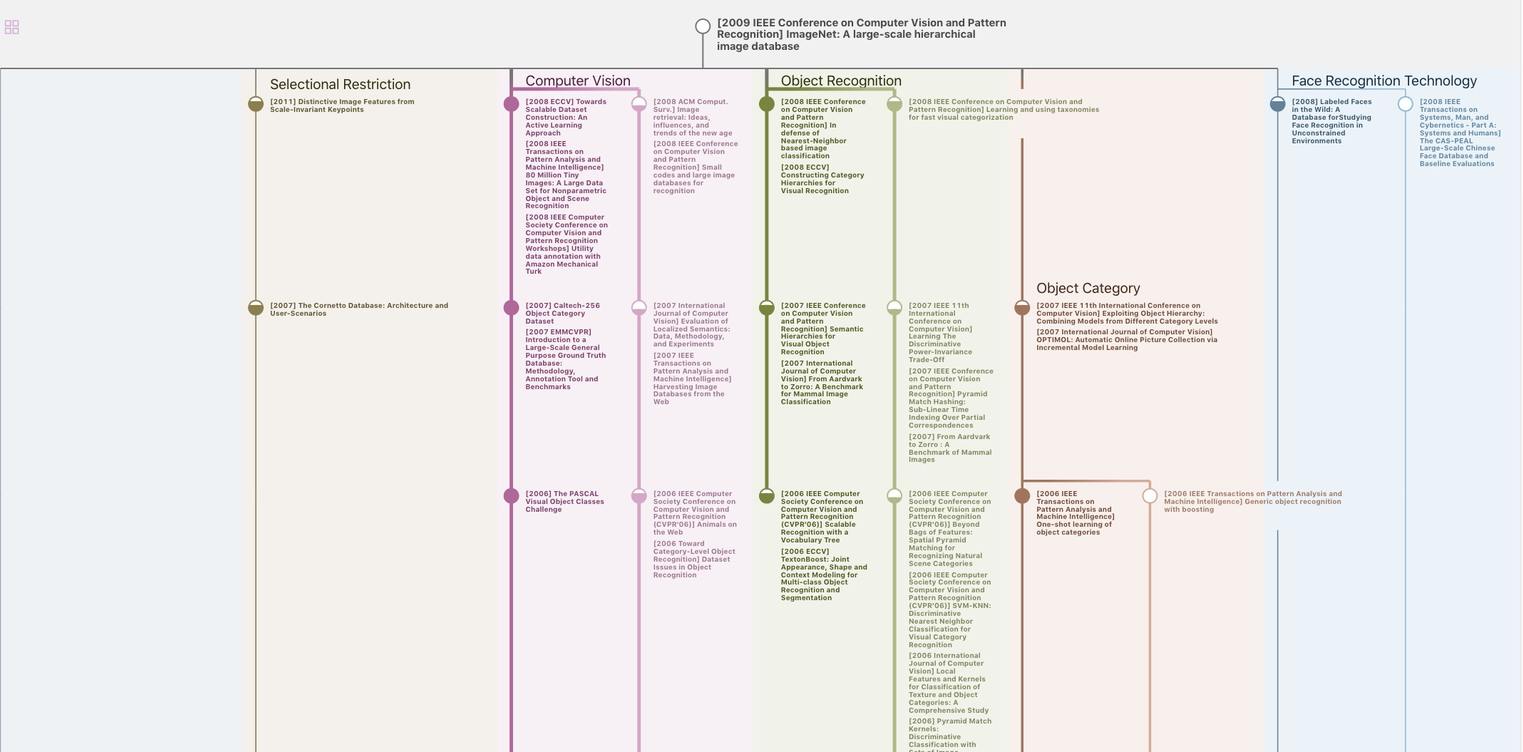
生成溯源树,研究论文发展脉络
Chat Paper
正在生成论文摘要