Less Can Be More: Sound Source Localization With a Classification Model
2022 IEEE/CVF Winter Conference on Applications of Computer Vision (WACV)(2022)
摘要
In this paper, we tackle sound localization as a natural outcome of the audio-visual video classification problem. Differently from the existing sound localization approaches, we do not use any explicit sub-modules or training mechanisms but use simple cross-modal attention on top of the representations learned by a classification loss. Our key contribution is to show that a simple audio-visual classification model has the ability to localize sound sources accurately and to give on par performance with state-of-the-art methods by proving that indeed "less is more". Furthermore, we propose potential applications that can be built based on our model. First, we introduce informative moment selection to enhance the localization task learning in the existing approaches compare to mid-frame usage. Then, we introduce a pseudo bounding box generation procedure that can significantly boost the performance of the existing methods in semi-supervised settings or be used for large-scale automatic annotation with minimal effort from any video dataset.
更多查看译文
关键词
Visual Reasoning, Analysis and Understanding Audio-Visual learning
AI 理解论文
溯源树
样例
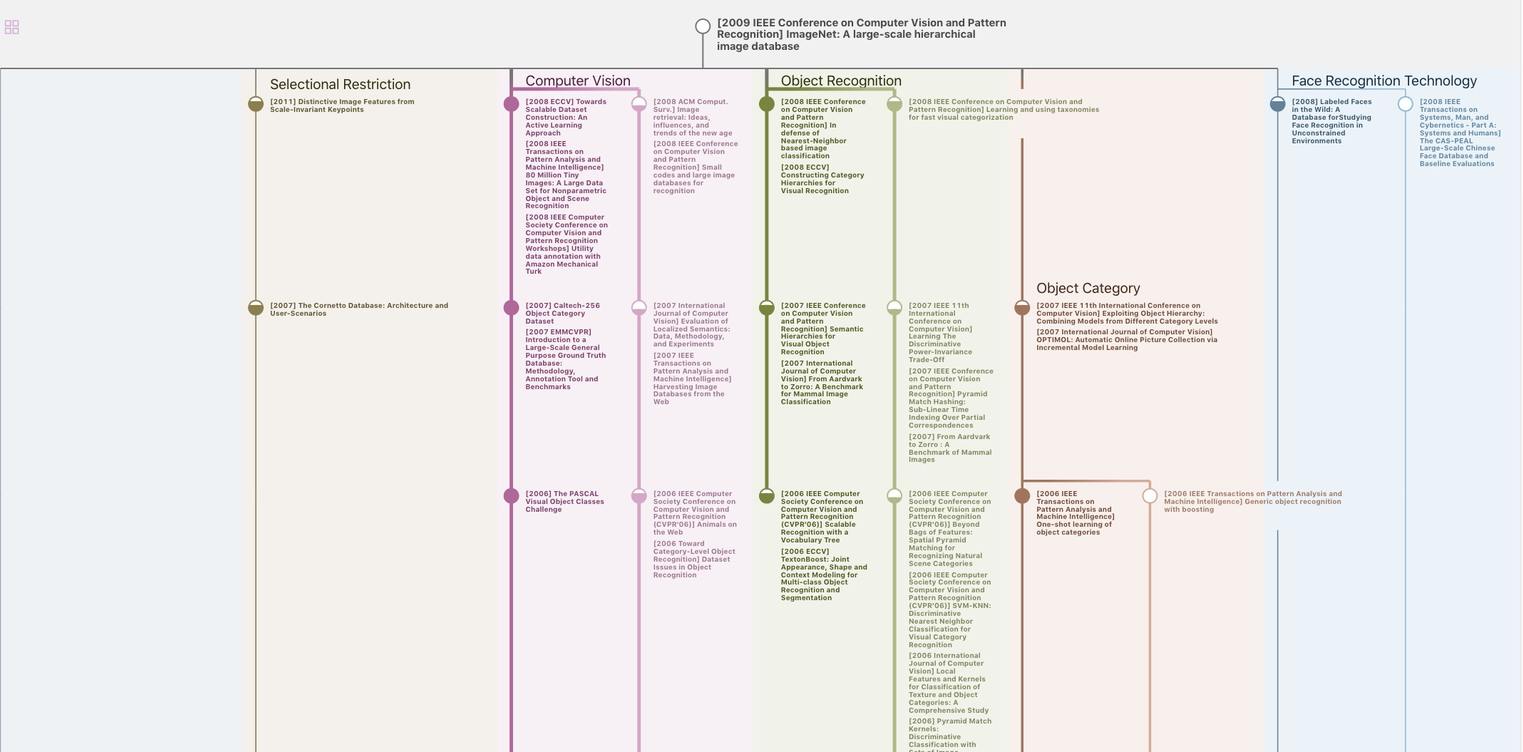
生成溯源树,研究论文发展脉络
Chat Paper
正在生成论文摘要