An essentially decentralized interior point method for control
2021 60TH IEEE CONFERENCE ON DECISION AND CONTROL (CDC)(2021)
摘要
Distributed and decentralized optimization are key for the control of networked systems. Application examples include distributed model predictive control and distributed sensing or estimation. Non-linear systems, however, lead to problems with non-convex constraints for which classical decentralized optimization algorithms lack convergence guarantees. Moreover, classical decentralized algorithms usually exhibit only linear convergence. This paper presents an essentially de-centralized primal-dual interior point method with convergence guarantees for non-convex problems at a superlinear rate. We show that the proposed method works reliably on a numerical example from power systems. Our results indicate that the proposed method outperforms ADMM in terms of computation time and computational complexity of the subproblems.
更多查看译文
关键词
decentralized optimization, non-convex optimization, interior point methods, optimal power flow
AI 理解论文
溯源树
样例
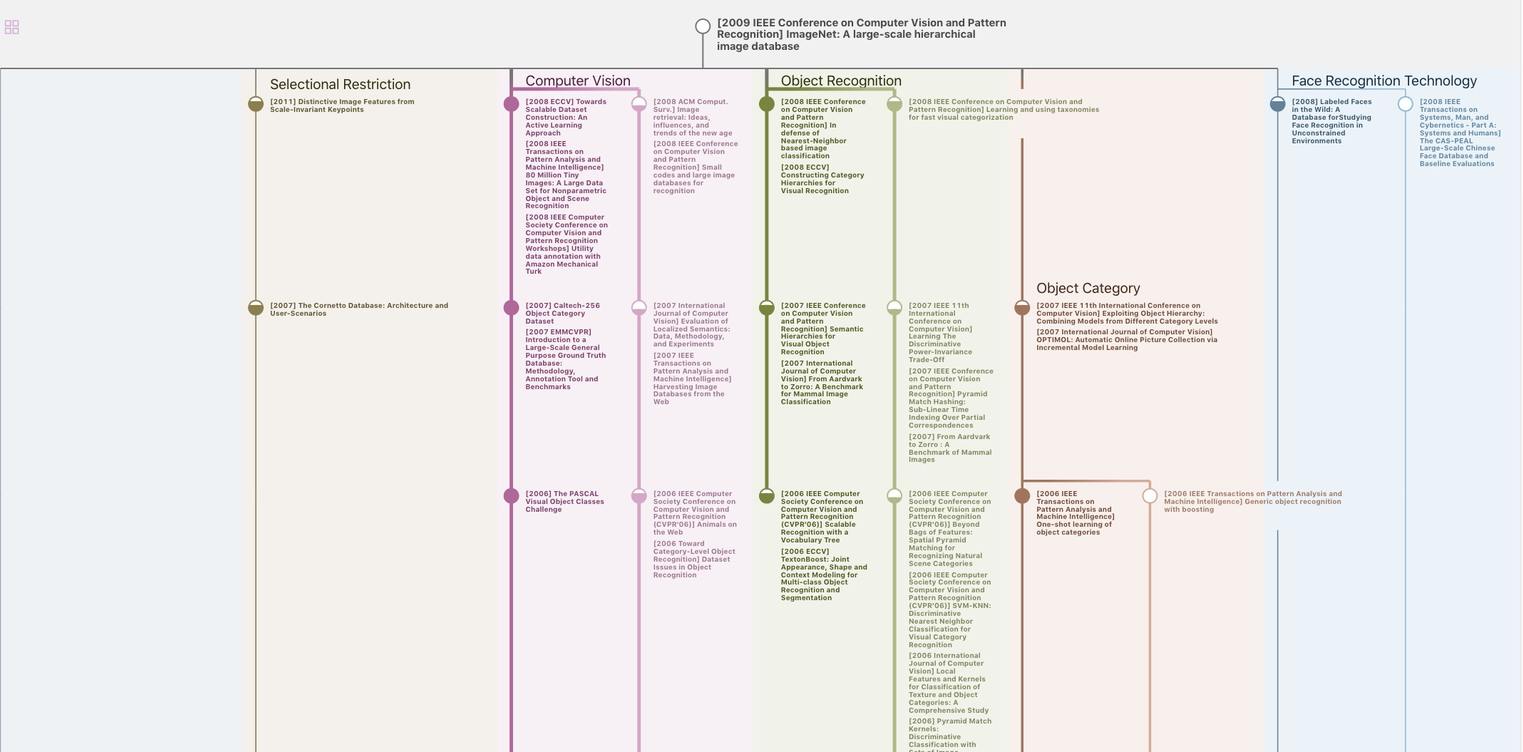
生成溯源树,研究论文发展脉络
Chat Paper
正在生成论文摘要