Reinforcement Learning Policies With Local LQR Guarantees For Nonlinear Discrete-Time Systems
CDC(2021)
摘要
Optimal control of nonlinear systems is a difficult problem which has been addressed by both the Control Theory (CT) and Reinforcement Learning (RL) communities. Frequently, the former relies on the linearization of the system thus obtaining only local guarantees. The latter relies on data to build model-free controllers, focused solely on performances. In this paper we propose a methodology to combine the advantages of both approaches, casting the formulation of an optimal local Linear Quadratic Regulator (LQR) into a Deep RL problem. Our solution builds on the linear framework to derive a learnt nonlinear controller showing local stability properties and global performances.
更多查看译文
关键词
local LQR guarantees,nonlinear discrete-time systems,optimal control,nonlinear systems,Control Theory,CT,Reinforcement Learning communities,linearization,local guarantees,model-free controllers,optimal local Linear Quadratic Regulator,Deep RL problem,linear framework,learnt nonlinear controller,local stability properties
AI 理解论文
溯源树
样例
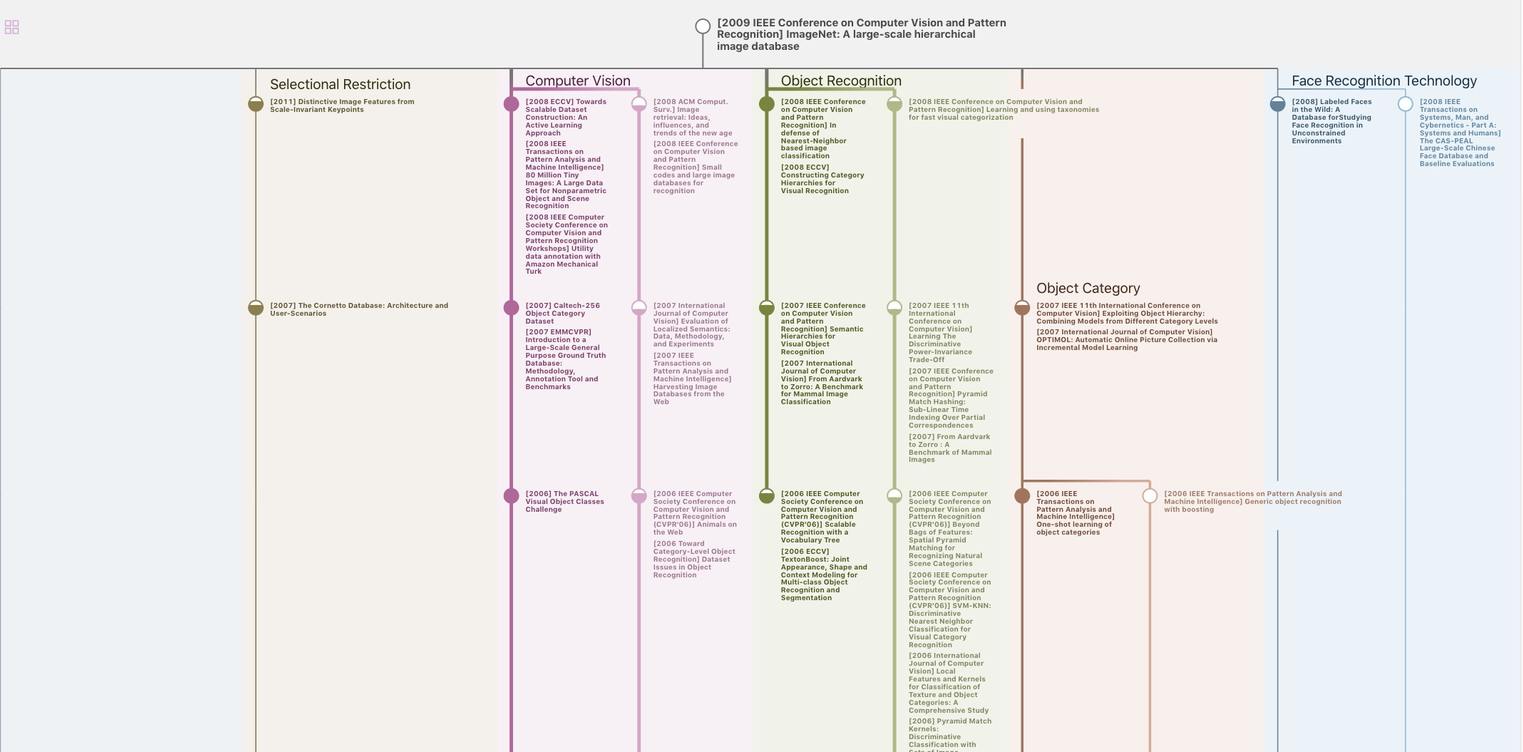
生成溯源树,研究论文发展脉络
Chat Paper
正在生成论文摘要