Coprime Microphone Arrays for Estimating Speech Direction of Arrival Using Deep Learning
2021 ASIA-PACIFIC SIGNAL AND INFORMATION PROCESSING ASSOCIATION ANNUAL SUMMIT AND CONFERENCE (APSIPA ASC)(2021)
摘要
This paper investigates deep neural network (DNNs) applied to coprime microphone arrays (CPMAs) and semi-coprime microphone arrays (SCPMAs) for direction of arrival (DOA) estimation of speech signals. Existing research has shown that the coprime arrangement increases the operating frequency of conventional uniform linear arrays (ULAs) by interleaving two uniform sub-arrays with different spacing. The SCPMA extends this arrangement and further increases the operating frequency, above which interfering signals are largely amplified in the recording and lead to confusion with the desired source. As a result, both types of coprime geometries improve the beampattern, array gain and DOA estimation results compared to the ULA. However, large side lobes may still occur in the beampattern of the two coprime arrangements, resulting in degraded DOA estimates using conventional beamforming-based approaches in an adverse environment. The proposed approach alternatively utilises deep learning (DL) to estimate speech DOAs using coprime microphone arrays. Experimental results evaluating the accuracy under different levels of noise using the mean absolute error (MAE) and root mean square error (RMSE) of the DOA estimate indicate satisfactory performance of the proposed method.
更多查看译文
关键词
deep learning,deep neural network,speech signals,uniform linear arrays,uniform sub-arrays,coprime geometries,beampattern array gain,coprime arrangements,coprime microphone arrays,DOA estimate,speech direction of arrival estimation,semicoprime microphone arrays,SCPMA,beamforming-based approaches,mean absolute error,root mean square error
AI 理解论文
溯源树
样例
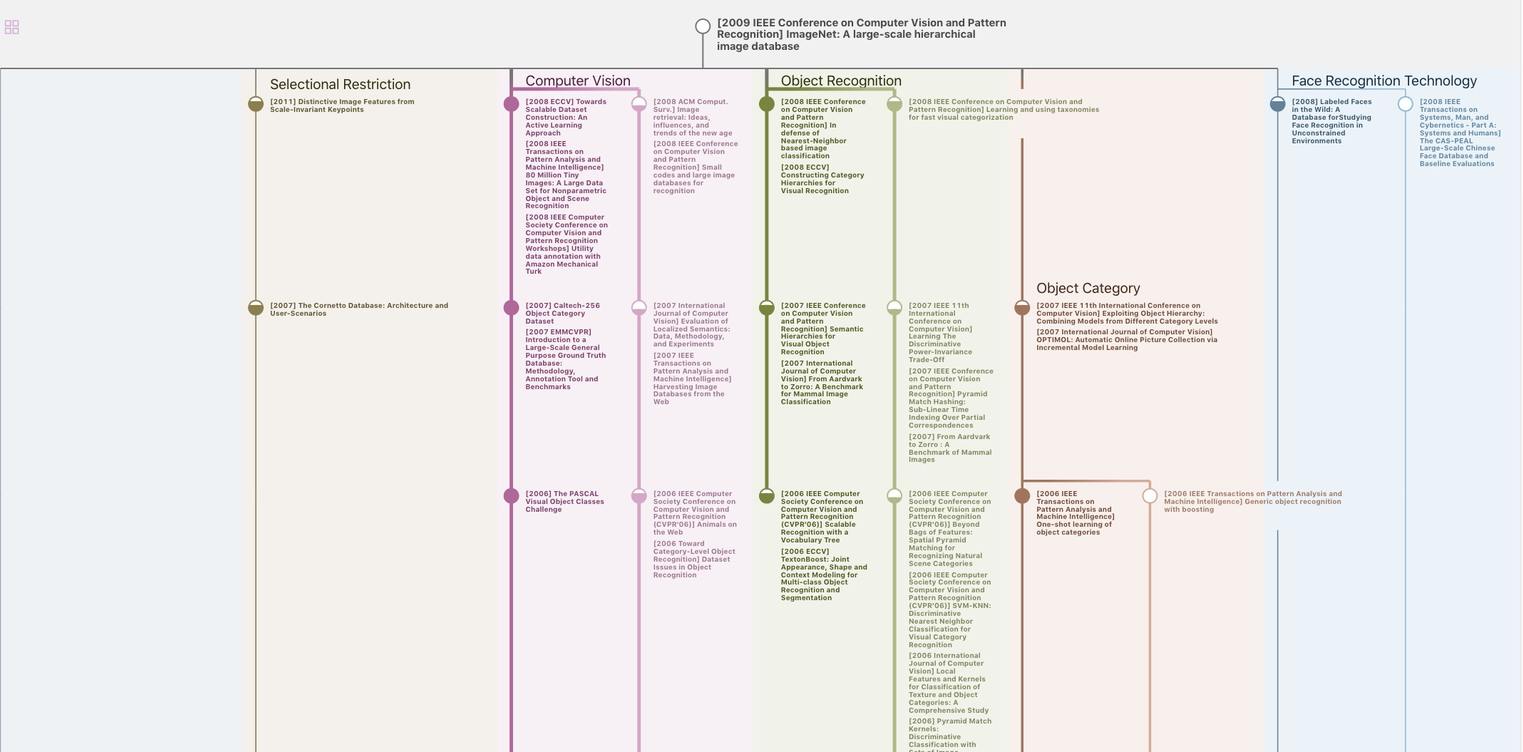
生成溯源树,研究论文发展脉络
Chat Paper
正在生成论文摘要