Neural Network Architecture for LLR Computation in 5G Systems and Related Business Aspects
2021 24th International Symposium on Wireless Personal Multimedia Communications (WPMC)(2021)
摘要
Log likelihood ratio (LLR) computations are an integral part of the communication decoder design. Soft decoding, the most accurate method of computing LLR, is computationally very expensive. Industries are thus using approximations that are designed keeping in mind the hardware complexity involved in the optimization process. In this paper, we propose a neural network based computation scheme that is trained to mimic the performance of soft decoding with high accuracy. Besides training, we further reduce the computational complexity by disabling the weak edges in the neural network and by approximating the activation function. The degree to which the weak connections are disabled varies according to the quality of service (QoS) and QoS class identifier (QCI) tables. Our scheme thus offers a three-fold benefit to a firm in terms of commercialization: the design of a near-optimal low-complex LLR computation scheme that performs well in a 5G setting that demand high speed and accuracy, implementability using state-of-the-art technologies, and the flexibility of design on the basis of the QoS requirements of the customer application.
更多查看译文
关键词
LLR,neural network,VRAN,5G
AI 理解论文
溯源树
样例
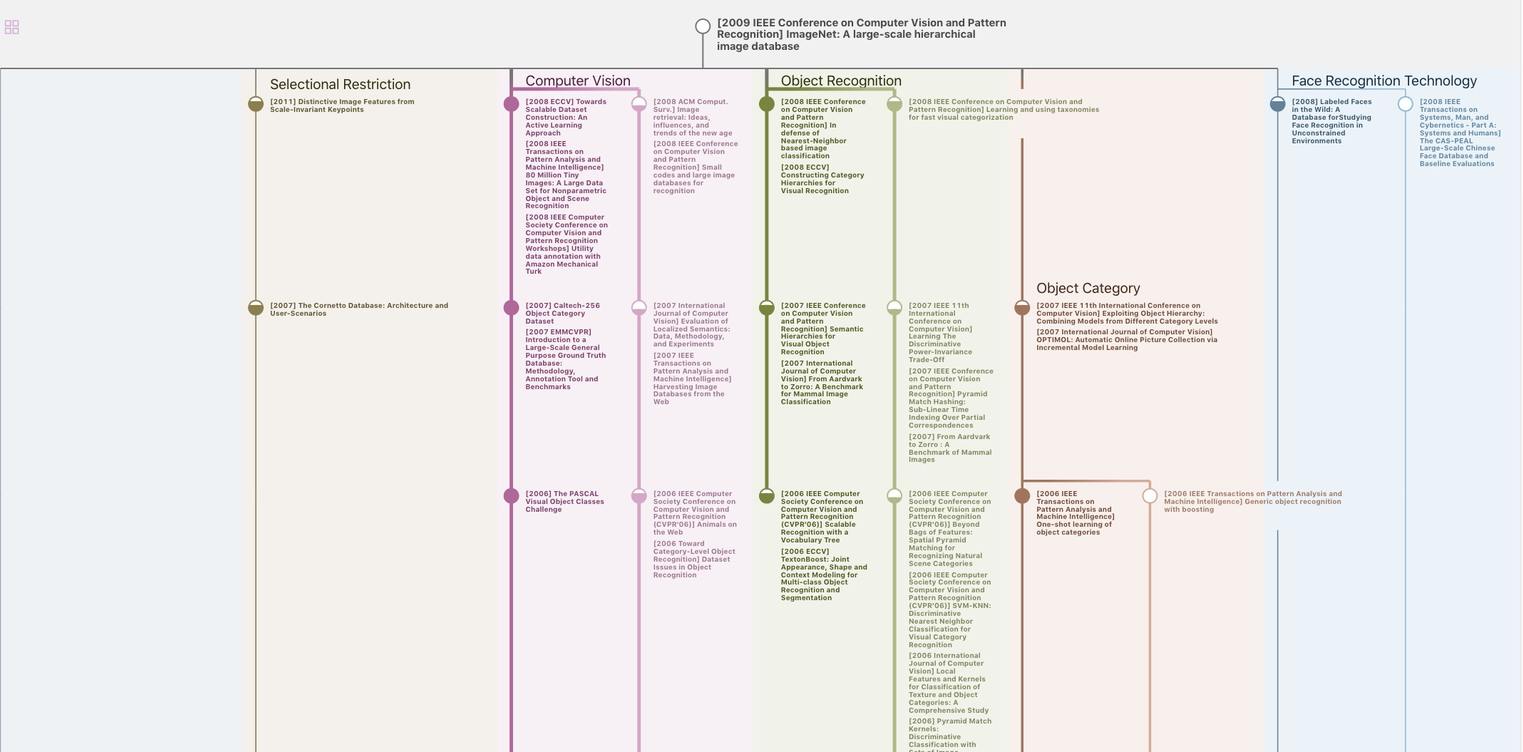
生成溯源树,研究论文发展脉络
Chat Paper
正在生成论文摘要