Discovering Antibiotic Efficacy Biomarkers
Molecular & Cellular Proteomics(2006)
摘要
As current antibiotic therapy is increasingly challenged by emerging drug-resistant bacteria, new technologies are required to identify and develop novel classes of antibiotics. A major bottleneck in today’s discovery efforts, however, is a lack of an efficient and standardized method for assaying the efficacy of a drug candidate. We propose a new high content screening approach for identifying efficacious molecules suitable for development of antibiotics. Key to our approach is a new microarray-based efficacy biomarker discovery strategy. We first produced a large dataset of transcriptional responses of Bacillus subtilis to numerous structurally diverse antibacterial drugs. Second we evaluated different protocols to optimize drug concentration and exposure time selection for profiling compounds of unknown mechanism. Finally we identified a surprisingly low number of gene transcripts (∼130) that were sufficient for identifying the mechanism of novel substances with reasonable accuracy (∼90%). We show that the statistics-based approach reveals a physiologically meaningful set of biomarkers that can be related to major bacterial defense mechanisms against antibiotics. We provide statistical evidence that a parallel measurement of the expression of the biomarkers guarantees optimal performance when using expression systems for screening libraries of novel substances. The general approach is also applicable to drug discovery for medical indications other than infectious diseases. As current antibiotic therapy is increasingly challenged by emerging drug-resistant bacteria, new technologies are required to identify and develop novel classes of antibiotics. A major bottleneck in today’s discovery efforts, however, is a lack of an efficient and standardized method for assaying the efficacy of a drug candidate. We propose a new high content screening approach for identifying efficacious molecules suitable for development of antibiotics. Key to our approach is a new microarray-based efficacy biomarker discovery strategy. We first produced a large dataset of transcriptional responses of Bacillus subtilis to numerous structurally diverse antibacterial drugs. Second we evaluated different protocols to optimize drug concentration and exposure time selection for profiling compounds of unknown mechanism. Finally we identified a surprisingly low number of gene transcripts (∼130) that were sufficient for identifying the mechanism of novel substances with reasonable accuracy (∼90%). We show that the statistics-based approach reveals a physiologically meaningful set of biomarkers that can be related to major bacterial defense mechanisms against antibiotics. We provide statistical evidence that a parallel measurement of the expression of the biomarkers guarantees optimal performance when using expression systems for screening libraries of novel substances. The general approach is also applicable to drug discovery for medical indications other than infectious diseases. In clinical practice, biomarkers are widely used for diagnosis, for disease prognosis, and for optimizing therapeutic strategies. Lately the use of biomarkers showed potential for guiding the discovery and development of novel therapeutics. For instance, the strategy sometimes referred to as toxicogenomics is a biomarker-based approach for evaluating the toxicity potential of a drug candidate by its characteristic biomarker expression profile (1Ellinger-Ziegelbauer H. Stuart B. Wahle B. Bomann W. Ahr H.J. Characteristic expression profiles induced by genotoxic carcinogens in rat liver.Toxicol. Sci. 2004; 77: 19-34Crossref PubMed Scopus (137) Google Scholar, 2Ellinger-Ziegelbauer H. Stuart B. Wahle B. Bomann W. Ahr H.J. Comparison of the expression profiles induced by genotoxic and nongenotoxic carcinogens in rat liver.Mutat. Res. 2005; 575: 61-84Crossref PubMed Scopus (194) Google Scholar). It has been shown that the mRNA expression profiles of toxicity biomarkers triggered by a toxicant correlate well with the underlying toxicity mechanism of the toxicant (3Goodsaid F.M. Genomic biomarkers of toxicity.Curr. Opin. Drug Discov. Dev. 2003; 6: 41-49PubMed Google Scholar). However, so far biomarkers are mostly used to predict adverse side effects of drug candidates. To date, there is little evidence that an analogous “efficacy biomarker approach” could help in evaluating the efficacy potential of a drug candidate (4Gunther E.C. Stone D.J. Gerwien R.W. Bento P. Heyes M.P. Prediction of clinical drug efficacy by classification of drug-induced genomic expression profiles in vitro.Proc. Natl. Acad. Sci. U. S. A. 2003; 100: 9608-9613Crossref PubMed Scopus (168) Google Scholar). A biomarker-based compound efficacy evaluation would be very attractive for many applications in the pharmaceutical industry as it would enable a standardized and automated approach to the drug discovery process. Here we propose a biomarker-based strategy for evaluating the efficacy of compounds with antibacterial activity. To systematically investigate the influence of currently known antibacterial compounds on bacterial gene expression, we produced a large antibiotic-response microarray dataset. We measured the whole-genome transcription response of Bacillus subtilis, a model organism for phylogenetically related major Gram-positive pathogens such as staphylococci, streptococci, and enterococci, to 112 different chemicals with antibacterial activity (see Supplemental Table S1). These antibiotic agents represent the majority of established and commercialized antibiotics such as β-lactams, macrolides, and quinolones. In addition, we included a number of developmental compounds as well as unspecifically acting substances with antibacterial activity such as DNA intercalators. For each of these compounds, we monitored the expression response of B. subtilis to different drug concentrations and exposure times. Here we present detailed results of the B. subtilis response to 46 different antibiotics. To define objective decision rules applicable to the problem of predicting the mechanism of action (MOA) 1The abbreviations used are: MOA, mechanism of action; MIC, minimum inhibitory concentration; SVM, support vector machine; MCR, misclassification rate; ANOVA, analysis of variance. of novel substances in compound library screens, we used supervised classification algorithms. These algorithms require a training set of mRNA profiles to define a separation function (“to train the classifier”) to assign a compound to its antibiotic MOA category solely based on the mRNA profile it triggers in B. subtilis. In this study, we focused on support vector machines (SVMs) as this algorithm class has been shown to be well suited for categorizing microarray data (5Ramaswamy S. Tamayo P. Rifkin R. Mukherjee S. Yeang C.H. Angelo M. Ladd C. Reich M. Latulippe E. Mesirov J.P. Poggio T. Gerald W. Loda M. Lander E.S. Golub T.R. Multiclass cancer diagnosis using tumor gene expression signatures.Proc. Natl. Acad. Sci. U. S. A. 2001; 98: 15149-15154Crossref PubMed Scopus (1657) Google Scholar). Using our mRNA profile reference database, we investigated several aspects of microarray-based MOA classifications. First we analyzed the effect of the experimental design on the MOA prediction performance. Second we identified gene sets whose expression carries most of the information that is indicative for characterizing the MOAs of the compound. In addition, we present an in depth discussion of the biological context of these “antibiotic efficacy biomarkers.” Last we discuss the biology underlying the biomarker concept by exemplifying the response of B. subtilis to two agents that both block DNA replication but via different molecular mechanisms. We show that the analysis of pathway activity patterns can pinpoint mechanistic details of novel substances. The central goal of our work was the identification of efficacy biomarkers whose compound-induced expression provides sufficient information about the underlying mechanisms of the compound. From a technical standpoint, this is critical information for developing robust assays to routinely characterize novel antibiotics. According to our knowledge, there are no published antibiotic efficacy biomarkers available to date that could be used for routinely assaying the mechanism of novel antibiotic substances. In addition, the implementation of MOA-specific screening processes is currently hampered by a lack of standard experiment protocols that could be used for compound library screens. Our work represents also in this regard a first step toward implementing novel expression-based high content screening strategies. B. subtilis 168 cultures were grown in Belitzky minimal medium (6Stulke J. Hanschke R. Hecker M. Temporal activation of beta-glucanase synthesis in Bacillus subtilis is mediated by the GTP pool.J. Gen. Microbiol. 1993; 139: 2041-2045Crossref PubMed Scopus (202) Google Scholar) to A600 = 0.4 at 37 °C. After removal of an aliquot (t = 0 min), the remaining untreated culture was treated with antibiotics and incubated further, t1 = 10 and t2 = 40 min. Each compound was examined in two concentrations, CH = 2 × MIC and CL = ⅕ × MIC (see Fig. 1). In case that the applied concentration already caused more than 25% growth reduction compared with the control at t = t2, the maximal concentration was reduced to 1-fold MIC or fractions of the MIC (see Supplemental Table S1). Details of sample preparation have been published previously (7Fischer H.P. Brunner N.A. Wieland B. Paquette J. Macko L. Ziegelbauer K. Freiberg C. Identification of antibiotic stress-inducible promoters: a systematic approach to novel pathway-specific reporter assays for antibacterial drug discovery.Genome Res. 2004; 14: 90-98Crossref PubMed Scopus (53) Google Scholar). In brief, experimental procedures followed the protocol for Eurogentec microarrays based on a two-color fluorescence technology (Eurogentec, Seraing, Belgium). The fluorescence intensities of the two dyes were detected via the Axon GenePix® 4000A confocal laser scanner using the image analysis software GenePix Pro (Axon Instruments, Foster City, CA). To minimize the influence of technical artifacts, we split each cDNA sample related to a drug concentration and exposure time and hybridized it separately to three different microarrays. For details regarding the technical and biological reproducibility, see Fischer et al. (7Fischer H.P. Brunner N.A. Wieland B. Paquette J. Macko L. Ziegelbauer K. Freiberg C. Identification of antibiotic stress-inducible promoters: a systematic approach to novel pathway-specific reporter assays for antibacterial drug discovery.Genome Res. 2004; 14: 90-98Crossref PubMed Scopus (53) Google Scholar). The signal intensities obtained from the microarray experiments were further processed by Genedata Expressionist® Pro 2.0 (Genedata, Basel, Switzerland) using the default settings. The signals of all features were used for a global microarray normalization, with the scaling factor being calculated, requiring the sum of all logarithmized values in the Cy3 channel to equal all logarithmized values in the Cy5 channel. Average -fold factors of replicate features were calculated following the data processing strategy described previously (7Fischer H.P. Brunner N.A. Wieland B. Paquette J. Macko L. Ziegelbauer K. Freiberg C. Identification of antibiotic stress-inducible promoters: a systematic approach to novel pathway-specific reporter assays for antibacterial drug discovery.Genome Res. 2004; 14: 90-98Crossref PubMed Scopus (53) Google Scholar). The performance of classifiers such as SVMs is typically assessed by so-called “leave-one-out” approaches or related strategies (5Ramaswamy S. Tamayo P. Rifkin R. Mukherjee S. Yeang C.H. Angelo M. Ladd C. Reich M. Latulippe E. Mesirov J.P. Poggio T. Gerald W. Loda M. Lander E.S. Golub T.R. Multiclass cancer diagnosis using tumor gene expression signatures.Proc. Natl. Acad. Sci. U. S. A. 2001; 98: 15149-15154Crossref PubMed Scopus (1657) Google Scholar, 8Staunton J.E. Slonim D.K. Coller H.A. Tamayo P. Angelo M.J. Park J. Scherf U. Lee J.K. Reinhold W.O. Weinstein J.N. Mesirov J.P. Lander E.S. Golub T.R. Chemosensitivity prediction by transcriptional profiling.Proc. Natl. Acad. Sci. U. S. A. 2001; 98: 10787-10792Crossref PubMed Scopus (589) Google Scholar). For this, the experiment dataset is split into the (trivial) set of one randomly chosen experiment to be classified and a training set of the remaining (N − 1) experiments. Here we applied the leave-one-out strategy by randomly separating the experiment set into X experiments to be classified in a blinded manner and N − X experiments remaining in the training set using a test set fraction P = X/N = 25%. To calculate the average misclassification rates (MCRs), we compared the SVM-predicted results with the known compound MOAs and averaged the misclassification ratios over r = 100 independent runs. The MCR was calculated for different sets of marker genes defined by ANOVA p value thresholds using the MOA classes listed in Table I. Figs. 2 and 3 show these dependences for various experimental conditions.Table IAntibiotic reference compounds and their mechanisms of actionCodeCompound nameMOA: targeted pathway (protein)DNA replication inhibitors (DNAaA priori MOA categorization. MOA categories: inhibition of protein biosynthesis (PROTEIN), aminoacyl-tRNA synthesis (AATS), cell wall biosynthesis (CELL WALL), fatty acid biosynthesis (FAB), RNA synthesis (RNA), and DNA replication (DNA). Antibiotics acting via unspecific effects are DNA-intercalating or -binding agents actinomycin D, ethidium bromide, and netropsin; the pyrimidine synthesis-inhibiting and protein-alkylating antibiotic showdomycin; the membrane-perturbing agents monensin and polymyxin B; the protein-alkylating or -oxidizing compounds N-ethylmaleimide and nitrofurantoin; and the cell wall biosynthesis-inhibiting but also directly or indirectly membrane-permeabilizing antibiotics nisin and tunicamycin.)AzAzaserinePurine synthesis/DNA carboxymethylationFlFluorouracilPyrimidine synthesisNNovobiocinDNA replication (gyrase-topoisomerase IV)NaNalidixic acidDNA replication (gyrase-topoisomerase IV)PCiprofloxacinDNA replication (gyrase-topoisomerase IV)SuSulfamethoxazoleNucleotide biosynthesis (folate biosynthesis)TTrimethoprimNucleotide biosynthesis (folate biosynthesis)TrTrovafloxacinDNA replication (gyrase-topoisomerase IV)XMoxifloxacinDNA replication (gyrase-topoisomerase IV)RNA synthesis inhibitors (RNAaA priori MOA categorization. MOA categories: inhibition of protein biosynthesis (PROTEIN), aminoacyl-tRNA synthesis (AATS), cell wall biosynthesis (CELL WALL), fatty acid biosynthesis (FAB), RNA synthesis (RNA), and DNA replication (DNA). Antibiotics acting via unspecific effects are DNA-intercalating or -binding agents actinomycin D, ethidium bromide, and netropsin; the pyrimidine synthesis-inhibiting and protein-alkylating antibiotic showdomycin; the membrane-perturbing agents monensin and polymyxin B; the protein-alkylating or -oxidizing compounds N-ethylmaleimide and nitrofurantoin; and the cell wall biosynthesis-inhibiting but also directly or indirectly membrane-permeabilizing antibiotics nisin and tunicamycin.)CoCorallopyroninRNA polymeraseRiRifampicinRNA polymeraseSvStreptavidinRNA polymeraseAminoacyl-tRNA synthesis inhibitors (AATS)aA priori MOA categorization. MOA categories: inhibition of protein biosynthesis (PROTEIN), aminoacyl-tRNA synthesis (AATS), cell wall biosynthesis (CELL WALL), fatty acid biosynthesis (FAB), RNA synthesis (RNA), and DNA replication (DNA). Antibiotics acting via unspecific effects are DNA-intercalating or -binding agents actinomycin D, ethidium bromide, and netropsin; the pyrimidine synthesis-inhibiting and protein-alkylating antibiotic showdomycin; the membrane-perturbing agents monensin and polymyxin B; the protein-alkylating or -oxidizing compounds N-ethylmaleimide and nitrofurantoin; and the cell wall biosynthesis-inhibiting but also directly or indirectly membrane-permeabilizing antibiotics nisin and tunicamycin.PhePhenyl-thiazolylurea derivativePhenylalanyl-tRNA synthetaseUMupirocinIsoleucyl-tRNA synthetaseProtein biosynthesis inhibitors (PROTEIN)aA priori MOA categorization. MOA categories: inhibition of protein biosynthesis (PROTEIN), aminoacyl-tRNA synthesis (AATS), cell wall biosynthesis (CELL WALL), fatty acid biosynthesis (FAB), RNA synthesis (RNA), and DNA replication (DNA). Antibiotics acting via unspecific effects are DNA-intercalating or -binding agents actinomycin D, ethidium bromide, and netropsin; the pyrimidine synthesis-inhibiting and protein-alkylating antibiotic showdomycin; the membrane-perturbing agents monensin and polymyxin B; the protein-alkylating or -oxidizing compounds N-ethylmaleimide and nitrofurantoin; and the cell wall biosynthesis-inhibiting but also directly or indirectly membrane-permeabilizing antibiotics nisin and tunicamycin.AActinoninPeptide deformylaseDoDoxycyclineTranslationEErythromycinTranslationFuFusidic acidTranslation (elongation factor G)GGentamicinTranslationHChloramphenicolTranslationKKanamycinTranslationLiLinezolidTranslationPaPactamycinTranslationPuPuromycinTranslationTanTAN 1057TranslationYAzithromycinTranslationFatty acid biosynthesis inhibitors (FAB)aA priori MOA categorization. MOA categories: inhibition of protein biosynthesis (PROTEIN), aminoacyl-tRNA synthesis (AATS), cell wall biosynthesis (CELL WALL), fatty acid biosynthesis (FAB), RNA synthesis (RNA), and DNA replication (DNA). Antibiotics acting via unspecific effects are DNA-intercalating or -binding agents actinomycin D, ethidium bromide, and netropsin; the pyrimidine synthesis-inhibiting and protein-alkylating antibiotic showdomycin; the membrane-perturbing agents monensin and polymyxin B; the protein-alkylating or -oxidizing compounds N-ethylmaleimide and nitrofurantoin; and the cell wall biosynthesis-inhibiting but also directly or indirectly membrane-permeabilizing antibiotics nisin and tunicamycin.BMoiramide BFatty acid biosynthesis (acetyl-CoA carboxylase)CCeruleninFatty acid biosynthesis (β-ketoacyl-acyl carrier protein synthetase)ITriclosanFatty acid biosynthesis (enoyl-acyl carrier protein reductase)Cell wall biosynthesis inhibitors (CELL WALL)aA priori MOA categorization. MOA categories: inhibition of protein biosynthesis (PROTEIN), aminoacyl-tRNA synthesis (AATS), cell wall biosynthesis (CELL WALL), fatty acid biosynthesis (FAB), RNA synthesis (RNA), and DNA replication (DNA). Antibiotics acting via unspecific effects are DNA-intercalating or -binding agents actinomycin D, ethidium bromide, and netropsin; the pyrimidine synthesis-inhibiting and protein-alkylating antibiotic showdomycin; the membrane-perturbing agents monensin and polymyxin B; the protein-alkylating or -oxidizing compounds N-ethylmaleimide and nitrofurantoin; and the cell wall biosynthesis-inhibiting but also directly or indirectly membrane-permeabilizing antibiotics nisin and tunicamycin.FCefoxitinCell wall biosynthesisMMethicillinCell wall biosynthesisMeMersacidinCell wall biosynthesisOOxacillinCell wall biosynthesisRRistocetinCell wall biosynthesisRaRamoplaninCell wall biosynthesisVVancomycinCell wall biosynthesisa A priori MOA categorization. MOA categories: inhibition of protein biosynthesis (PROTEIN), aminoacyl-tRNA synthesis (AATS), cell wall biosynthesis (CELL WALL), fatty acid biosynthesis (FAB), RNA synthesis (RNA), and DNA replication (DNA). Antibiotics acting via unspecific effects are DNA-intercalating or -binding agents actinomycin D, ethidium bromide, and netropsin; the pyrimidine synthesis-inhibiting and protein-alkylating antibiotic showdomycin; the membrane-perturbing agents monensin and polymyxin B; the protein-alkylating or -oxidizing compounds N-ethylmaleimide and nitrofurantoin; and the cell wall biosynthesis-inhibiting but also directly or indirectly membrane-permeabilizing antibiotics nisin and tunicamycin. Open table in a new tab Fig. 3.Identification of optimal experimental conditions for expression-based antibiotic MOA screens. The average MCR is calculated for specific experimental conditions defined by combinations of antibiotic dosage and exposure time (analogously as in Fig. 2). Solid blue curves indicate the MCR for high dosage experiments (for short and long exposure times, H-1 and H-2), while solid violet lines indicate the MCR for low concentration experiments (L-1 and L-2, respectively). The black curve represents the MOA classifier performance when using all experiments. Our results show that the low concentration, long exposure experiments (L-2) are not capable of reasonably discriminating the correct antibiotic MOA classes (optimal MCR = 29.1%). The L-1 curve, by contrast, achieves much better MCRs (optimal MCR = 11.6%), requiring the expression profiles of 1,024 biomarker profiles for optimally identifying the antibacterial mechanism of uncharacterized compounds. The optimal MOA classification rate (MCR = 13.6%) is obtained for the H-2 experiment training set, requiring only a relatively small set of 181 antibiotic efficacy biomarkers (see arrow).View Large Image Figure ViewerDownload Hi-res image Download (PPT) The whole-genome annotation of B. subtilis was taken from the Genedata Phylosopher® system. Additionally we compiled and incorporated information from specialized publications. In this work, the following regulons were considered: relA-dependent stringent response, FapR-dependent fatty acid and phospholipid biosynthesis genes, and the SOS-, CodY-, and alternative RNA polymerase sigma factor-dependent regulons such as sigB, sigH, sigF, sigE, sigG, sigK, sigW, sigM, sigX, and sigY (for details and references see Freiberg et al. (9Freiberg C. Fischer H.P. Brunner N.A. Discovering the mechanism of action of novel antibacterial agents through transcriptional profiling of conditional mutants.Antimicrob. Agents Chemother. 2005; 49: 749-759Crossref PubMed Scopus (93) Google Scholar)). The basis for pathway categorizations are the Genedata Phylosopher annotations. The full biomarker annotations are compiled in Supplemental Table S2. We calculated the over-representation of biomarkers assigned to specific pathways or regulons using Fisher’s exact test. The frequency of each functional category in the biomarker set was compared with the frequency in the “background set” corresponding to the whole set of genes encoded by the B. subtilis genome. As a background, we used the annotation information for all ∼4,100 B. subtilis genes. More specifically the probability of observing i biomarkers that are predicted to be related to a specific functional class Y in a biomarker set consisting of n genes (in our example is n = 181) is given by (PFE(Y) = (fi) (g − fn − i)/ (gn) where f is the total number of genes being associated to the functional class Y and g is the total number of genes encoded by B. subtilis. The pathway over-representation calculations were performed using the Genedata Expressionist Pro 2.0 system. A whole-genome, two-channel microarray technology was used to monitor the transcriptional response of virtually all ∼4,100 B. subtilis genes (10Kunst F. Ogasawara N. Moszer I. Albertini A.M. Alloni G. Azevedo V. Bertero M.G. Bessieres P. Bolotin A. Borchert S. Borriss R. Boursier L. Brans A. Braun M. Brignell S.C. Bron S. Brouillet S. Bruschi C.V. Caldwell B. Capuano V. Carter N.M. Choi S.K. Codani J.J. Connerton I.F. Danchin A. et al.The complete genome sequence of the gram-positive bacterium Bacillus subtilis.Nature. 1997; 390: 249-256Crossref PubMed Scopus (3134) Google Scholar). In previous studies, we showed that this technology enables the parallel measurement of the transcriptional induction or repression of all genes when exposing bacterial cultures to substances with antibacterial potential (7Fischer H.P. Brunner N.A. Wieland B. Paquette J. Macko L. Ziegelbauer K. Freiberg C. Identification of antibiotic stress-inducible promoters: a systematic approach to novel pathway-specific reporter assays for antibacterial drug discovery.Genome Res. 2004; 14: 90-98Crossref PubMed Scopus (53) Google Scholar, 9Freiberg C. Fischer H.P. Brunner N.A. Discovering the mechanism of action of novel antibacterial agents through transcriptional profiling of conditional mutants.Antimicrob. Agents Chemother. 2005; 49: 749-759Crossref PubMed Scopus (93) Google Scholar, 11Freiberg C. Brunner N.A. Genome-wide mRNA profiling: impact on compound evaluation and target identification in anti-bacterial research.Targets. 2002; 1: 20-29Crossref Google Scholar). For the purpose of this study, the transcriptional response to a set of 36 well investigated antibiotics and 10 unspecifically acting substances were examined. The antibiotic compounds represent six different MOA classes (see Table I) broadly categorized as inhibitors of protein biosynthesis (“PROTEIN”), aminoacyl-tRNA synthesis (“AATS”), cell wall biosynthesis (“CELL WALL”), fatty acid biosynthesis (“FAB”), RNA synthesis (“RNA”), and replication inhibitors (“DNA”) (12Chopra I. Hesse L. O’Neill A. Exploiting current understanding of antibiotic action for discovery of new drugs.J. Appl. Microbiol. 2002; 92: 4S-15SCrossref PubMed Google Scholar). Antibiotic dosage and treatment time are known to be of critical importance for gene expression (13Freiberg C. Brotz-Oesterhelt H. Functional genomics in antibacterial drug discovery.Drug Discov. Today. 2005; 10: 927-935Crossref PubMed Scopus (45) Google Scholar), hence we suspected that these parameters will also play a role when using microarrays as highly parallel assay systems. Thus, in contrast to previous antibiotic microarray studies (14Hutter B. Schaab C. Albrecht S. Borgmann M. Brunner N.A. Freiberg C. Ziegelbauer K. Rock C.O. Ivanov I. Loferer H. Prediction of mechanisms of action of antibacterial compounds by gene expression profiling.Antimicrob. Agents Chemother. 2004; 48: 2838-2844Crossref PubMed Scopus (133) Google Scholar), it was necessary to systematically measure dosage and exposure time effects. We assayed each compound according to a standard protocol based on objective growth curve characteristics. Each bacterial culture was split and treated with two different drug concentrations, a “high” (=H) and a “low” (=L) concentration. For each culture treated, mRNA was harvested after t1 = 10 min and t2 = 40 min exposure time, respectively (see Fig. 1 and “Experimental Procedures”). Exposure times t1 and t2 (=“1” and “2”) were chosen with respect to the typical cell division time of B. subtilis in Belitzky minimal medium (t2 = treplication ∼ 40 min (6Stulke J. Hanschke R. Hecker M. Temporal activation of beta-glucanase synthesis in Bacillus subtilis is mediated by the GTP pool.J. Gen. Microbiol. 1993; 139: 2041-2045Crossref PubMed Scopus (202) Google Scholar), t1 = t2/4) to resolve antibiotic stress effects occurring on two biologically relevant time scales. The combination of different dosages and exposure times for a wide range of antibiotic compounds allowed us to obtain an unbiased view on a wide spectrum of antibiotics-induced stress states. In total, the results presented in this study are based on 309 individual hybridization experiments. Obviously not all gene expression values contribute equally to the MOA-specific information of a compound-induced expression profile. Thus, identifying a biomarker set for MOA prediction with minimal false negative and false positive rates is a crucial step toward developing assays for routine compound MOA profiling. A primary goal of this study was to quantitatively evaluate the performance of supervised classification algorithms for predicting the MOA of novel antibiotics when using only the expression values of specific gene subsets as input. Here we focus on linear-kernel SVMs for classifying the MOA of a compound. The performance of the classifier was evaluated using a “leave-X-out” strategy (see “Experimental Procedures”). In brief, we randomly separated the experiment set into X experiments to be classified in a blinded manner and N − X experiments remaining in the training set. By comparing the results of the classifier with the known MOAs of the compounds, we were able to quantify the MOA prediction accuracy of the classifier. To investigate the dependence of the classifier accuracy on different gene sets, we ranked the genes according to their specific expression patterns, here quantified by their ANOVA p value based on the experiment groups defined by the six major a priori MOA categorizations (see Table I). The dependence of the averaged MCRs on the biomarker set size and various experimental conditions are displayed in Figs. 2 and 3. When pooling all experimental conditions, the MCR is relatively high for smaller biomarker sets (Fig. 2, black solid line) even if the ANOVA p values of the biomarker are highly significant. For instance, for the 10 most discriminating ANOVA genes (all p values <10−35) the misclassification rate still exceeds 50%. The expression profiles of the most discriminative genes are apparently not sufficient for distinguishing the underlying MOA classes. However, as more biomarkers are taken into account, the average misclassification rate is significantly reduced. The optimal biomarker set was identified for n = 2,896 genes with an MCR of 7.1%, which is only slightly below the MCR when taking into account all 4,100 B. subtilis gene expression profiles (MCR = 7.4%). Although the correct MOA classification rate is reasonably good in this setup (∼93%), the number of efficacy biomarker signals that is required for accomplishing the optimal MOA predict
更多查看译文
AI 理解论文
溯源树
样例
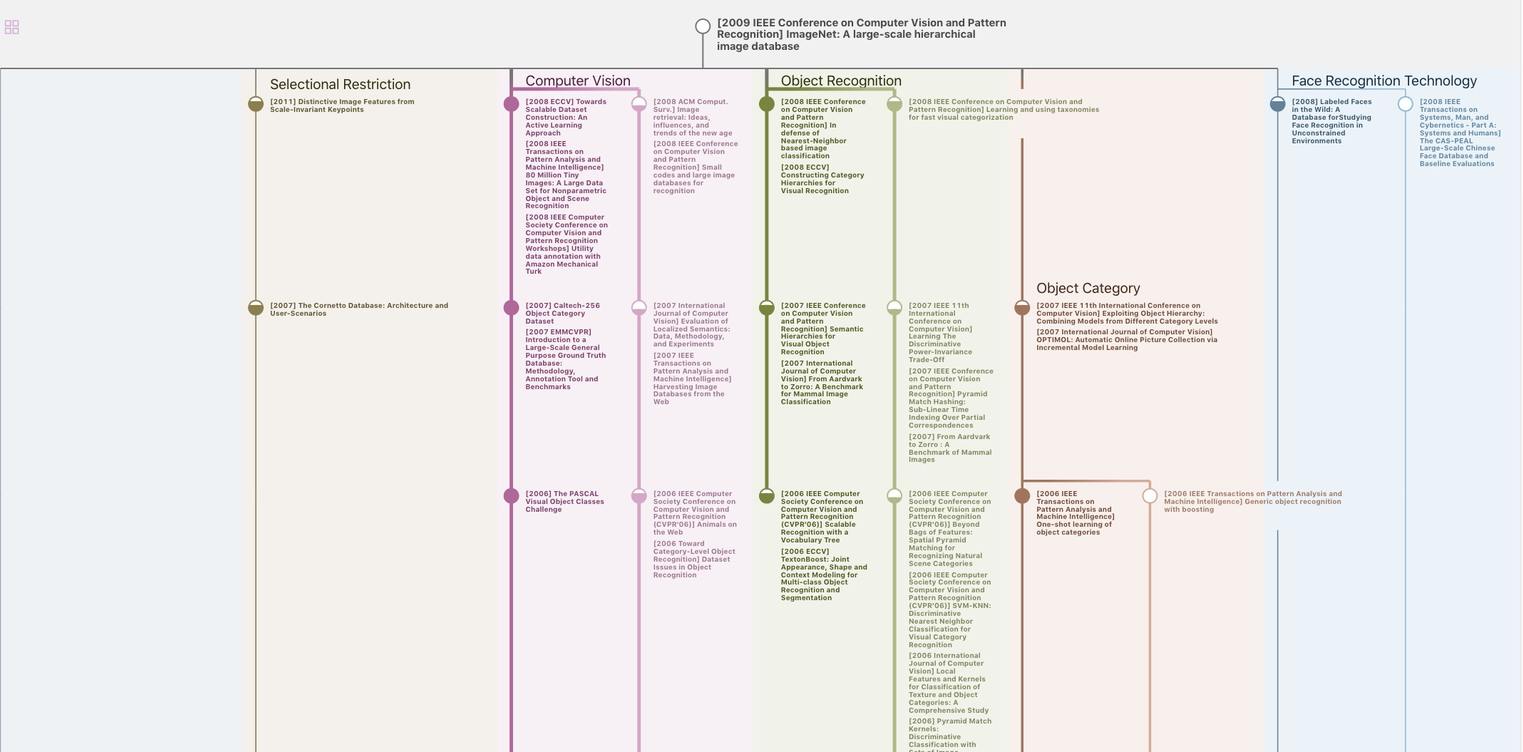
生成溯源树,研究论文发展脉络
Chat Paper
正在生成论文摘要