End-to-end LPCNet: A Neural Vocoder With Fully-Differentiable LPC Estimation
Conference of the International Speech Communication Association (INTERSPEECH)(2022)
摘要
Neural vocoders have recently demonstrated high quality speech synthesis, but typically require a high computational complexity. LPCNet was proposed as a way to reduce the complexity of neural synthesis by using linear prediction~(LP) to assist an autoregressive model. At inference time, LPCNet relies on the LP coefficients being explicitly computed from the input acoustic features. That makes the design of LPCNet-based systems more complicated, while adding the constraint that the input features must represent a clean speech spectrum. We propose an end-to-end version of LPCNet that lifts these limitations by learning to infer the LP coefficients in the frame rate network from the input features. Results show that the proposed end-to-end approach can reach the same level of quality as the original LPCNet model, but without explicit LP analysis. Our open-source end-to-end model still benefits from LPCNet's low complexity, while allowing for any type of conditioning features.
更多查看译文
关键词
neural vocoder,end-to-end,fully-differentiable
AI 理解论文
溯源树
样例
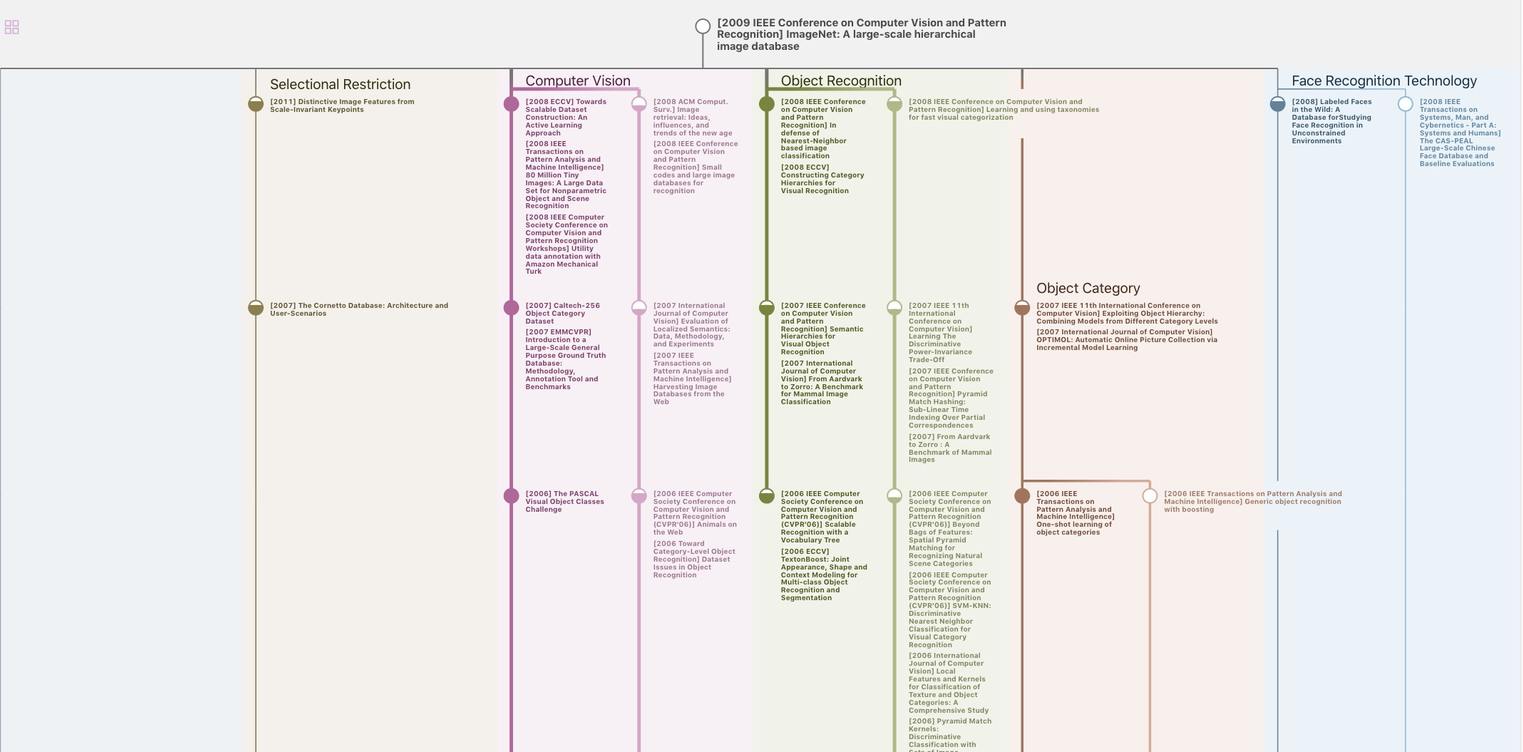
生成溯源树,研究论文发展脉络
Chat Paper
正在生成论文摘要