Mosaic
Complex Data Warehousing and Knowledge Discovery for Advanced Retrieval Development(2010)
摘要
Strong theoretical foundation and low computational complexity make representative-based clustering one of the most popular approaches for a clustering problem. Despite those superiorities, it presents two main drawbacks: the shape of clusters obtained is limited to convex shapes, and its performance is highly dependent on seeds initialization. To address these problems, the authors introduce MOSAIC, a novel agglomerative clustering algorithm, which greedily merges neighboring clusters maximizing a plug-in fitness function. The key idea is that by considering neighboring relationship computed using Gabriel Graphs among cluster, MOSAIC can derive non-convex shapes as the unions of small clusters previously generated by a representative-based clustering algorithm. The authors evaluate MOSAIC for traditional unsupervised clustering with k-means and DBSCAN, and also for supervised clustering. The experimental results show that compared to k-means stand-alone, their proposed post-processing techniques obtain higher quality clusters, whereas compared to DBSCAN results, MOSAIC is capable of identifying comparable arbitrary shape clusters, given a suitable fitness function. In addition, MOSAIC can cope with problems of clustering on high dimensional data. The authors also claim that MOSAIC can be employed as an effective post-processing clustering algorithm to further improve the quality of clustering.
更多查看译文
AI 理解论文
溯源树
样例
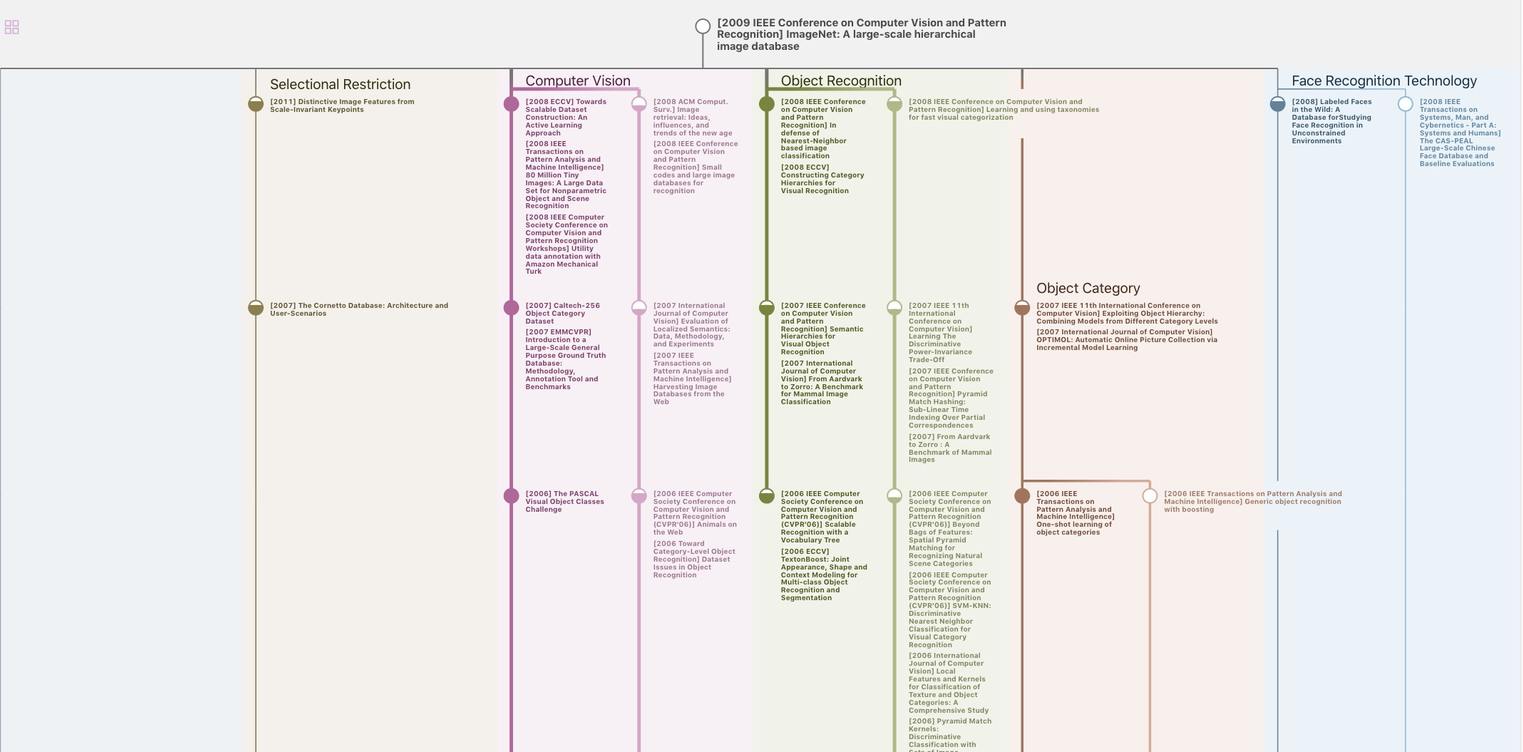
生成溯源树,研究论文发展脉络
Chat Paper
正在生成论文摘要