Joint spatiotemporal modelling reveals seasonally dynamic patterns of Japanese encephalitis vector abundance across India
PLOS NEGLECTED TROPICAL DISEASES(2022)
摘要
Predicting vector abundance and seasonality, key components of mosquito-borne disease (MBD) hazard, is essential to determine hotspots of MBD risk and target interventions effectively. Japanese encephalitis (JE), an important MBD, is a leading cause of viral encephalopathy in Asia with 100,000 cases estimated annually, but data on the principal vector Culex tritaeniorhynchus is lacking. We developed a Bayesian joint-likelihood model that combined information from available vector occurrence and abundance data to predict seasonal vector abundance for C. tritaeniorhynchus (a constituent of JE hazard) across India, as well as examining the environmental drivers of these patterns. Using data collated from 57 locations from 24 studies, we find distinct seasonal and spatial patterns of JE vector abundance influenced by climatic and land use factors. Lagged precipitation, temperature and land use intensity metrics for rice crop cultivation were the main drivers of vector abundance, independent of seasonal, or spatial variation. The inclusion of environmental factors and a seasonal term improved model prediction accuracy (mean absolute error [MAE] for random cross validation = 0.42) compared to a baseline model representative of static hazard predictions (MAE = 0.51), signalling the importance of seasonal environmental conditions in predicting JE vector abundance. Vector abundance varied widely across India with high abundance predicted in northern, north-eastern, eastern, and southern regions, although this ranged from seasonal (e.g., Uttar Pradesh, West Bengal) to perennial (e.g., Assam, Tamil Nadu). One-month lagged predicted vector abundance was a significant predictor of JE outbreaks (odds ratio 2.45, 95% confidence interval: 1.52-4.08), highlighting the possible development of vector abundance as a proxy for JE hazard. We demonstrate a novel approach that leverages information from sparse vector surveillance data to predict seasonal vector abundance-a key component of JE hazard-over large spatial scales, providing decision-makers with better guidance for targeting vector surveillance and control efforts. Author summary Japanese encephalitis (JE) is the leading cause of viral encephalopathy in Asia with an estimated 100,000 annual cases and 25,000 deaths. However, insufficient data on the predominant mosquito vector Culex tritaeniorhynchus-a key component of JE hazard-precludes hazard estimation required to target public health interventions. Previous studies have provided limited estimates of JE hazard, often predicting geographic distributions of potential vector occurrence without accounting for vector abundance, seasonality, or uncertainty in predictions. This study details a novel approach to predict spatiotemporal patterns in JE vector abundance using a joint-likelihood modelling technique that leverages information from sparse vector surveillance data. We showed that patterns in JE vector abundance were driven by seasonality and environmental factors and so demonstrated the limitations of previously available static vector distribution maps in estimating the vector population component of JE hazard. One-month lagged vector abundance predictions showed a positive relationship with JE outbreaks, signalling the potential use of vector abundance as a proxy for JE hazard. While vector surveillance data are limited, joint-likelihood models offer a useful approach to inform vector abundance predictions. This study provides decision-makers with a more complete picture of the distribution of JE vector abundance and can be used to target vector surveillance and control efforts and enhance the allocation of resources.
更多查看译文
AI 理解论文
溯源树
样例
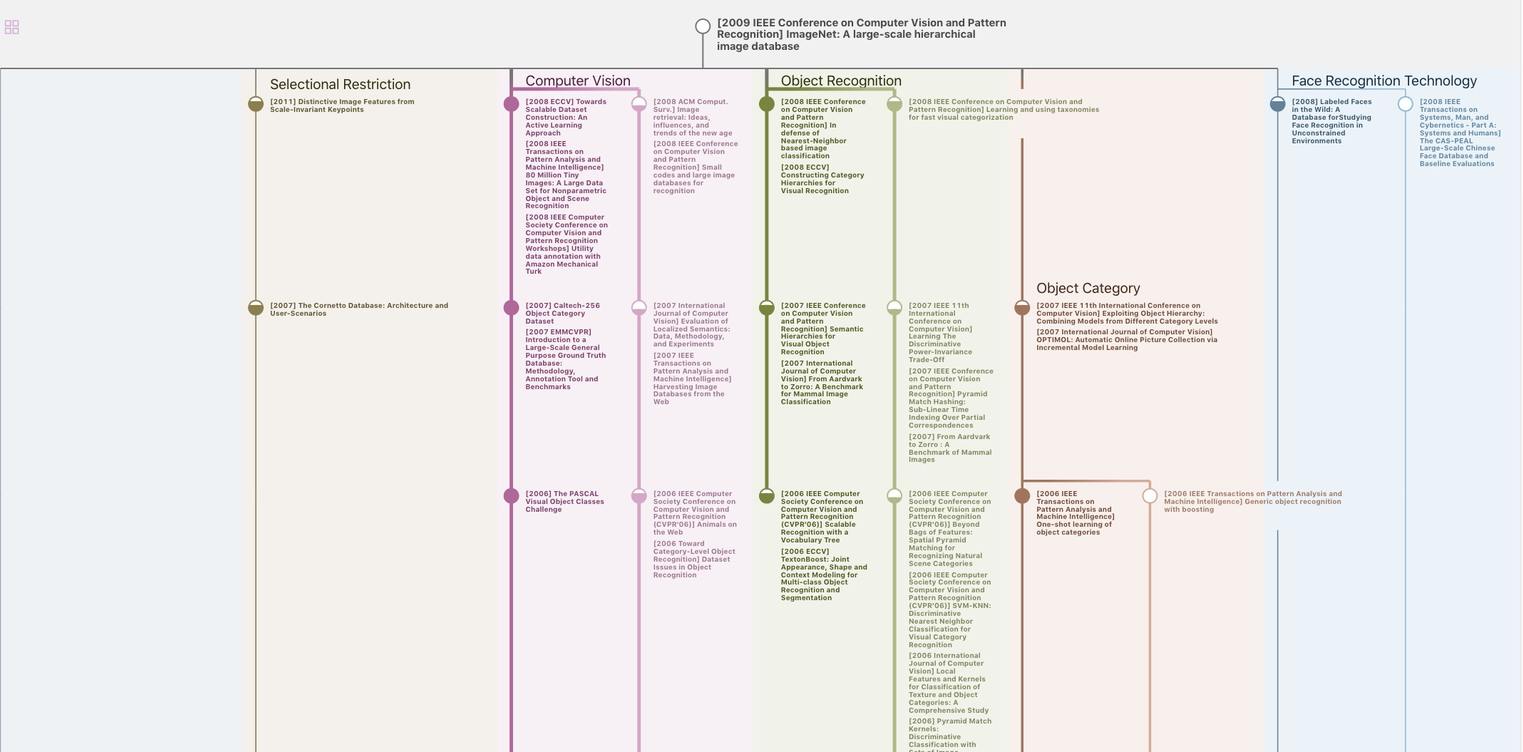
生成溯源树,研究论文发展脉络
Chat Paper
正在生成论文摘要