Radiomics-based tumor phenotype determination based on medical imaging and tumor microenvironment in a preclinical setting.
Radiotherapy and oncology : journal of the European Society for Therapeutic Radiology and Oncology(2022)
摘要
BACKGROUND AND PURPOSE:Radiomics analyses have been shown to predict clinical outcomes of radiotherapy based on medical imaging-derived biomarkers. However, the biological meaning attached to such image features often remains unclear, thus hindering the clinical translation of radiomics analysis. In this manuscript, we describe a preclinical radiomics trial, which attempts to establish correlations between the expression of histological tumor microenvironment (TME)- and magnetic resonance imaging (MRI)-derived image features.
MATERIALS & METHODS:A total of 114 mice were transplanted with the radioresistant and radiosensitive head and neck squamous cell carcinoma cell lines SAS and UT-SCC-14, respectively. The models were irradiated with five fractions of protons or photons using different doses. Post-treatment T1-weighted MRI and histopathological evaluation of the TME was conducted to extract quantitative features pertaining to tissue hypoxia and vascularization. We performed radiomics analysis with leave-one-out cross validation to identify the features most strongly associated with the tumor's phenotype. Performance was assessed using the area under the curve (AUCValid) and F1-score. Furthermore, we analyzed correlations between TME- and MRI features using the Spearman correlation coefficient ρ.
RESULTS:TME and MRI-derived features showed good performance (AUCValid,TME = 0.72, AUCValid,MRI = 0.85, AUCValid,Combined=0.85) individual tumor phenotype prediction. We found correlation coefficients of ρ=-0.46 between hypoxia-related TME features and texture-related MRI features. Tumor volume was a strong confounder for MRI feature expression.
CONCLUSION:We demonstrated a preclinical radiomics implementation and notable correlations between MRI- and TME hypoxia-related features. Developing additional TME features may help to further unravel the underlying biology.
更多查看译文
AI 理解论文
溯源树
样例
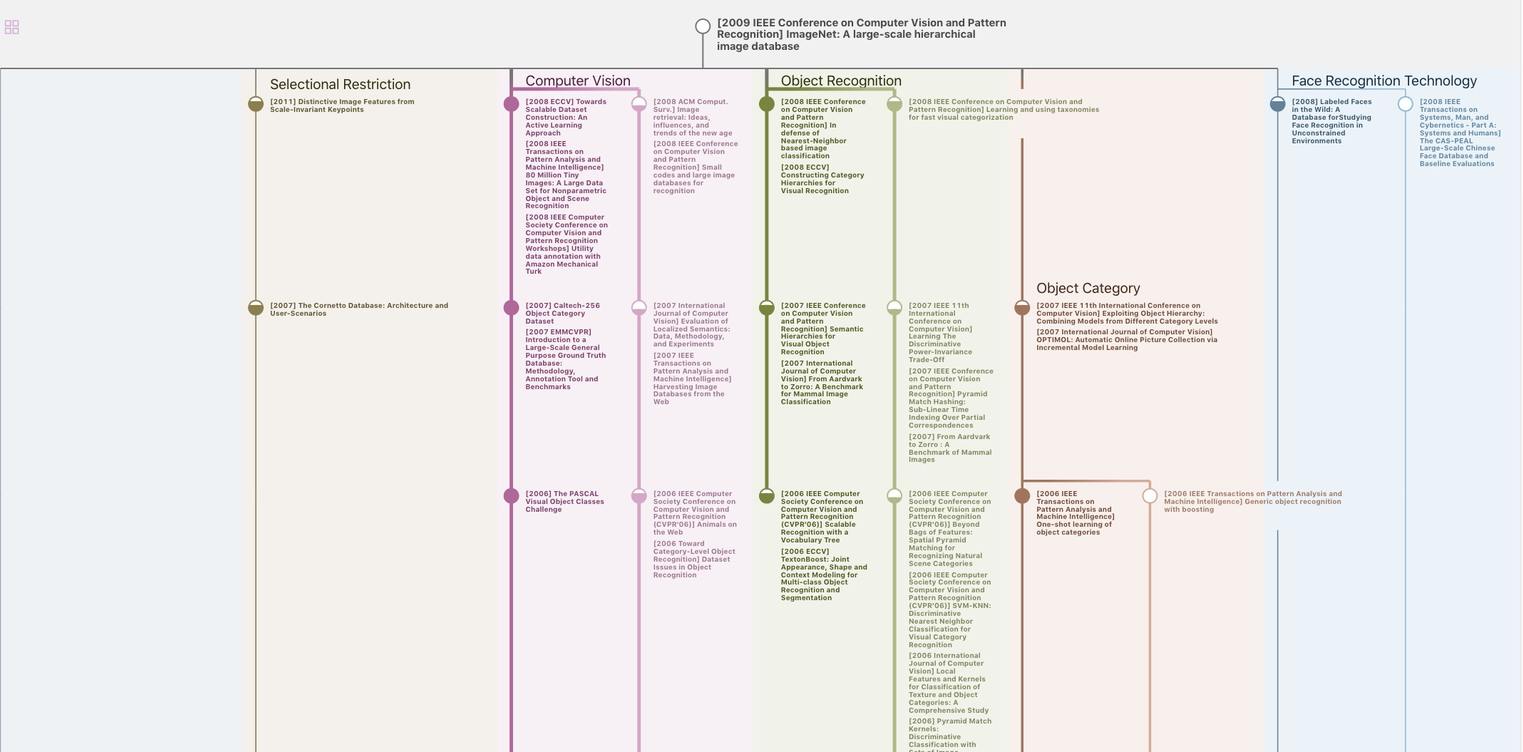
生成溯源树,研究论文发展脉络
Chat Paper
正在生成论文摘要