Streamline Rock Facies Classification with Deep Learning Cognitive Process
Day 3 Wed, October 11, 2017(2017)
摘要
Abstract Hydrocarbon resource identification process involves significant endeavors and persistent efforts to identify sweet spots and economical discoveries. To help streamline the process and better support subsurface teams, a suite of machine learning models have been piloted for integration into the workflow. This paper introduces a sub-process of classification models for automatic prediction of reservoir rock facies types based on acquired sample well logs. We present results from a synthesized dataset based on a formation with a series of different stacked reservoir rock types. The main reservoir is further sub-divided into four sections. Our method is based on cognitive deep structures, where more complex facies attributes are inferred from lower level raw features. The algorithm mimics biological brain hierarchical order for recognizing patterns/objects. The incremental learning process iteratively improves on facies identification as new evidence is introduced to the machine learner. We train the model on a small sample of well log attributes and validate performance on excluded wells. The model performs relatively well on a limited training sample. In a typical field development process, only a few appraisal wells are available for explorers to determine resources location and juxtaposition. Results show that distinction between good quality homogenous to parallel or cross-bedded rock from poor quality clay rich laminated facies is well predicted. This is an important step forward, as it helps geologists and geophysicists re-affirm conclusions about best, good, and poor quality rock type locations. The integration of deep learning models into the appraisal process provides an additional assessment lens and helps accelerate full field development of the resource. This paper introduces an enhanced capability for intelligent classification of reservoir facies based on well log attributes. The cognitive deep learning sub-system has the advantage of self-adaptability as more samples become available. Our approach yield superior results relative to the best outcome of an industry collaborative facies prediction using machine learning, and is a demonstration of the potential of emerging digital capabilities for Upstream workflows.
更多查看译文
AI 理解论文
溯源树
样例
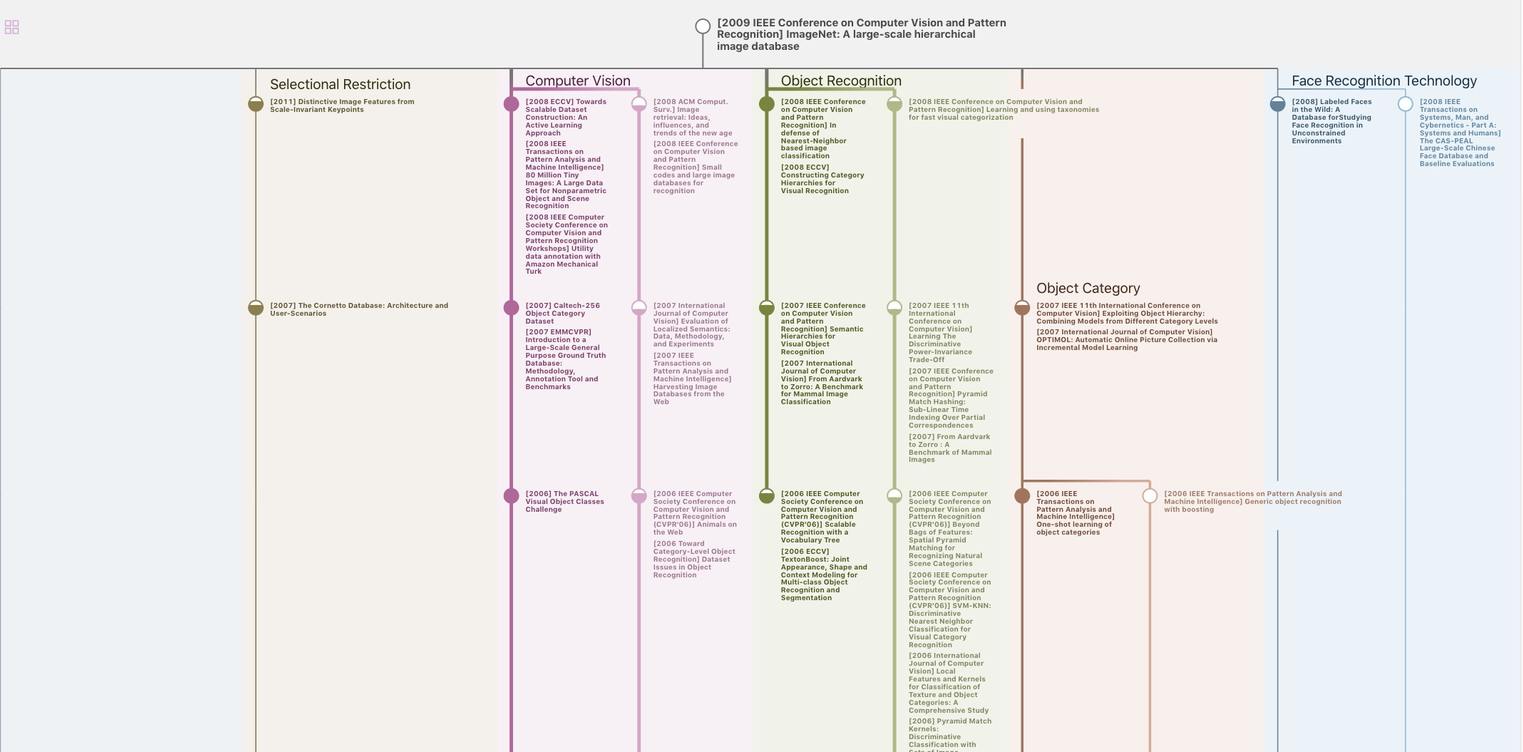
生成溯源树,研究论文发展脉络
Chat Paper
正在生成论文摘要