Conditionally optimal weights and forward-looking approaches to combining forecasts
International Journal of Forecasting(2024)
摘要
In forecasting, there is a tradeoff between in-sample fit and out-of-sample forecast accuracy. Parsimonious model specifications typically outperform richer model specifications. Consequently, information is often withheld from a forecast to prevent over-fitting the data. We show that one way to exploit this information is through forecast combination. Optimal combination weights in this environment minimize the conditional mean squared error that balances the conditional bias and the conditional variance of the combination. The bias-adjusted conditionally optimal forecast weights are time varying and forward looking. Real-time tests of conditionally optimal combinations of model-based forecasts and surveys of professional forecasters show significant gains in forecast accuracy relative to standard benchmarks for inflation and other macroeconomic variables.
更多查看译文
关键词
Forecast combination,Conditionally optimal weights,Forecast combination puzzle,Inflation,Phillips curve
AI 理解论文
溯源树
样例
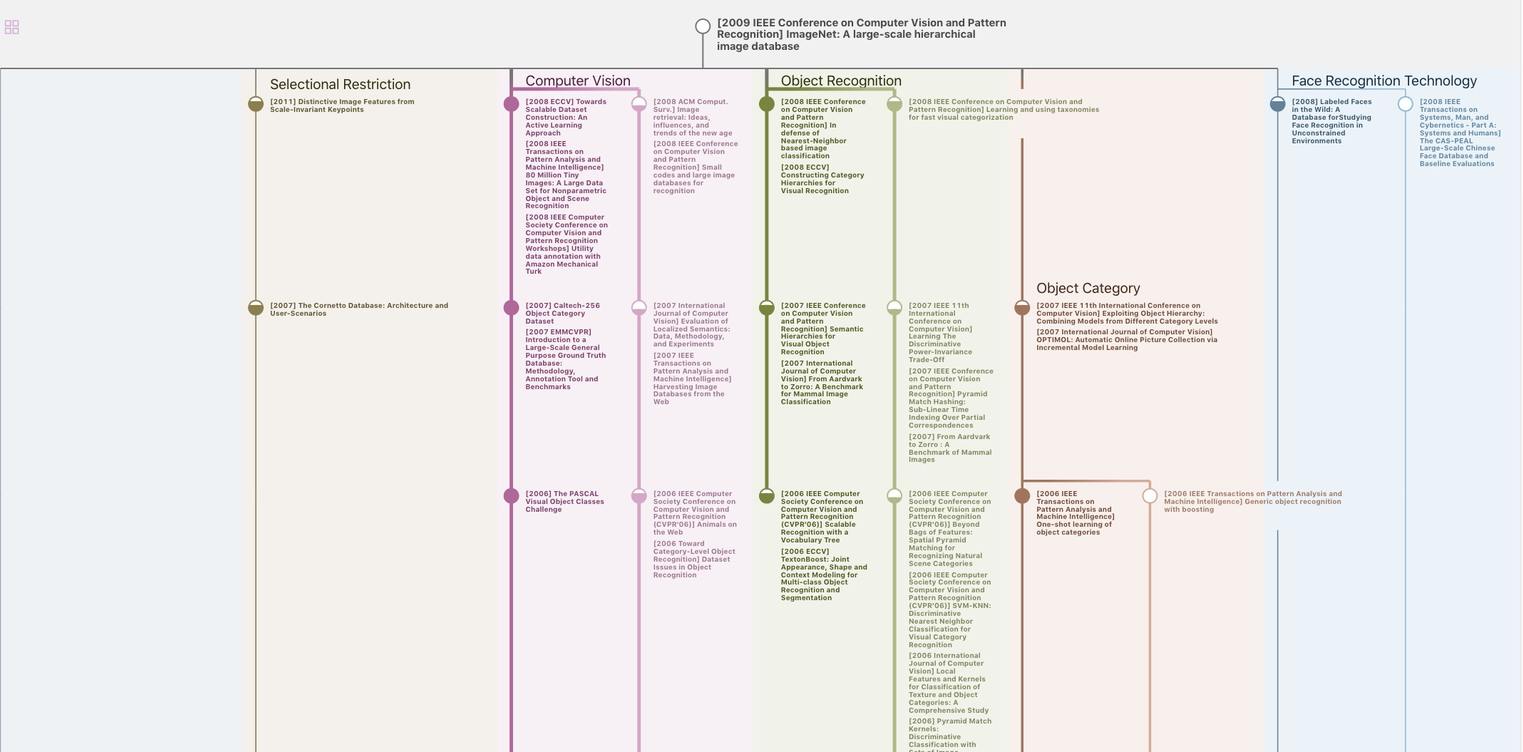
生成溯源树,研究论文发展脉络
Chat Paper
正在生成论文摘要