Iterative Algorithm for Optimal Super Resolution Sampling
springer
摘要
The resolution recovery in PET iterative methods such as OSEM, including all the physical effects involved in the system response matrix, is often limited by the reduced sampling in the projection space. In this work, we propose a method to further improve resolution recovery in the PET image reconstruction process by iteratively refining the measurements with data-driven increased sampling. In this method we first reconstruct the image by standard OSEM methods. After that, we define four subLORs around each initially measured LOR, and estimate the subLOR contribution spreading the original number of counts with maximum-likelihood based weights, computed with the relative value of the projections in each subLOR with respect to the four subLORs. Now we reconstruct the image with the standard OSEM algorithm using the subLORs set of data. We call this step a superiteration, which may be repeated 2–3 times until convergence is achieved. We have evaluated the improvements in image quality obtained using data acquired in the Argus PET/CT scanner. The method shows promising results increasing the recovery coefficients without noise raise measured in an Image Quality phantom, and also remarkable image quality improvements for live patients reconstructions.
更多查看译文
关键词
Imaging,Spectral Imaging,Perfusion Imaging,Susceptibility Weighted Imaging,Magnetic Resonance Imaging
AI 理解论文
溯源树
样例
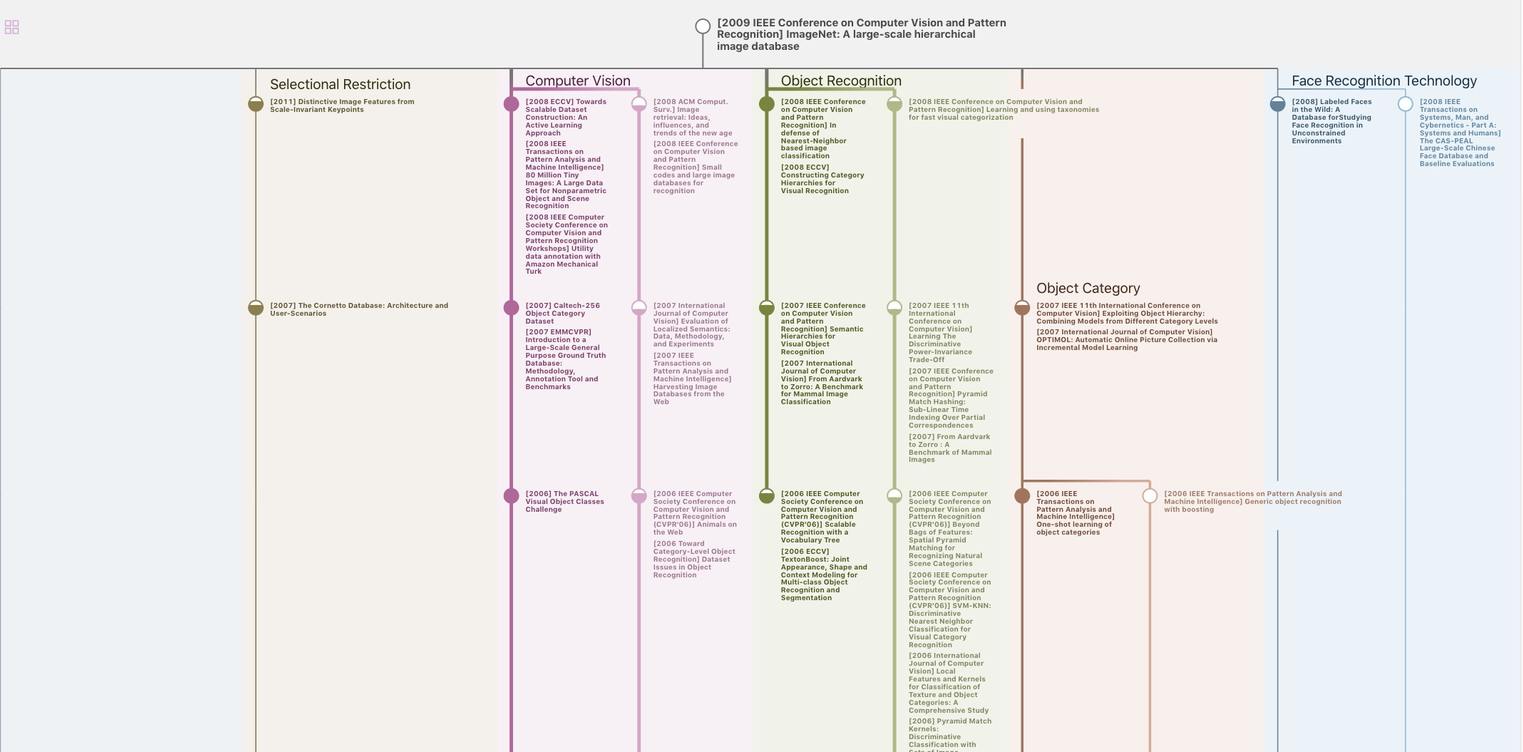
生成溯源树,研究论文发展脉络
Chat Paper
正在生成论文摘要