Integrating Digital Image Processing and Machine Learning for Estimating Rock Texture Characterization from Thin Section
Day 2 Tue, November 12, 2019(2019)
摘要
Abstract A methodical rock texture characterization of core samples and cuttings can provide powerful information that can be used reliably and cost-effectively to optimize fracture stimulation designs by placing fracture stages based on rock characteristics and drill bit selection. This paper presents a method to quantify rock texture using a developed integrated digital image processing to the neural network segmentaion for the analysis of the core-plug thin section. The standard routine uses the binarization process that converts the colored (RGB; Red Green Blue) core-plug thin section image into a set of binary images corresponding to different mineralogy content using the R, G, and B pixel values for thresholding. The idea is that the threshold values must accommodate all different colors that correlate to the rock texture and pore and help in creating a different mask (binary image) for each content type based on the threshold values used. The collection of the binary images for the different mineralogy will help in the rock texture interpretation. However, the neural network method used the data for multiple thin section images to learn based on the pattern of the color difference between pores and matrix. All results are validated with x-ray deflection (XRD). The proposed integrated system results show that, the thin section used in this work from an oilfield in the southern of Iraq is mainly quartz with minor Kaolinite and other components, where the mineral color code and weight percentages are recorded and validated with XRD. The comparison between the proposed image processing approach, neural network, and the XRD analysis show that good agreement with an acceptable percentage of error. These variations can help in understanding the rock behavior, optimize fracture stimulation designs and selecting the appropriate drill bits for drilling applications. The variations can also help in smart completion designs.
更多查看译文
AI 理解论文
溯源树
样例
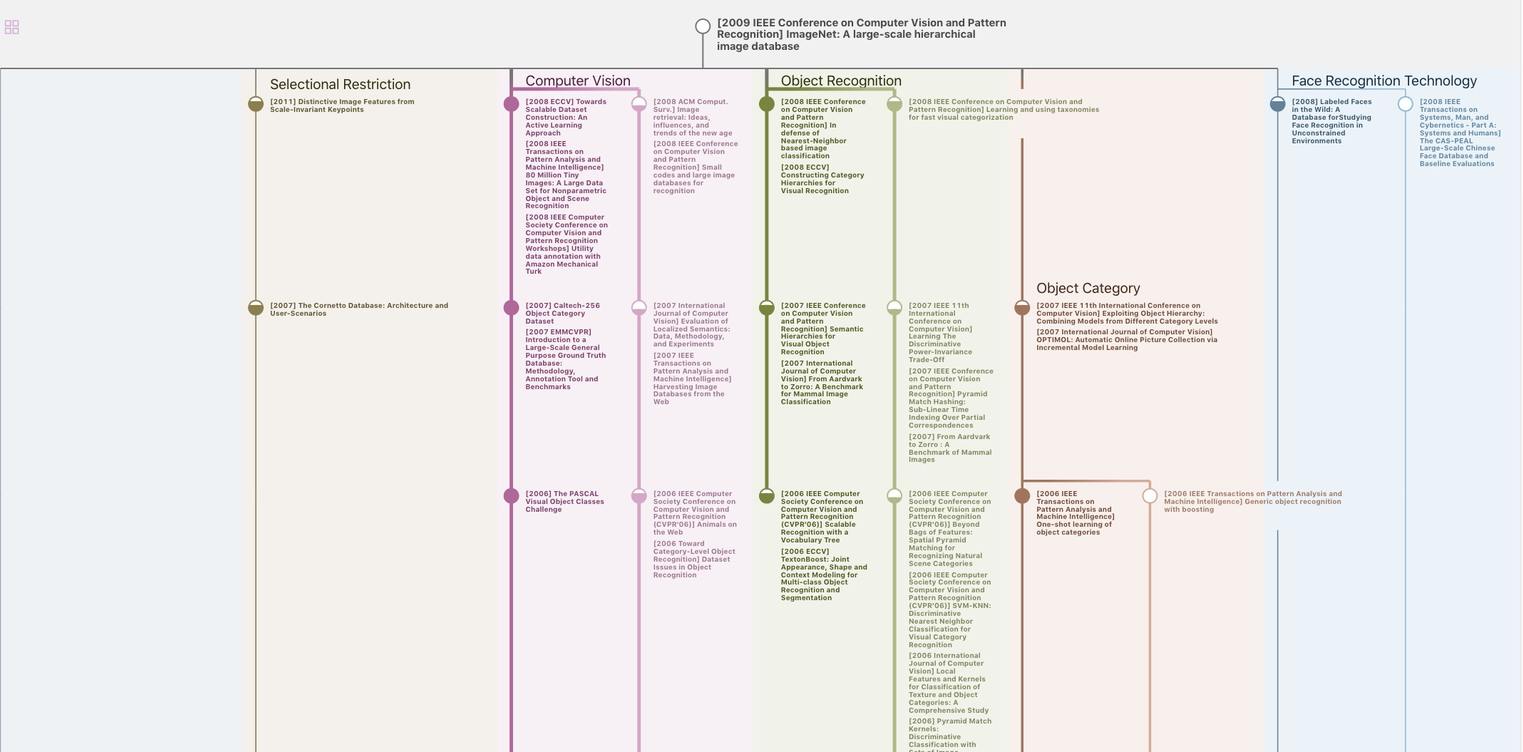
生成溯源树,研究论文发展脉络
Chat Paper
正在生成论文摘要